Prediction of ultimate condition of FRP-confined recycled aggregate concrete using a hybrid boosting model enriched with tabular generative adversarial networks
Thin-Walled Structures(2023)
摘要
Despite its multiple benefits, recycled aggregate concrete (RAC) usually exhibits inferior properties compared with natural aggregate concrete, which has been deemed as a hurdle to its widespread use. One way to overcome this roadblock is to apply FRP jacketing. However, it appears not easy to quantify the axial performance of FRP-confined recycled aggregate concrete columns (FRACC), due largely to the complex load-resisting mechanisms involved. Also, the weakness of RAC itself further compound the problem. This paper aspires to deliver an alternative means to address this difficulty. A powerful boosting approach, XGBoost, was developed to fulfill the goal, where its hyperparameters was fine-tuned by a beetle antennae search metaheuristic algorithm. Meanwhile, a synthetic data generator, tabular generative adversarial networks, was introduced to supplement the limited training data. The model developed outperformed existing empirical equations and several baseline machine learning models. Teng et al.’s FRP-confined concrete model was also improved for better tracing the axial stress–strain behavior. Besides, interpreting the model allows better understanding of the underlying mechanisms such as the minimum reinforcement ratio of FRP required to mitigate the negative effects of RAC. Finally, two data-driven, explicit design equations are given for practical design of FRACC.
更多查看译文
关键词
FRP confined concrete,Recycled aggregate concrete,Machine learning,XGBoost,Beetle antennae search algorithm,Axial behavior
AI 理解论文
溯源树
样例
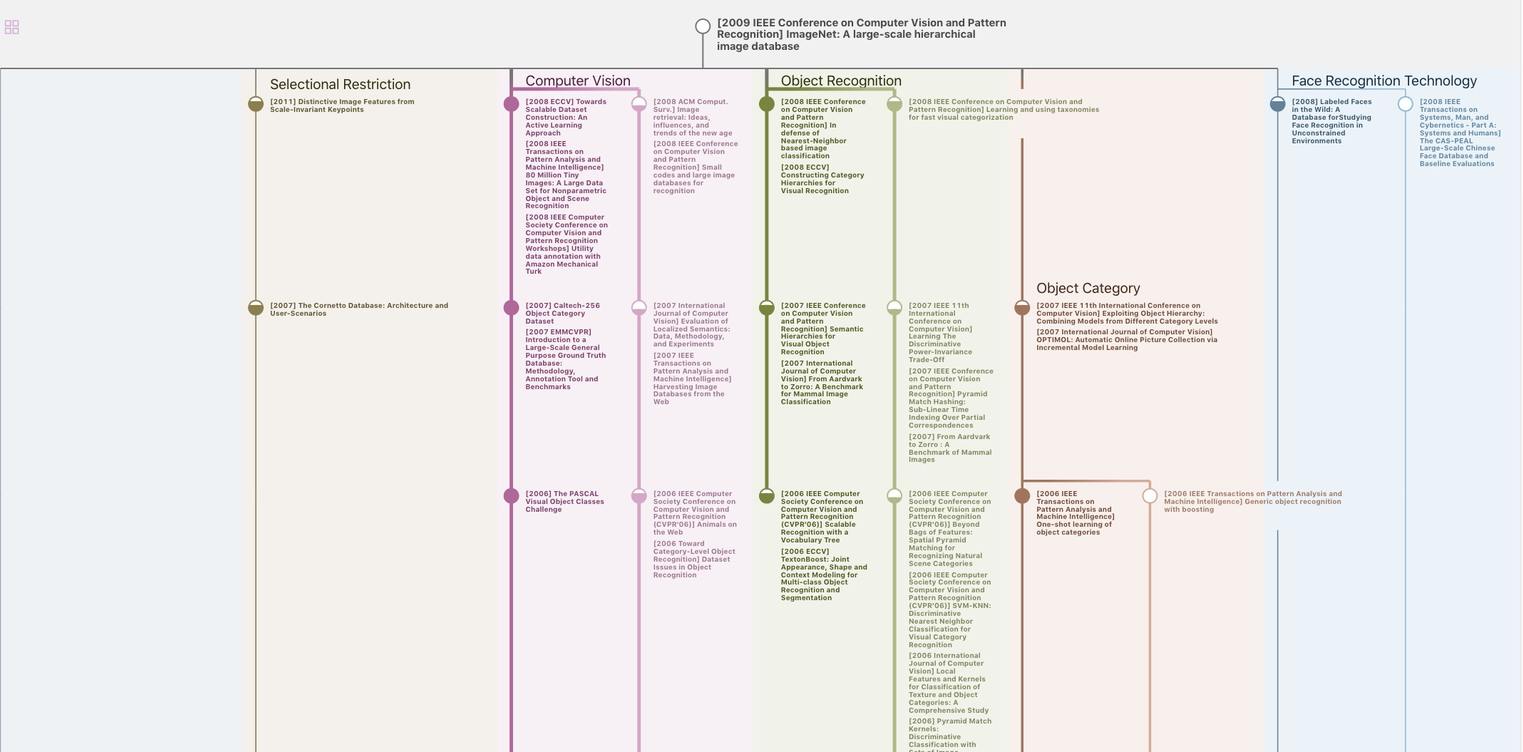
生成溯源树,研究论文发展脉络
Chat Paper
正在生成论文摘要