Multi-Class Support Vector Machine for Classifying Defect Types of High Voltage Stator Windings
2022 9th International Conference on Condition Monitoring and Diagnosis (CMD)(2022)
摘要
For high-voltage rotating machines used in power plants, partial discharge (PD) monitoring is performed during maintenance and inspection periods. However, nowadays, an online PD diagnostic system is generally employed as the tool to provide early warning of an incipient fault in high-voltage stator windings. In this study, an algorithm is described that can classify the defect types of stator windings. The classification process is based on analysis of the phase-resolved PD spectrum obtained by a capacitive slot coupler (CSC) sensor inserted between the stator core and bottom of the stator winding. The first technique to utilize the CSC sensor is the application of sweep frequency response technique (SFRA) to monitor the degradation level of interturn insulation. The second is the adoption of machine learning technique to classify the type of insulation defect in stator windings. After reviewing several artificial intelligence techniques, a multiclass SVM was selected as the main classification algorithm, and six defect types of stator winding were trained as multiple classes and then tested. Based on the Python Scikit-learn library, both polynomial-type and radial basis function (RBF) type kernels were utilized for the SVM analysis by changing the dimensional level to find the optimal hyperplane. In the RBF kernel method, the optimal classification accuracy was derived by varying the gamma and cost parameters. Finally, the classification accuracy was 90% for the polynomial kernel and 94% for the RBF kernel. This classification algorithm will be adopted in the novel on-line diagnosis unit as an initial step toward the digital power plant.
更多查看译文
关键词
Online PD,Digital Power Plant,PD Diagnostic,SVM
AI 理解论文
溯源树
样例
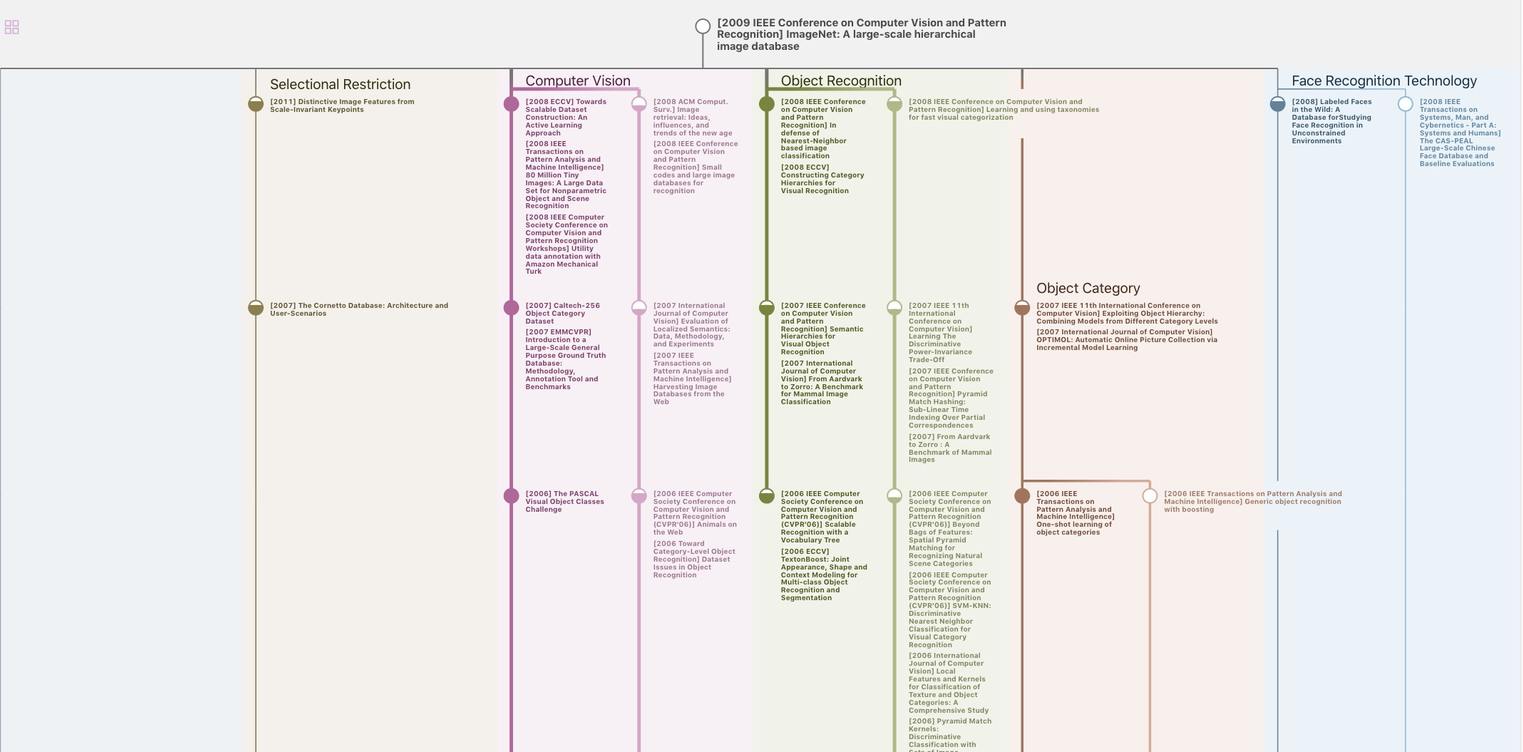
生成溯源树,研究论文发展脉络
Chat Paper
正在生成论文摘要