Towards a Real-Time Smart Prognostics and Health Management (PHM) of Safety Critical Embedded Systems
2022 25th Euromicro Conference on Digital System Design (DSD)(2022)
摘要
Machine learning techniques for the prognostics and health management of safety critical embedded systems is an area that has raised an increased interest recently. This paper investigates an implementable machine learning pipeline to address PHM requirements. Different types of machine learning techniques are evaluated on a real case study considering accuracy and real-time effectiveness. The referred system exhibited abnormal behaviour with multiple faults during an observed period, which led it to a low system availability and required maintenance, disturbing the normal operation. This paper presents a system approach to the fault finding, aiming its use in real-time applications. We started with a thorough review of the available features, followed by a consistent hyperparameters optimization across all different techniques, ensuring a comparable baseline. Finally, the results were compared according to a defined evaluation and performance criteria, using a) metrics such as mean square error and R-squared to determine how well the model fitted the dataset, and b) execution times for their fit and predict methods for different training and test sizes, to anticipate their performance on real-time applications. All models presented a very good result to predict the output state of the system, but the stacked models outperformed the remaining ones. The proposed framework has been tested and validated on a real application case.
更多查看译文
关键词
PHM,Machine Learning,Safety-Critical Systems,Embedded Systems,Condition Monitoring,Remaining Useful Life,Fault Prediction,LSTM,Neural Networks,Support Vector Regression,Decision Tree,Logistic Regression
AI 理解论文
溯源树
样例
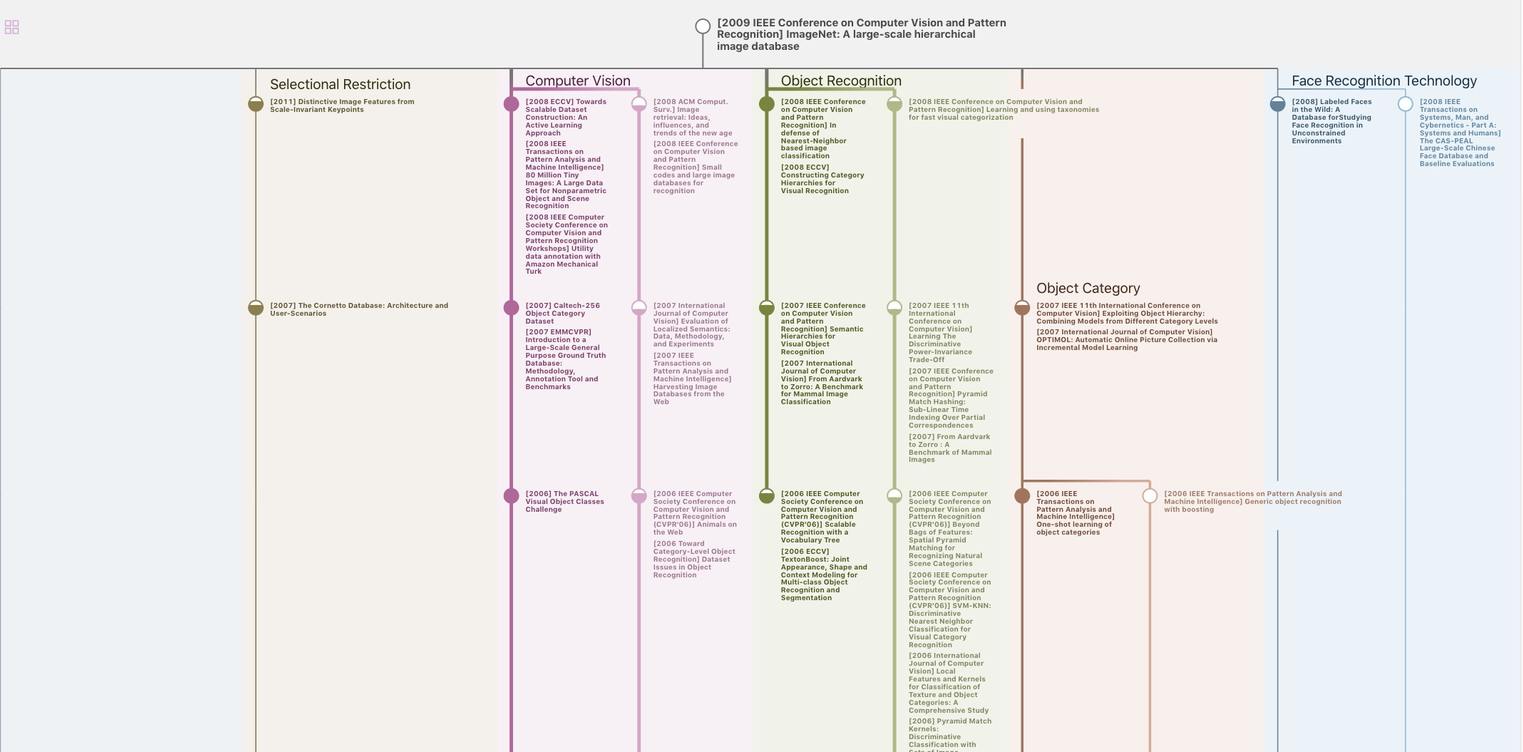
生成溯源树,研究论文发展脉络
Chat Paper
正在生成论文摘要