Using machine learning techniques to predict academic success in an introductory programming course
2022 41st International Conference of the Chilean Computer Science Society (SCCC)(2022)
摘要
The great advances in processes and services automation has turned programming skills into a key element in the formation of new professionals, specially in scientific disciplines. However, students often struggle to develop such skills. This article aims to identify which variables show the highest correlation with success in learning to program. For this purpose, the research team gathered data of various cohorts of students coursing an initial programming course, common to all the engineering programmes offered by the Facultad de Ingeniería of the Universidad de Santiago de Chile. The data set contained information of 3,130 students who took the course between 2015 and 2019. The data was then studied in order to predict success or failure in the theory part of the course, which also has a laboratory. Several classifying methods were considered for this purpose, namely: Support Vector Machines, Multivariate Logistic Regression, CART Trees, Extreme Learning Machines, Random Forests and Extreme Gradient Boosting. Best results are achieved using radial kernel Support Vector Machines, with an accuracy of 68.6%, to predict if a given student passes or fails the theory part of the course.
更多查看译文
关键词
machine learning,CS01,programming in engineering
AI 理解论文
溯源树
样例
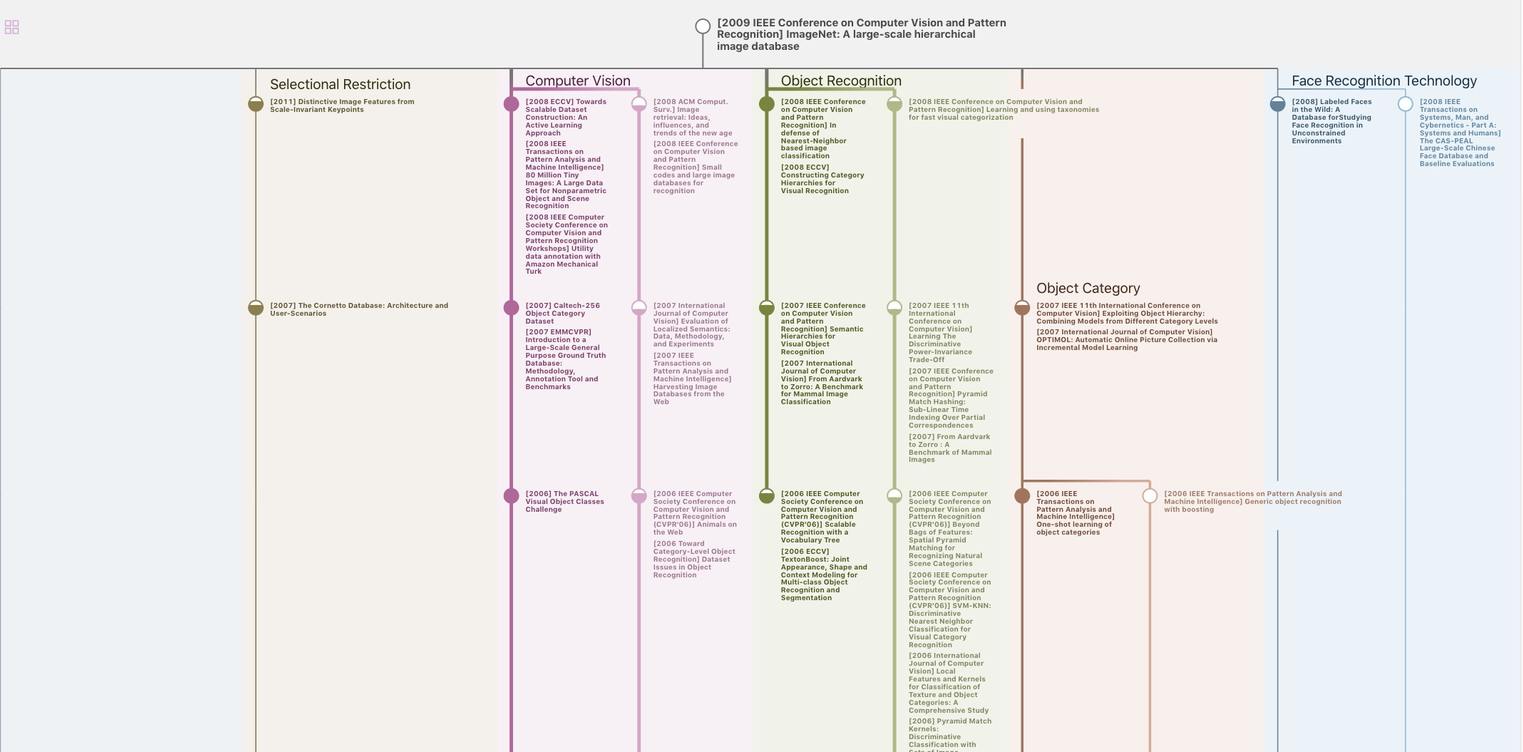
生成溯源树,研究论文发展脉络
Chat Paper
正在生成论文摘要