Methodology for multi-temporal prediction of crop rotations using recurrent neural networks
SMART AGRICULTURAL TECHNOLOGY(2023)
摘要
In a context of growing demand for food and the scarcity of natural resources, the development of more sustainable agriculture is imperative. This means it is necessary to limit the environmental impact of agricultural activities on soil and water and to be mindful of the carbon footprint, while maintaining crop yields and economic benefits for producers. Crop rotation is a valuable tool in sustainable agriculture, but this technique has to be appropriately coupled with sustainable fertilization plans to optimize crops. The proposed methodology uses recurrent neural networks (RNN); more precisely, LSTMs, in a Seq2Seq architecture, to predict the most probable scenarios of crop rotations to be exploited in a field in subsequent growing seasons, according to cropping habits. The output can be used in crop models to build a decision support system for greater sustainability in agricultural production by allowing producers to choose the strategy that offers the best compromise between profitability and environmental impact.
更多查看译文
关键词
Agriculture 4.0,Crop rotation,Deep learning,Seq2Seq,LSTM
AI 理解论文
溯源树
样例
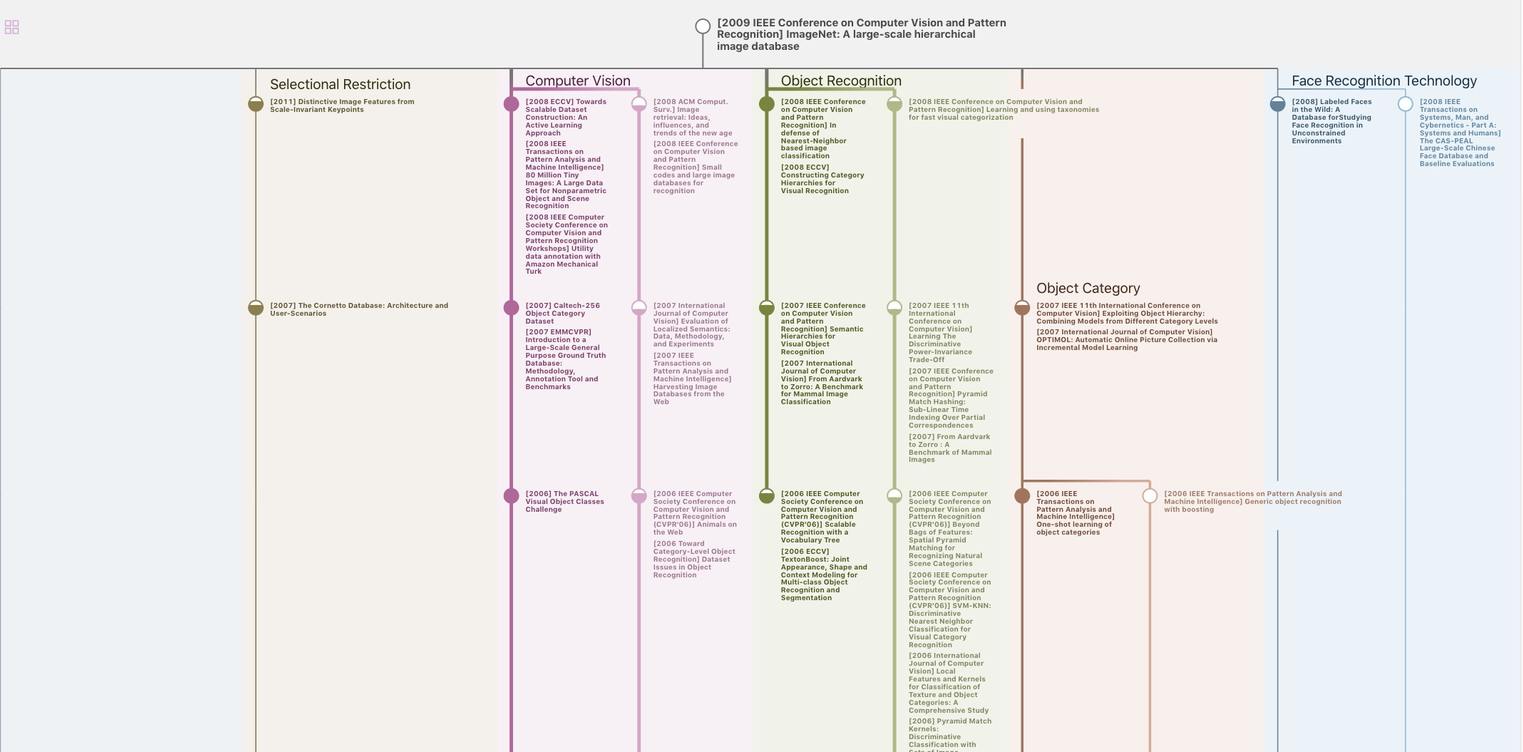
生成溯源树,研究论文发展脉络
Chat Paper
正在生成论文摘要