Dual regularized feature extraction and adaptation for cross-subject motor imagery EEG classification
2022 IEEE International Conference on Bioinformatics and Biomedicine (BIBM)(2022)
Abstract
Bioinformatics theoretical-based methods have attracted great attention in rehabilitation-assisted motor imagery (MI) brain-computer interface (BCI) using EEG, and gained promising results to deal with cross-subject MI-EEG classification. However, most of the existing methods are either suffered from outliers during feature learning across subjects or inefficient in computing discriminative features. Thus, they may not be able to obtain an optimal feature representation across subjects, resulting in classification performance deterioration. This paper proposes a dual regularization-based MI-EEG feature learning framework, in which feature weighted common spatial pattern, joint probability distribution alignment, and minimum Mahalanobis distance are taken into account, thus facilitating cross-subject classification. Specially, we adopt the multi-source to single target domain adaptation strategy, which is more suitable for real-world MI-BCI scenarios. Empirical studies on three benchmark MI-EEG datasets reveal the effectiveness and efficiency of the proposed method, which achieves a great performance improvement with less time consumption.
MoreTranslated text
Key words
motor imagery,EEG,cross-subject,domain adaptation,regularization
AI Read Science
Must-Reading Tree
Example
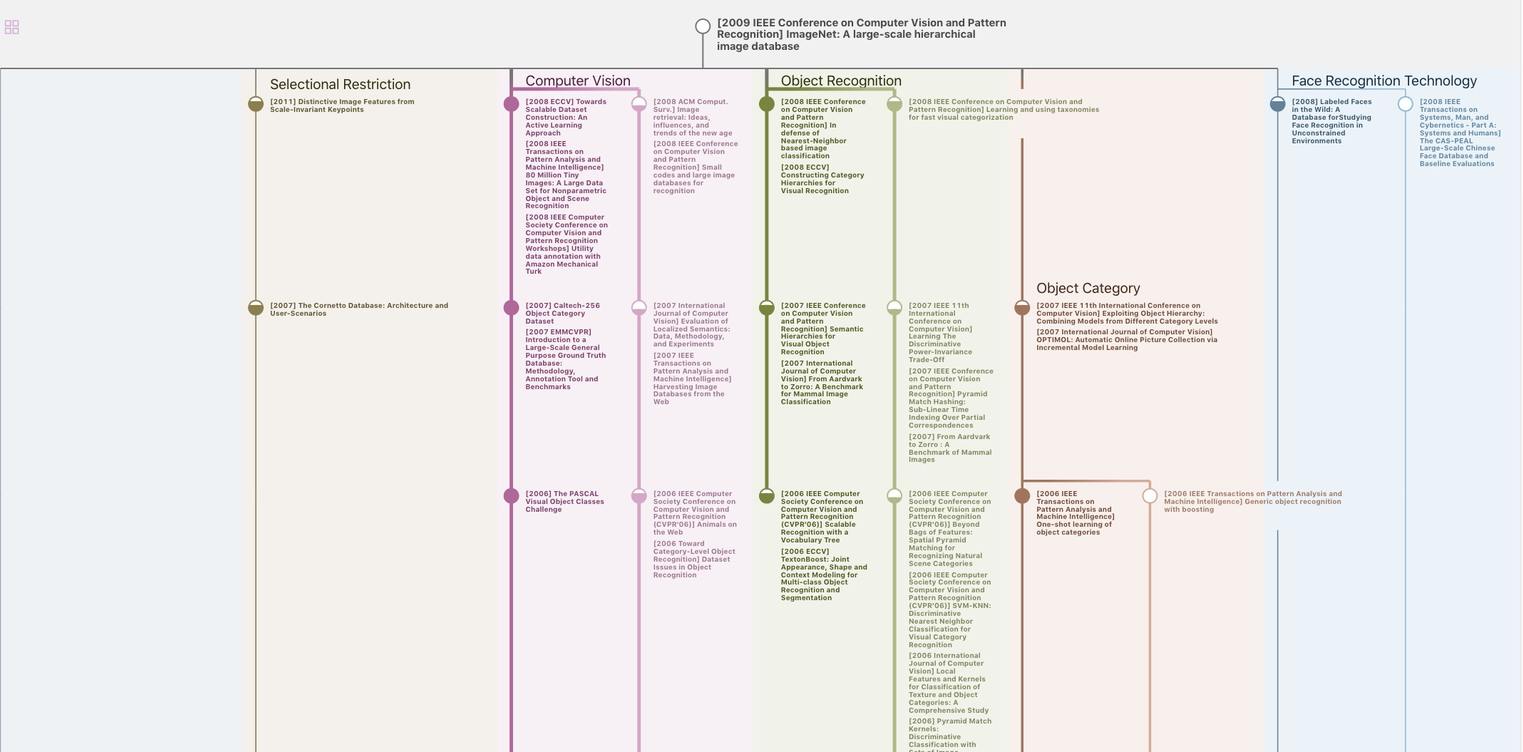
Generate MRT to find the research sequence of this paper
Chat Paper
Summary is being generated by the instructions you defined