FedTeams: Towards Trust-Based and Resource-Aware Federated Learning
2022 IEEE International Conference on Cloud Computing Technology and Science (CloudCom)(2022)
摘要
Federated Learning (FL) has enabled Machine Learning (ML) applications to capture a larger spectrum of data by allowing such data to remain on-device, a desirable privacy guarantee in many applications. However, the highly iterative nature of FL optimization algorithms requires low-latency and high-throughput connections to clients. Unfortunately, realistic FL training scenarios include heterogeneous clients that are restricted by computation and communication, thereby slowing down or even failing FL training. In this paper, we propose FedTeams; a trust-based and resource-aware FL system that minimizes training latency, while improving accuracy. To achieve this, we mitigate the risk of straggling and weakly-connected clients by leveraging social trust and allowing these clients to offload their data to more powerful trusted peers that can train on their behalf. In specific, we formulate and solve an optimization problem that leverages the FedTeam’s trust graph and client resource information to optimize the distribution of training and minimize training latency. We evaluate FedTeams in a simulated environment, demonstrating up to a 81.6% decrease in training latency and 11.2% increase in global model accuracy when compared to existing state-of-the-art solutions.
更多查看译文
关键词
Federated Learning,Internet of Things,Edge Computing,Trust,Privacy
AI 理解论文
溯源树
样例
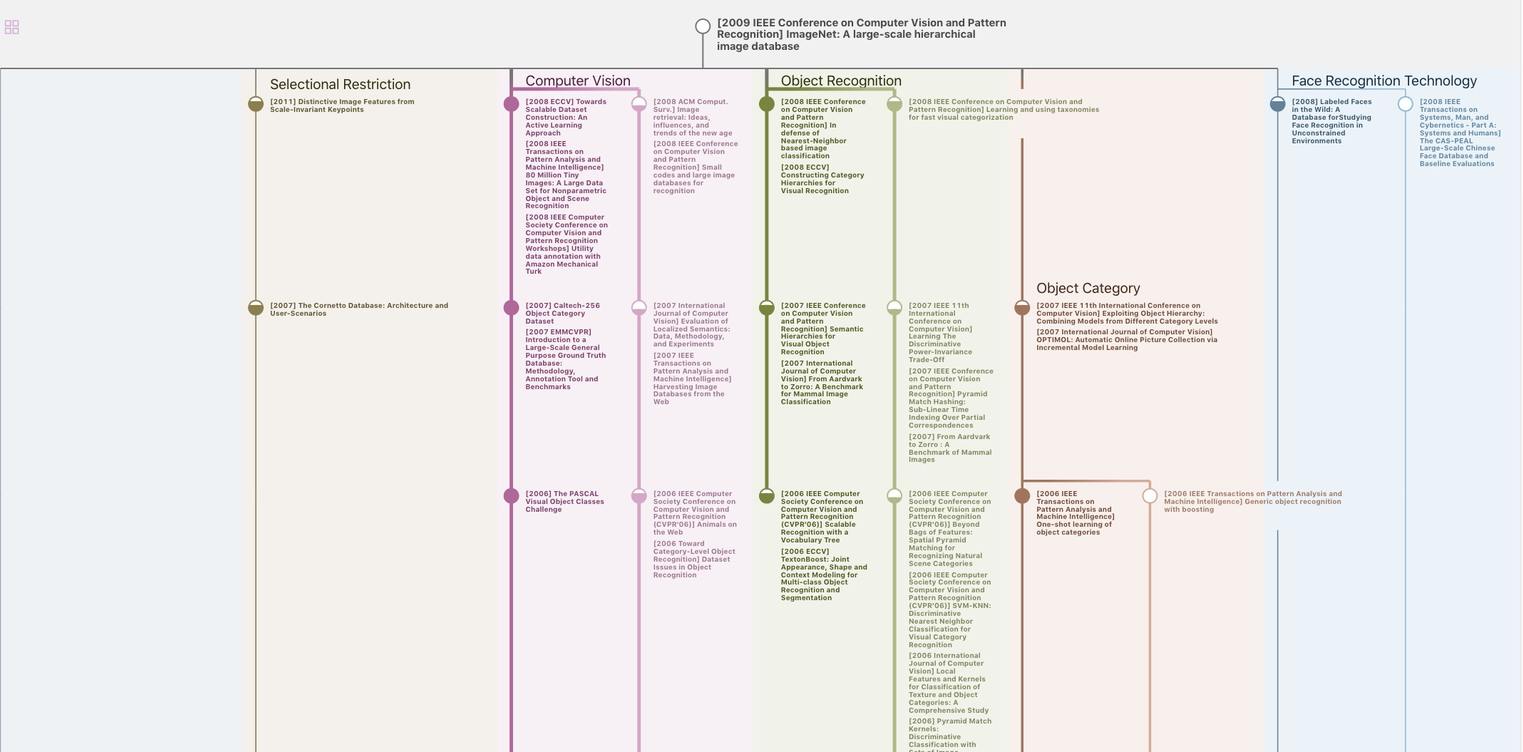
生成溯源树,研究论文发展脉络
Chat Paper
正在生成论文摘要