A Multi-Factor Driven Spatiotemporal Wind Power Prediction Model Based on Ensemble Deep Graph Attention Reinforcement Learning Networks
Energy(2023)
摘要
Spatiotemporal wind power prediction technology could provide technical support for wind farm energy regulation and dynamic planning. In the paper, a novel ensemble deep graph attention reinforcement learning network is designed to build a multi-factor driven spatiotemporal wind power prediction model. Firstly, the graph attention network (GAT) algorithm is applied to aggregate and extract the spatiotemporal features of the raw wind power data. Then, the extracted features were put into the gated recursion unit (GRU) and temporal convolutional network (TCN) methods to form the wind power forecasting model and the results are obtained respectively. Finally, the deep deterministic policy gradient (DDPG) algorithm integrates the forecasting results of TCN and GRU by dynamically optimizing the weight coefficients and the results are thus obtained. Based on several comparative experiments and case studies, several important conclusions are drawn: (1) GAT can effectively extract the depth feature information of spatial and temporal wind power data and optimize the results of the predictor. (2) DDPG can increase the robustness and generalization of the prediction framework by integrating GAT-TCN and GAT-GRU. (3) The proposed ensemble model can obtain accurate wind power prediction results and is better than twenty-six contrast algorithms proposed by other researchers.
更多查看译文
关键词
Spatiotemporal wind power forecasting,Graph attention network,GRU,TCN,DDPG
AI 理解论文
溯源树
样例
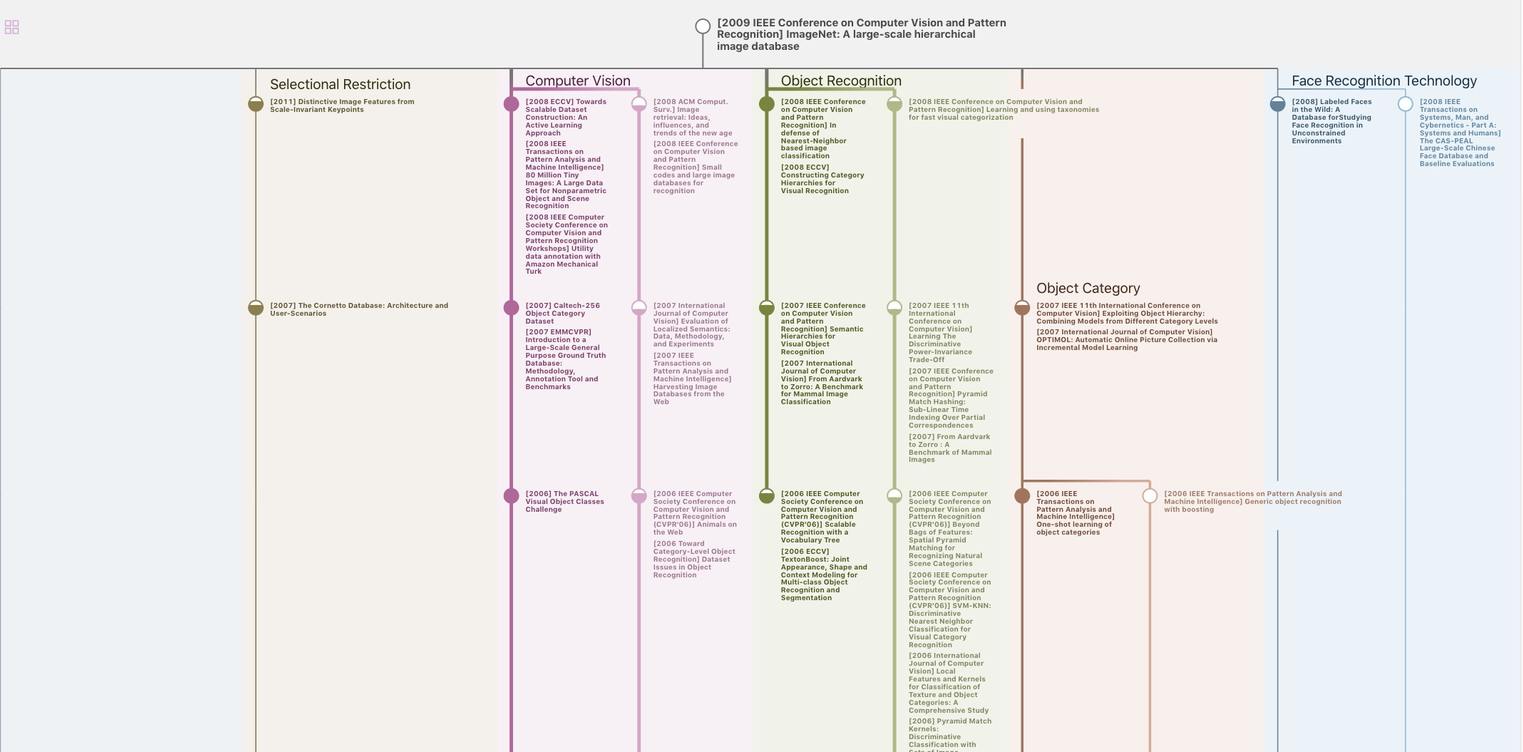
生成溯源树,研究论文发展脉络
Chat Paper
正在生成论文摘要