Joint Classification of Hyperspectral and LiDAR Data Using a Hierarchical CNN and Transformer
IEEE Transactions on Geoscience and Remote Sensing(2023)
摘要
The joint use of multisource remote-sensing (RS) data for Earth observation missions has drawn much attention. Although the fusion of several data sources can improve the accuracy of land-cover identification, many technical obstacles, such as disparate data structures, irrelevant physical characteristics, and a lack of training data, exist. In this article, a novel dual-branch method, consisting of a hierarchical convolutional neural network (CNN) and a transformer network, is proposed for fusing multisource heterogeneous information and improving joint classification performance. First, by combining the CNN with a transformer, the proposed dual-branch network can significantly capture and learn spectral–spatial features from hyperspectral image (HSI) data and elevation features from light detection and ranging (LiDAR) data. Then, to fuse these two sets of data features, a cross-token attention (CTA) fusion encoder is designed in a specialty. The well-designed deep hierarchical architecture takes full advantage of the powerful spatial context information extraction ability of the CNN and the strong long-range dependency modeling ability of the transformer network based on the self-attention (SA) mechanism. Four standard datasets are used in experiments to verify the effectiveness of the approach. The experimental results reveal that the proposed framework can perform noticeably better than state-of-the-art methods. The source code of the proposed method will be available publicly at
https://github.com/zgr6010/Fusion_HCT.git
.
更多查看译文
关键词
Convolutional neural network (CNN),hyperspectral image (HSI),joint classification,light detection and ranging (LiDAR) data,tokenization,transformer
AI 理解论文
溯源树
样例
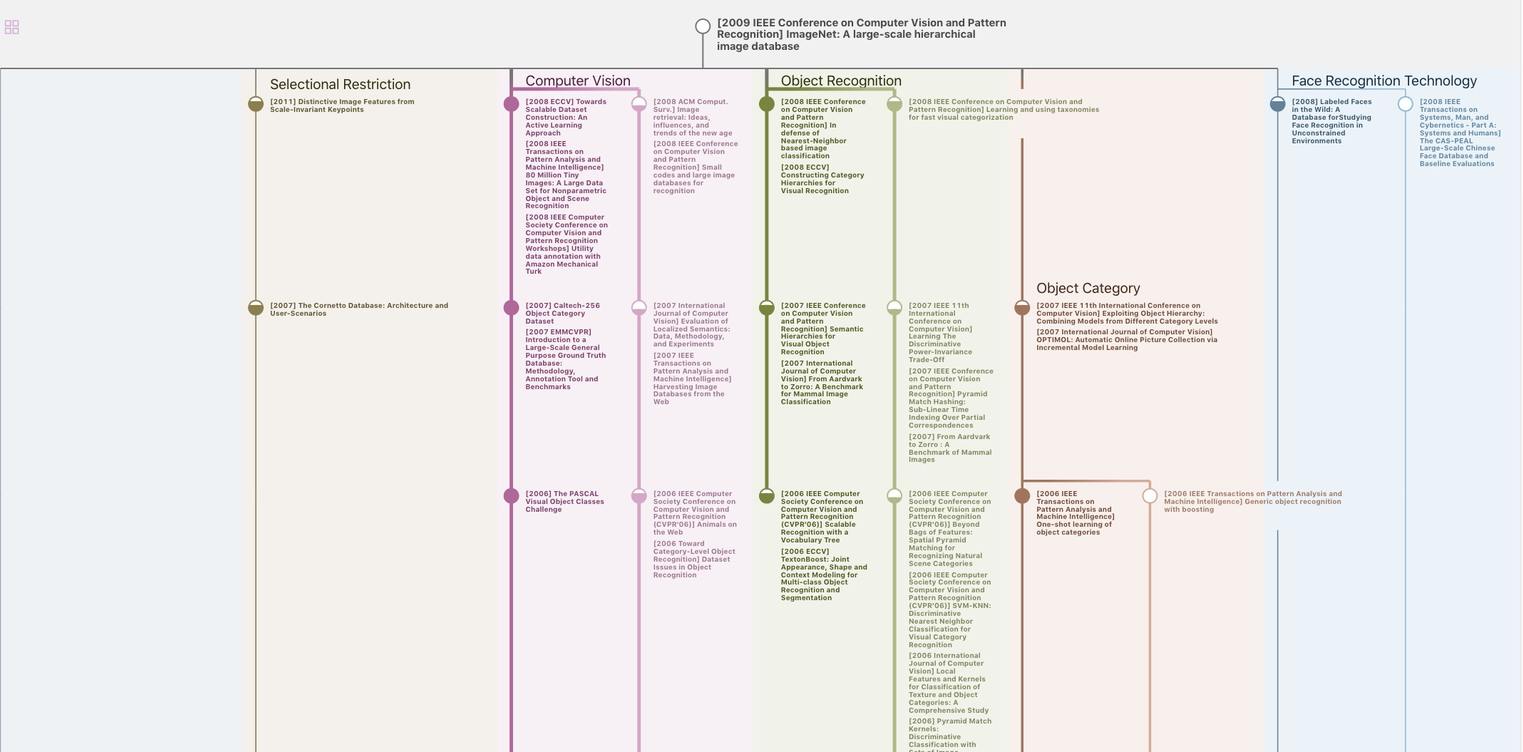
生成溯源树,研究论文发展脉络
Chat Paper
正在生成论文摘要