Predicting the Force Map of an ERT-Based Tactile Sensor Using Simulation and Deep Networks
IEEE Transactions on Automation Science and Engineering(2023)
摘要
Electrical resistance tomography (ERT) can be used to create large-scale soft tactile sensors that are flexible and robust. Good performance requires a fast and accurate mapping from the sensor’s sequential voltage measurements to the distribution of force across its surface. However, particularly with multiple contacts, this task is challenging for both previously developed approaches: physics-based modeling and end-to-end data-driven learning. Some promising results were recently achieved using sim-to-real transfer learning, but estimating multiple contact locations and accurate contact forces remains difficult because simulations tend to be less accurate with a high number of contact locations and/or high force. This paper introduces a modular hybrid method that combines simulation data synthesized from an electromechanical finite element model with real measurements collected from a new ERT-based tactile sensor. We use about 290 000 simulated and 90000 real measurements to train two deep neural networks: the first (Transfer-Net) captures the inevitable gap between simulation and reality, and the second (Recon-Net) reconstructs contact forces from voltage measurements. The number of contacts, contact locations, force magnitudes, and contact diameters are evaluated for a manually collected multi-contact dataset of 150 measurements. Our modular pipeline’s results outperform predictions by both a physics-based model and end-to-end learning. Note to Practitioners–ERT-based tactile sensors use high-speed voltage measurements from electrodes distributed over a piezoresistive area to output a force map that shows where contact is occurring, and how strong each contact is. Such sensors hold promise for giving robots and other devices a sense of touch over large surfaces with low hardware complexity. However, the software problem of converting voltages to an accurate force map has not previously been solved well, requiring either extensive model calibration or extensive contact data collection. This paper suggests a hybrid approach where a straightforward physics model simulates multi-contact experiments that are too costly to acquire in reality and a practical automatic indentation setup acquires real but geometrically limited multi-contact data. Although the number of real measurements required to learn the discrepancy between the sensor and the model is still large due to the inherent inverse nature of ERT-based tactile sensors, our combination of simulation and deep networks achieves better performance than either physical modeling or learning alone. This approach can advance practical large-area tactile sensing for industrial automation systems where multiple contacts occur, such as in manufacturing and assistive robotics. It could also likely be adapted to other nonlinear inverse problems.
更多查看译文
关键词
Multi-point tactile sensing,electrical impedance tomography,electromechanical modeling,sim-to-real transfer,machine learning
AI 理解论文
溯源树
样例
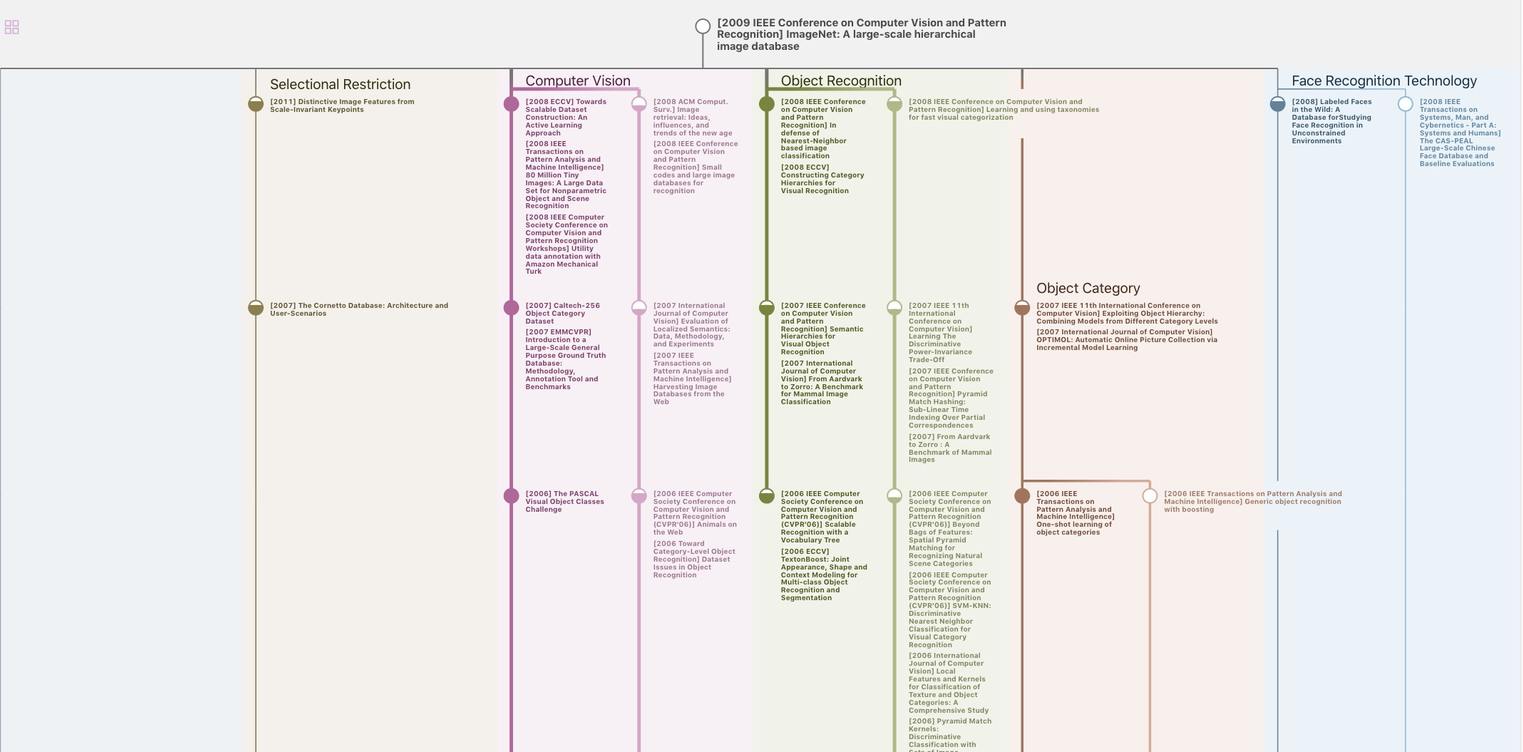
生成溯源树,研究论文发展脉络
Chat Paper
正在生成论文摘要