Traffic classification using distributions of latent space in software-defined networks: An experimental evaluation
Engineering Applications of Artificial Intelligence(2023)
摘要
With the emergence of new Internet services and the drastic increase in Internet traffic, traffic classification has become increasingly important to effectively satisfy the quality of service to users. The traffic classification system should be resilient and operate smoothly regardless of network conditions or performance and should be capable of handling various classes of Internet services. This paper proposes a traffic classification method in a software-defined network environment that employs a variational autoencoder (VAE) to accomplish this. The proposed method trains the VAE using six statistical features and extracts the distributions of latent features for the flows in each service class. Furthermore, it classifies the query traffic by comparing the distributions of latent features for the query traffic with the learned distributions of the service classes. For the experiment, the statistical features of network flows were collected from real-world domestic and overseas Internet services for training and testing. According to the experimental results, the proposed method has an average accuracy of 89%. This accuracy was 52%, 47%, 39%, 59%, and 26% higher than conventional statistics-based classification methods, MLP, AE+MLP, VAE+MLP, and SVM, respectively. This result clearly suggests that probability distributions of latent features, rather than specific values for latent features, can be used as more stable features.
更多查看译文
关键词
Flow classification,Jensen–Shannon divergence,Latent features,Software-defined network,Variational autoencoder
AI 理解论文
溯源树
样例
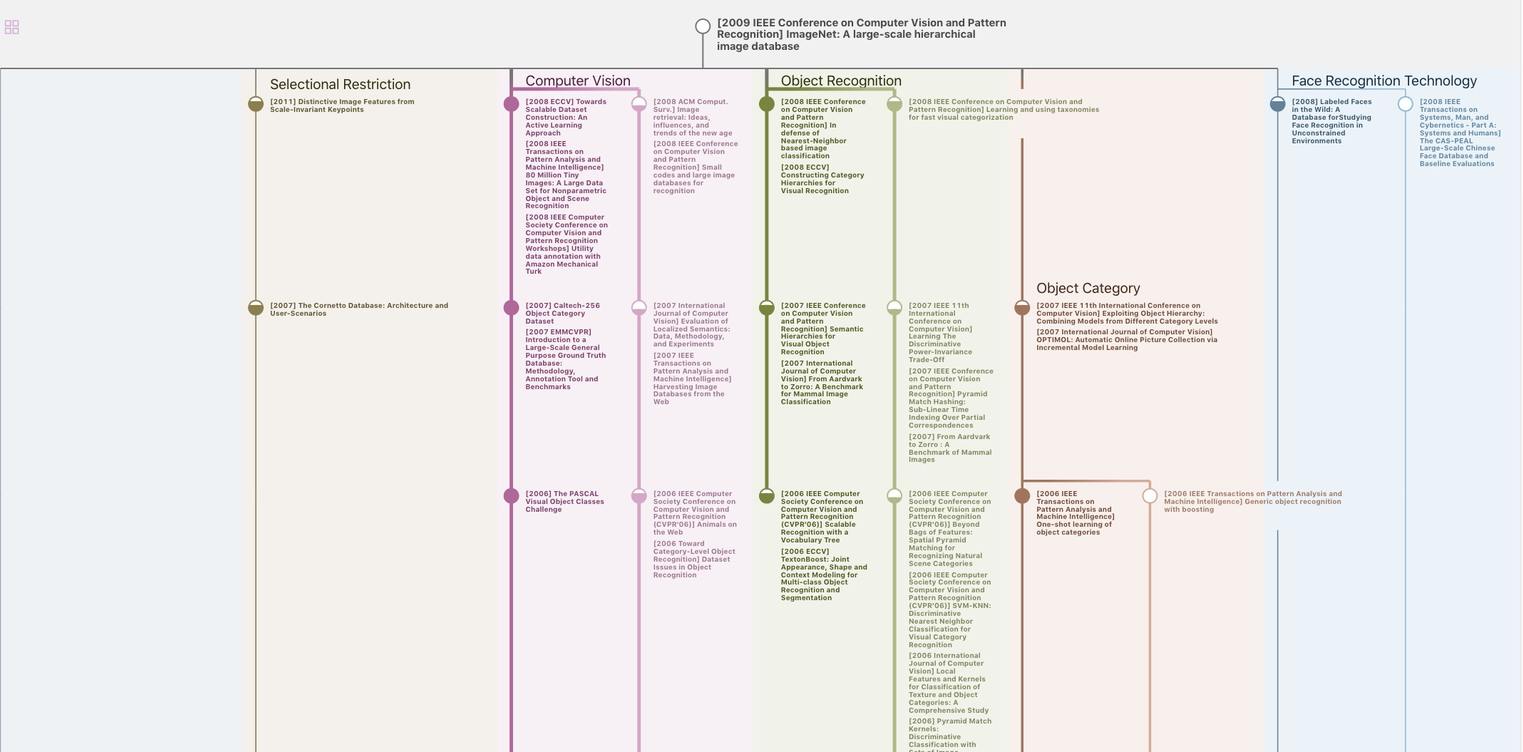
生成溯源树,研究论文发展脉络
Chat Paper
正在生成论文摘要