Decision making for autonomous vehicles in highway scenarios using Harmonic SK Deep SARSA
Applied Intelligence(2022)
摘要
The complexity of taking decisions for an autonomous vehicle (AV) to avoid road accident fatalities, provide safety, comfort, and reduce traffic raises the need for improvements in the field of decision making. To solve these challenges, many algorithms and techniques were applied, and the most common ones were reinforcement learning (RL) algorithms combined with deep learning techniques. Therefore, in this paper we proposed a novel extension of the popular “SARSA” (State-Action-Reward-State-Action) RL technique called “Harmonic SK Deep SARSA” that takes advantage of the stability which SARSA algorithm provides and uses the notion of similar and cumulative states saved in an alternative memory to enhance the stability of the algorithm and achieve remarkable performance that SARSA could not accomplish due to its on policy nature. Through the investigation of our novel extension the adaptability of the algorithm to unexpected situations during learning and to unforeseen changes in the environment was proved while reducing the computational load in the learning process and increasing the convergence rate that plays a key role in upgrading decision making application that require numerous real time consecutive decisions, including autonomous vehicles, industrial robots, gaming, aerial navigation... The novel algorithm was tested in a gym environment simulator called “Highway-env” with multiple highway situations (multiple lanes configurations, highway with dynamic number of lanes (from 4-lane to 2-lane, from 4-lane to 6-lane), merge) with numerous dynamic obstacles. For the purpose of comparison, we used a benchmark of cutting edge algorithms known for their prominent performance. The experimental results showed that the proposed algorithm outperformed the comparison algorithms in learning stability and performance that were validated by the following metrics: average loss value per episode, average accuracy per episode, maximum speed value reached per episode, average speed per episode, and the total reward per episode.
更多查看译文
关键词
Reinforcement learning, Deep learning, Human inspired meta-heuristics, Decision making, Autonomous vehicles
AI 理解论文
溯源树
样例
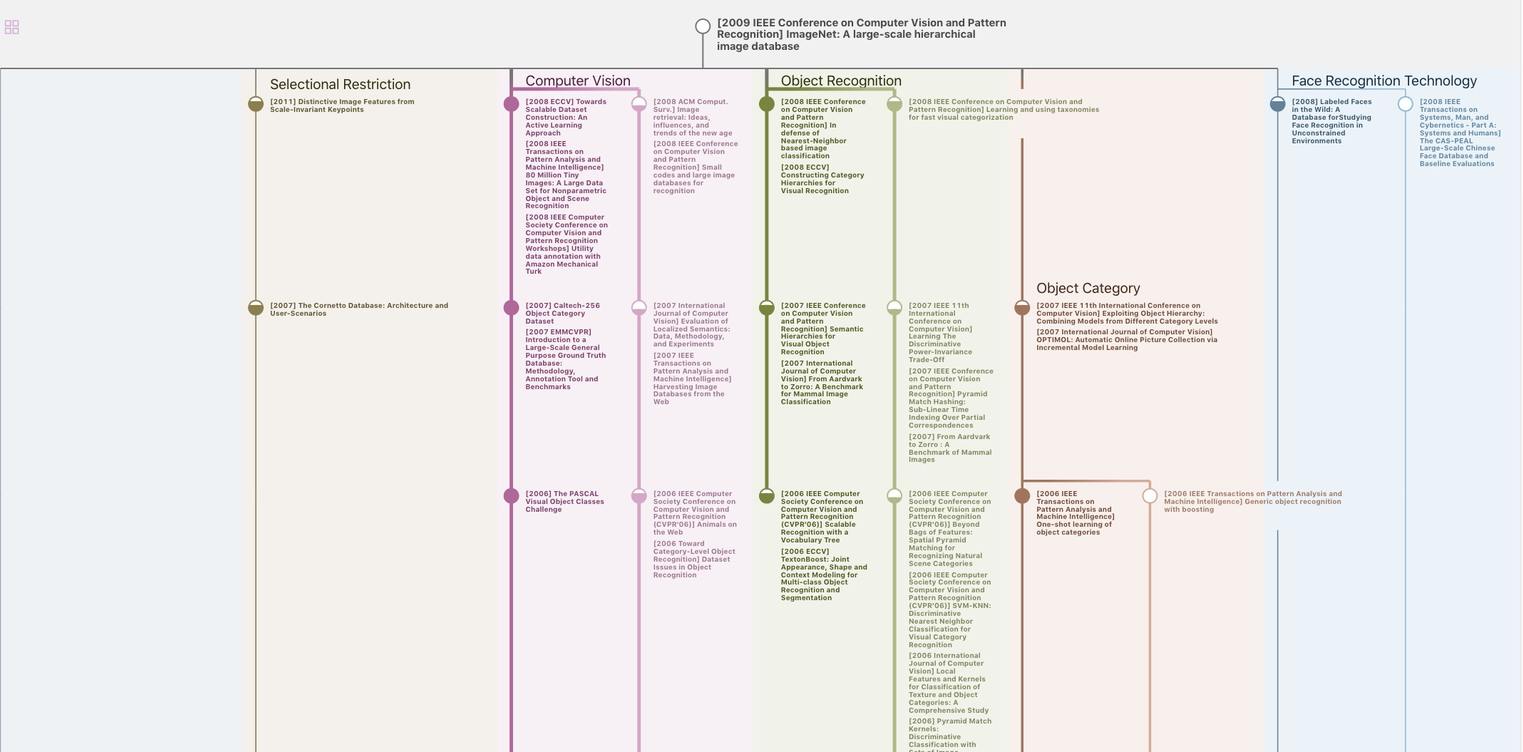
生成溯源树,研究论文发展脉络
Chat Paper
正在生成论文摘要