Forward Kinematics Solution for Stewart and TBBP: A Neural Network Approach Based on LSTM
2022 5th International Conference on Robotics, Control and Automation Engineering (RCAE)(2022)
摘要
Aiming the problems of the traditional numerical method for solving the pose forward kinematics of parallel mechanism, which relies heavily on the initial value of iteration, has low accuracy and offers poor real-time performance, this article thus proposed a method combining LSTM (Long-Short-Term Memory) prediction model with inverse kinematics solution of parallel mechanism. This method takes the typical Stewart platform (SP) and the test bench for bogie parameters (TBBP) as sample robots. First, the constraint conditions of the two mechanisms were considered in order to obtain the workspace for each; the pose inverse solution Simulink models for both mechanisms were then generated. Second, given the motion posture in the workspace, the telescopic amount of the driving rod can be obtained. Then the data corresponding to the time series of the 6-DOF pose and the telescopic amount was taken as sample training data for LSTM in the next step. Finally, RMSE (Root Mean Square Error) and MAPE (Mean Absolute Percentage Error) were applied as optimisation indexes to determine the optimal structural parameter combinations of LSTM networks to analyse the prediction effects for both the SP and TBBP mechanisms under a circular trajectory, a spiral trajectory, and 0∽5 Hz linear sweep vibration trajectory. The results showed that the order of magnitude of RMSE of the prediction models for both mechanisms was 10–
4
for both the circular and spiral trajectory, especially the RMSE for TBBP was 10–
5
for the spiral trajectory, a MAPE of just 0.0013%. Comparing the above-fixed frequency trajectory, the RMSE and MAPE under the 0∽5Hz linear variable frequency trajectory are 0.0931 and 4.45% respectively; The prediction results for both structures meet the stated accuracy requirements and verify that the proposed LSTM prediction method offers both strong universality and good rationality.
更多查看译文
关键词
parallel mechanism,forward kinematics,Stewart,TBBP,LSTM neural network,time series forecasting
AI 理解论文
溯源树
样例
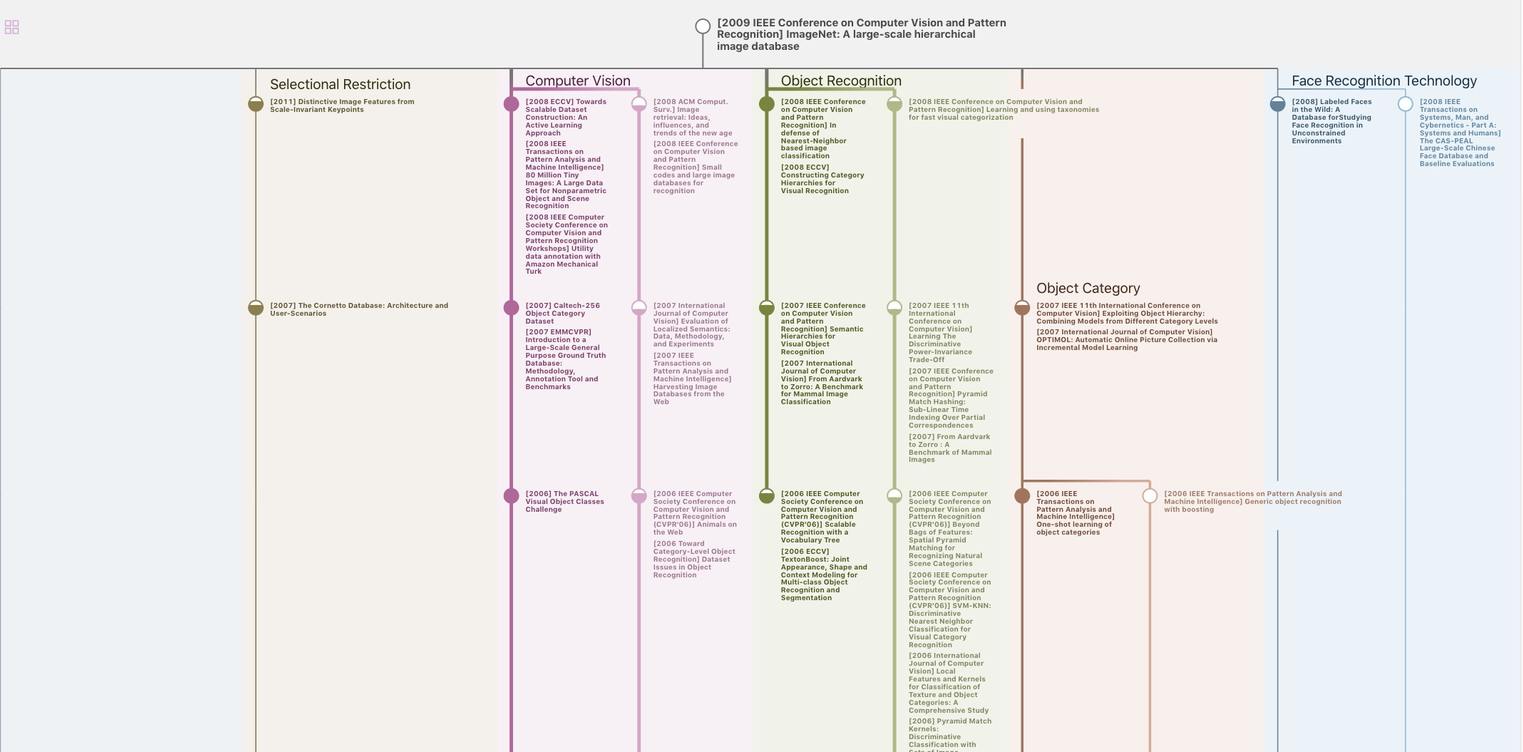
生成溯源树,研究论文发展脉络
Chat Paper
正在生成论文摘要