Human Skeleton Feature Optimizer and Adaptive Structure Enhancement Graph Convolution Network for Action Recognition
IEEE Transactions on Circuits and Systems for Video Technology(2023)
摘要
Human action recognition based on the graph convolution network (GCN) is a hot topic in computer vision. Existing GCN-based methods fail to capture internal implicit information when extracting action features, thereby leading to over-smoothing in the training stage. These issues result in poor performance and inaccurate extraction of action features. To address these problems, a new GCN is constructed. In this paper, a human skeleton feature optimizer (SFO) and adaptive structure enhancement graph convolution network (ASE-GCN) for action recognition are proposed in an end-to-end manner. To obtain discriminative features, the SFO is proposed to construct a new skeleton representation for action recognition through the connection criterion, which extracts the internal implicit information of action. The action feature of the joint coordinates is extracted by graph structure mask (GSM), directed graph mapping (DGM), and adaptive pooling operation (APO) in the proposed ASE-GCN network. The GSM acts as the regularizer of skeleton structure information to strengthen the representation of the graph structure. The DGM correlates the directed graph with human motion information through kinematic principle, and the APO strengthens the global high-frequency features to alleviate over-smoothing. The proposed method achieves comparable or superior results over state-of-the-art methods when used in experiments on two large public-scale datasets, NTU-RGB+D and Kinetics.
更多查看译文
关键词
Action recognition,graph convolution network,skeleton feature optimizer,graph structure mask,directed graph mapping,adaptive pooling operation
AI 理解论文
溯源树
样例
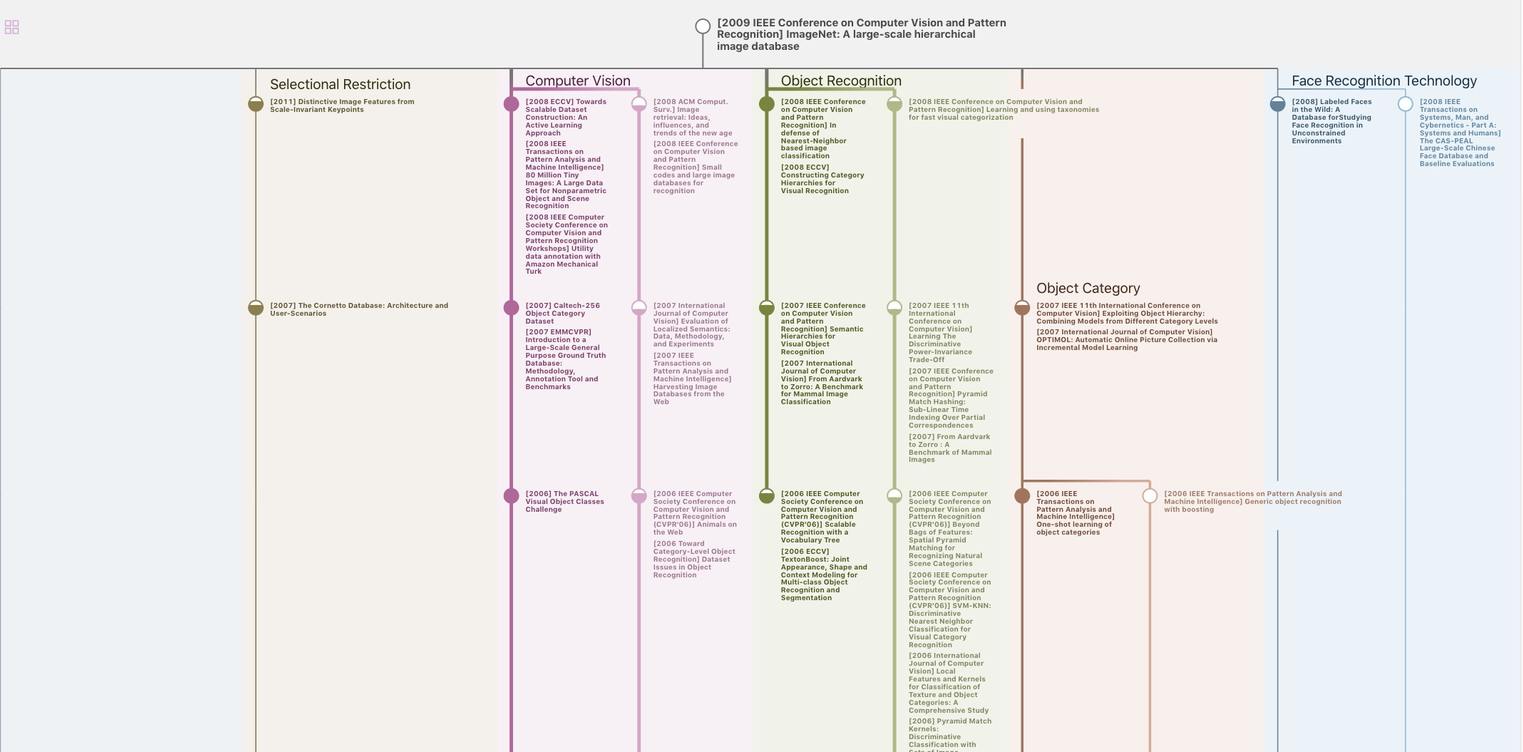
生成溯源树,研究论文发展脉络
Chat Paper
正在生成论文摘要