Deep Learning for Single-Channel EEG-Based Driver Drowsiness: Comparative Study
2022 International Arab Conference on Information Technology (ACIT)(2022)
摘要
To prevent car accidents caused by drowsiness, a transitional state between alertness and sleepiness, numerous authors have conducted studies employing various monitoring approaches and detecting techniques. However, the Electroencephalogram (EEG) was the most used monitoring approach due to its advantages compared to other techniques. Also, Deep Learning (DL) techniques have been widely used in this context and have given prominent results. In this paper, we have established a comparative study between four deep learning methods. The aim of this comparison is to determine the optimal DL model for detecting EEG-Based Drowsiness using a small balanced dataset. We evaluated the performance of the models using the hold-out method and the Leave-One-Subject-Out Cross-Validation (LOSO CV) techniques. With an accuracy of 77% with the Hold-Out method and 70.31% with the LOSO CV method, the GRU model outperforms other deep learning and machine learning models, followed by the BI-LSTM with 74.88% and 68.07, respectively.
更多查看译文
关键词
Driver Drowsiness,Electroencephalogram (EEG),Deep Learning,CNN,LSTM,GRU,Bi-LSTM,Cross-Subject
AI 理解论文
溯源树
样例
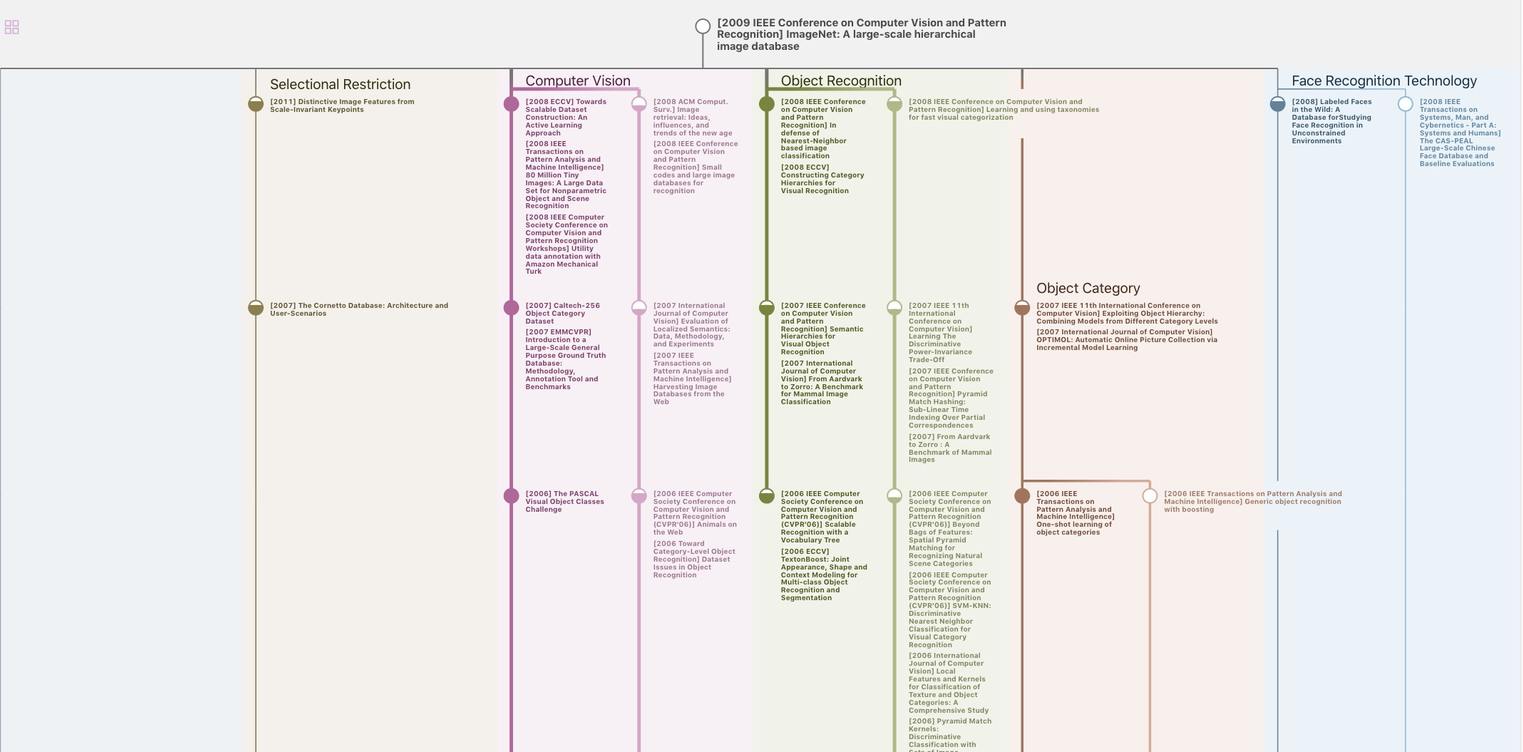
生成溯源树,研究论文发展脉络
Chat Paper
正在生成论文摘要