A Bayesian Meta-Learning-Based Method for Few-Shot Hyperspectral Image Classification
IEEE Transactions on Geoscience and Remote Sensing(2023)
摘要
Few-shot learning provides a new way to solve the problem of insufficient training samples in hyperspectral classification. It can implement reliable classification under several training samples by learning meta-knowledge from similar tasks. However, most existing works perform frequency statistics, which may suffer from the prevalent uncertainty in point estimates (PEs) with limited training samples. To overcome this problem, we reconsider the hyperspectral image few-shot classification (HSI-FSC) task as a hierarchical probabilistic inference from a Bayesian view and provide a careful process of meta-learning probabilistic inference. We introduce a prototype vector for each class as latent variables and adopt distribution estimates (DEs) for them to obtain their posterior distribution. The posterior of the prototype vectors is maximized by updating the parameters in the model via the prior distribution of HSI and labeled samples. The features of the query samples are matched with prototype vectors drawn from the posterior; thus, a posterior predictive distribution over the labels of query samples can be inferred via an amortized Bayesian variational inference approach. Experimental results on four datasets demonstrate the effectiveness of our method. Especially given only three to five labeled samples, the method achieves noticeable upgrades of overall accuracy (OA) against competitive methods.
更多查看译文
关键词
Bayesian meta-learning,few-shot learning,hyperspectral image classification (HSIC),prototype vector
AI 理解论文
溯源树
样例
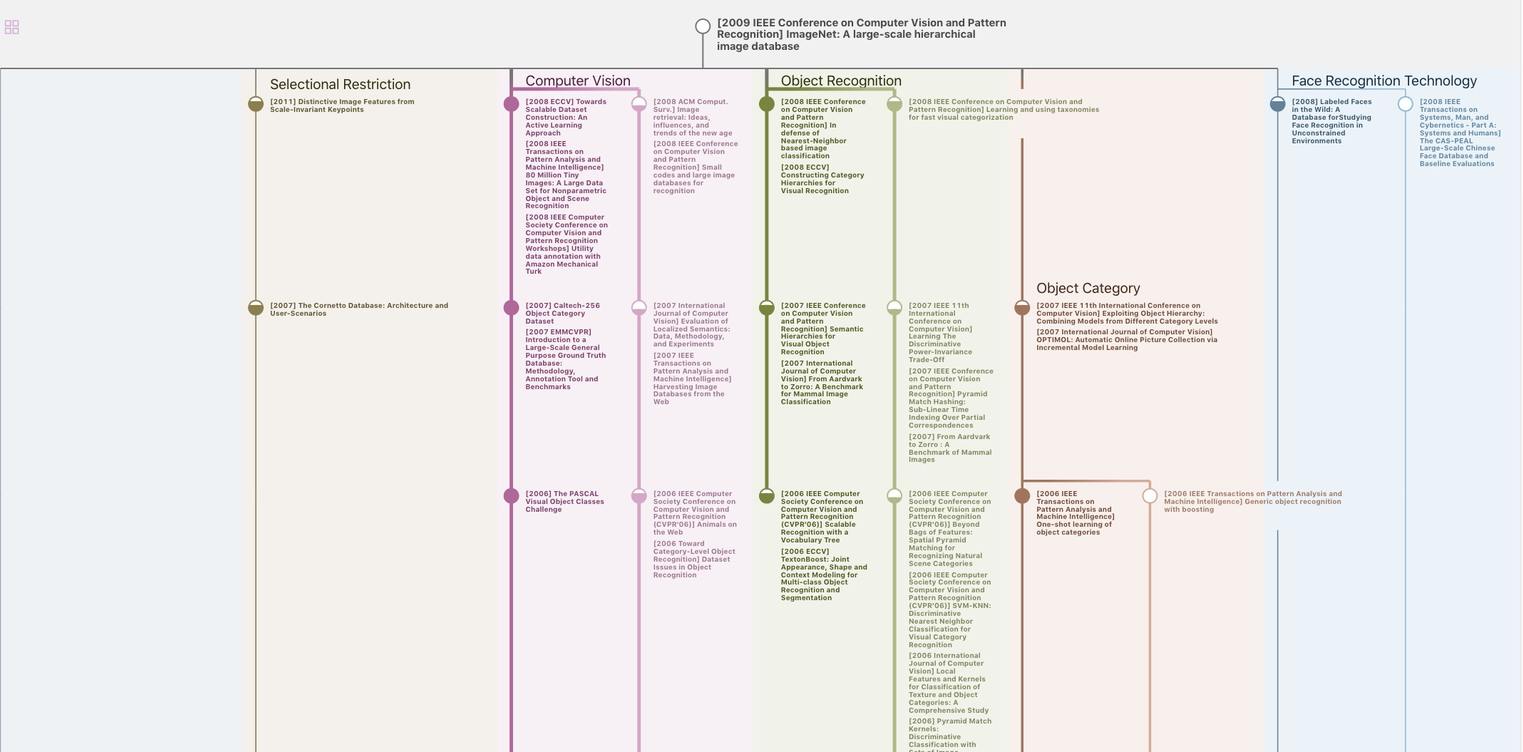
生成溯源树,研究论文发展脉络
Chat Paper
正在生成论文摘要