Comparison of anomaly detection algorithms for near-fall detection with video surveillance
2022 E-Health and Bioengineering Conference (EHB)(2022)
摘要
Falls are a major problem for seniors living in the community. To prevent their negative consequences, we propose a video surveillance system to detect early signs of mobility decline based on the frequency of near-fall occurrences (e.g., stumble, slip, misstep, loss of balance) for more efficient intervention before a fall occurs. Velocity features were extracted from a simplified skeleton fitted to the body based on the keypoint RCNN (Region-based Convolutional Neural Network) algorithm. Then, three anomaly detection algorithms (One-Class SVM, Gaussian Mixture Model (GMM) and Isolation Forest (IF)) were tested to identify abnormal events in a dataset of 55 videos (> 16000 frames) of simulated normal and abnormal activities in a realistic apartment-laboratory. Our experimental results showed that all three algorithms could successfully distinguish anomalies (near-falls) with an EER (Equal Error Rate) of 10% (AUC = 87%) for the One-Class SVM, EER = 20% (AUC = 85%) for the GMM, and EER = 10% (AUC = 92%) for the Isolation Forest. This study demonstrates the potential of a smart video surveillance system to identify near-falls associated with mobility decline for the benefit of older adults living alone at home.
更多查看译文
关键词
Video surveillance,Near-falls,One-class SVM,Gaussian Mixture Model,Isolation forest
AI 理解论文
溯源树
样例
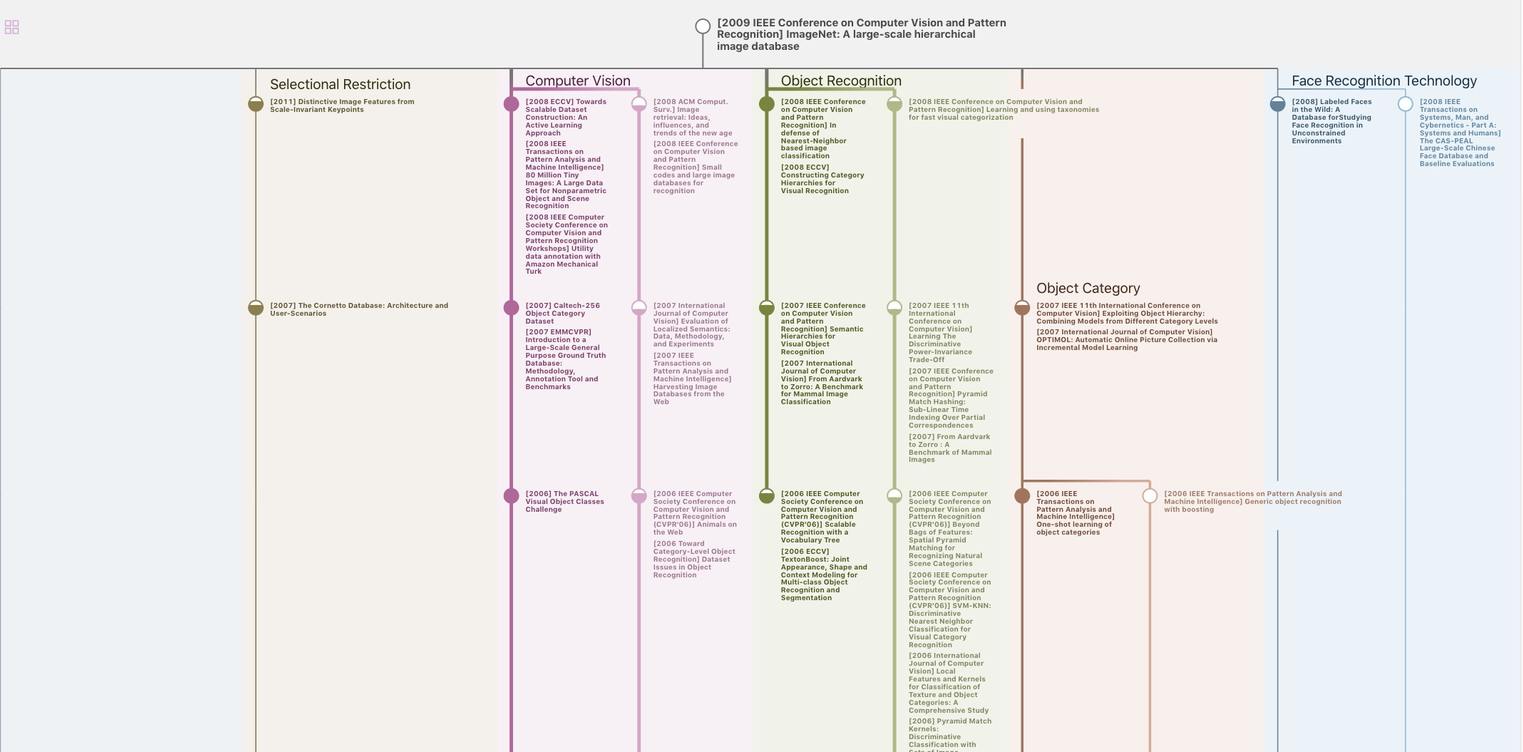
生成溯源树,研究论文发展脉络
Chat Paper
正在生成论文摘要