KSMDB: A classification method in imbalanced COVID dataset based on KmeansSMOTE and DeBERT
2022 IEEE International Conference on Bioinformatics and Biomedicine (BIBM)(2022)
摘要
2022 is already the third year of the COVID-19 outbreak, and public opinion information about the outbreak has always been at the forefront of hot searches. The imbalance problem prevalent in many reviews of COVID-19 causes classification models to favor most categories in training and prediction process, resulting in low accuracy of small sample classification data generated by imbalanced data sets. Therefore, it is suggested here that the text classification model is based on the combination of the KMeansSMOTE method combined with DeBERT. First of all, during data processing, the KmeansSMOTE algorithm is utilized to oversample the imbalance of the COVID dataset, which increases the classification accuracy of the model. Besides, we put a stacked denoising bidirectional transformer encoder (DeBERT) to use, a more abstract and richer hidden feature vector is extracted by adding an embedded layer after the input tag, and the noise data is reconstructed to solve the noise problem in the process of raw data existence and oversampling. Furthermore, on the basis of model training, overfitting can be alleviated by adopting an early stopping strategy. A world of experiments using the COVID dataset demonstrates the effectiveness of the proposed method for solving simple imbalance and noise problems. With an overall accuracy of 87%, which improves the classification effect of minority samples and provides a new feasible method for the war of epidemic prevention.
更多查看译文
关键词
KMeansSMOTE,imbalanced text,denoising COVID data,Oversampling,transformer
AI 理解论文
溯源树
样例
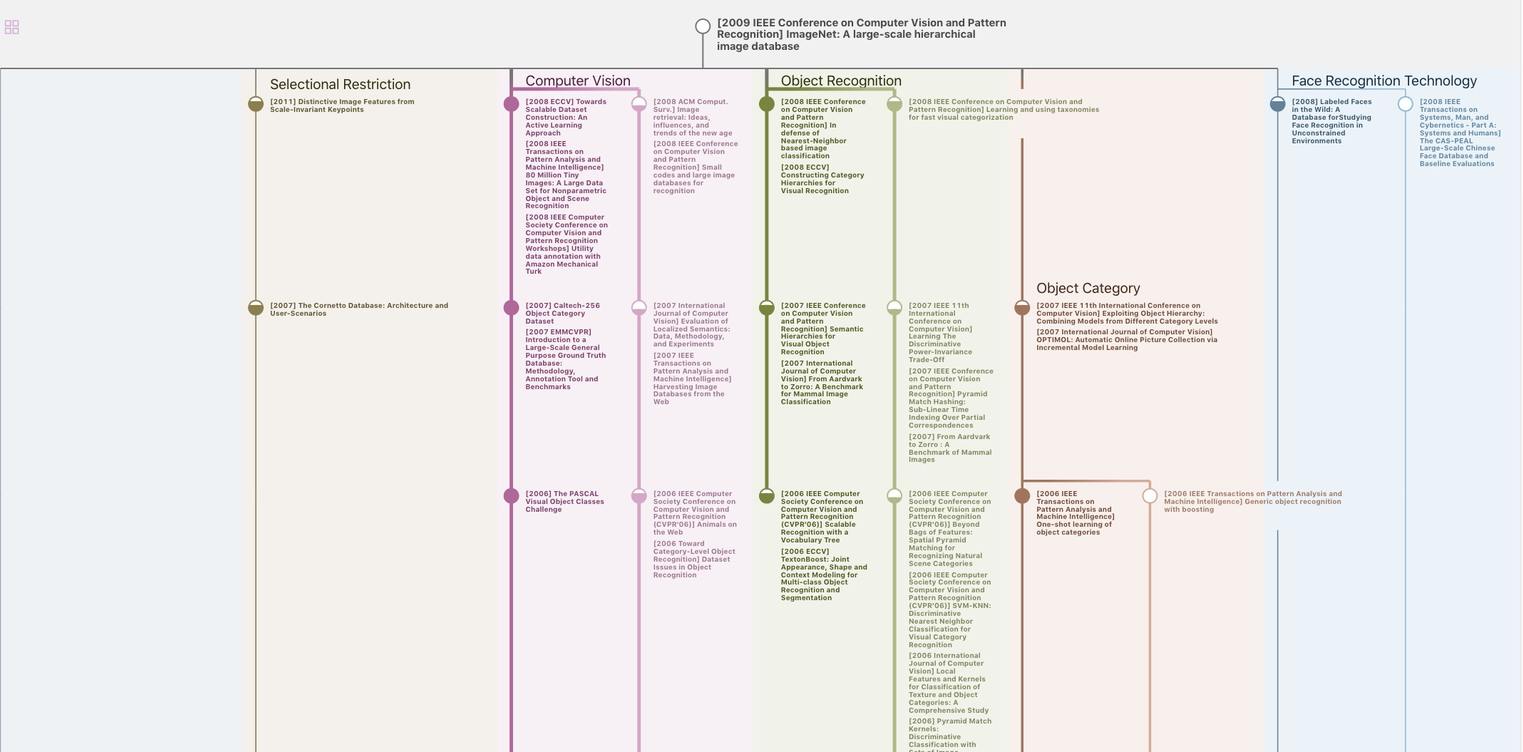
生成溯源树,研究论文发展脉络
Chat Paper
正在生成论文摘要