Nonlocal B-spline Representation of Tensor Decomposition for Hyperspectral Image Inpainting
Signal processing(2023)
摘要
Hyperspectral image (HSI) completion is a fundamental problem in image processing and remote sens -ing. Typical methods, either perform suboptimally due to lack of appropriate priors or suffer from ex-cessive complexity since the combination of too many constraints. Recently, nonlocal low-rank tensor approximation-based (NLTA) HSI completion methods are widespreadly investigated taking full advan-tage of global spectral correlation and non-local self-similarity. Common NLTA methods are mainly based on tensor train (TT) and tensor ring (TR) decompositions, but their representation of tensors is inade-quate and inflexible. In this paper, a fully-connected tensor network (FCTN) decomposition is introduced for HSI completion exploring both the global spectral correlation and the spatial structure. FCTN approx-imates an N-order tensor as a sequence of smaller sized N-order tensors, which has outstanding capabil-ity to explore the essential invariance for transposition and also to improve intrinsic correlations among factors. Furthermore, motivated by the transformation based methods on HSI reconstruction and after deeply comparing its superiority over other techniques, we employ an iterative B-spline representation to construct a transformed tensor for better revelation of prior information. The proposed model can be efficiently solved using proximal alternating minimization (PAM) approach. Extensive experiments results demonstrate that our method achieves state-of-the-art performance both in quantitative metrics and vi-sual effect evaluations.(c) 2022 Elsevier B.V. All rights reserved.
更多查看译文
关键词
Tensor completion,Fully connected tensor network decomposition,Low-rank,Framelet transform,Nonlocal self-similarity
AI 理解论文
溯源树
样例
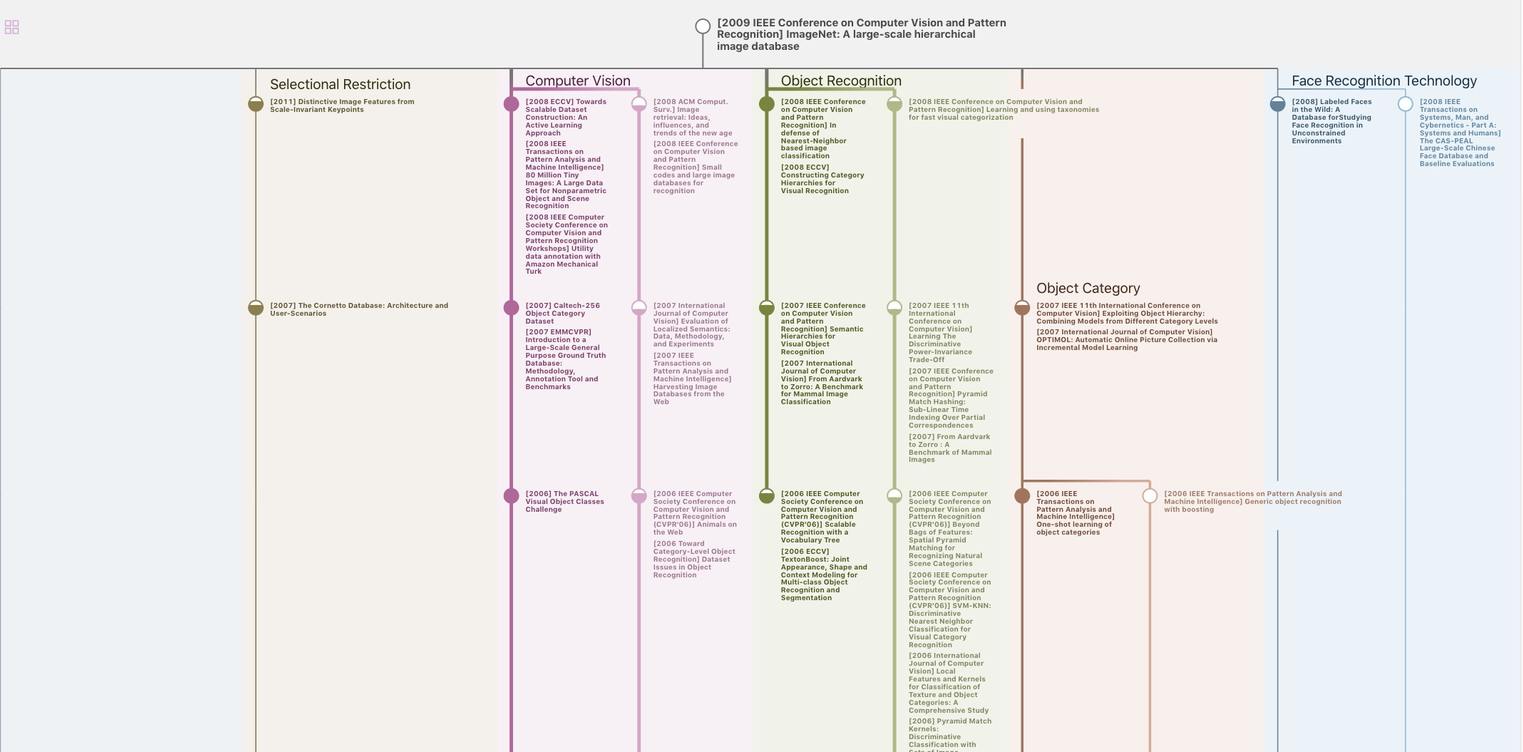
生成溯源树,研究论文发展脉络
Chat Paper
正在生成论文摘要