A Reliability-constrained Association Rule Mining Method for Explaining Machine Learning Predictions on Continuity of Asthma Care
2022 IEEE International Conference on Bioinformatics and Biomedicine (BIBM)(2022)
摘要
Continuity of care has been shown to possess numerous health benefits for asthma. However, the use of methodology to predetermine the level of care continuity has been underinvestigated. We recently built a machine learning model to predict the continuity of care for diagnosed asthma patients at the University of Washington Medicine. As there is a consensus on the un-explainable featured nowadays by black-box machine learning, which impedes the clinical deployment of our model. To tackle this issue, we proposed a reliability-constrained association rule mining method, called RC-ARM, to automatically explain the predictions of any machine learning model. First, we introduced the belief function to build a reliability-constrained rule framework. Then, the two-step reliable association rule mining algorithms were developed to generate reliable explanation rules for the machine learning model’s predictions. At last, clinical intervention suggestions regarding each mined rule were embedded for further understanding. The results showed that the proposed method could explain all (110/110) predictions of our machine learning model for asthma patients with low levels of continuity of care. This semantic-fused method sheds light on black-box models and encourages clinical experts to embrace the benefits of machine learning without any prior concern about its lack of explainability.
更多查看译文
关键词
association rule mining,automated explanation,machine learning,asthma,continuity of care
AI 理解论文
溯源树
样例
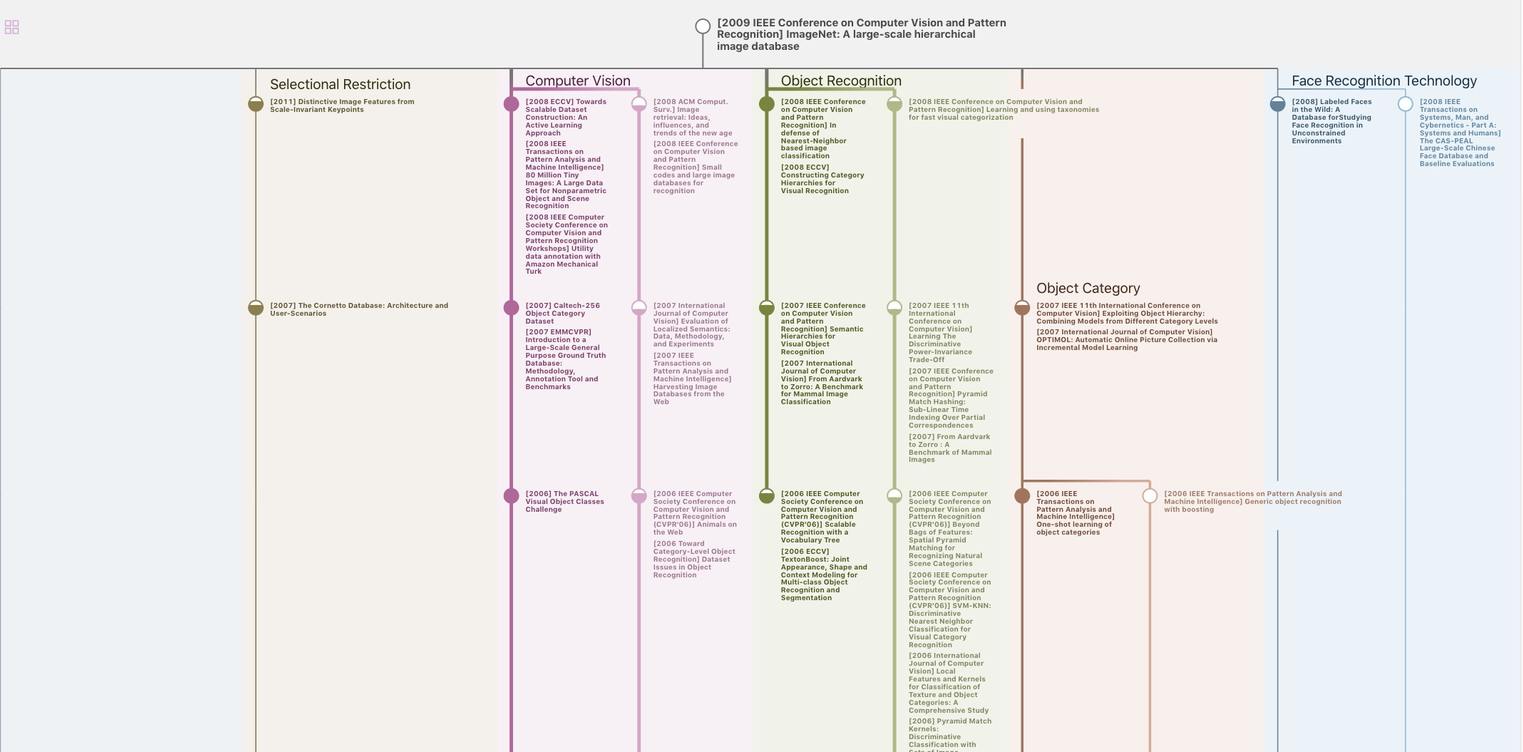
生成溯源树,研究论文发展脉络
Chat Paper
正在生成论文摘要