Unlocking de novo antibody design with generative artificial intelligence
biorxiv(2024)
摘要
Generative AI has the potential to redefine the process of therapeutic antibody discovery. In this report, we describe and validate deep generative models for the de novo design of antibodies against human epidermal growth factor receptor (HER2) without additional optimization. The models enabled an efficient workflow that combined in silico design methods with high- throughput experimental techniques to rapidly identify binders from a library of ~10^6 heavy chain complementarity-determining region (HCDR) variants. We demonstrated that the workflow achieves binding rates of 10.6% for HCDR3 and 1.8% for HCDR123 designs and is statistically superior to baselines. We further characterized 421 diverse binders using surface plasmon resonance (SPR), finding 71 with low nanomolar affinity similar to the therapeutic anti-HER2 antibody trastuzumab. A selected subset of 11 diverse high-affinity binders were functionally equivalent or superior to trastuzumab, with most demonstrating suitable developability features. We designed one binder with ~3x higher cell-based potency compared to trastuzumab and another with improved cross-species reactivity. Our generative AI approach unlocks an accelerated path to designing therapeutic antibodies against diverse targets.
### Competing Interest Statement
The authors are current or former employees, contractors, interns, or executives of Absci Corporation and may hold shares in Absci Corporation. Methods and compositions described in this manuscript are the subject of one or more pending patent applications.
更多查看译文
AI 理解论文
溯源树
样例
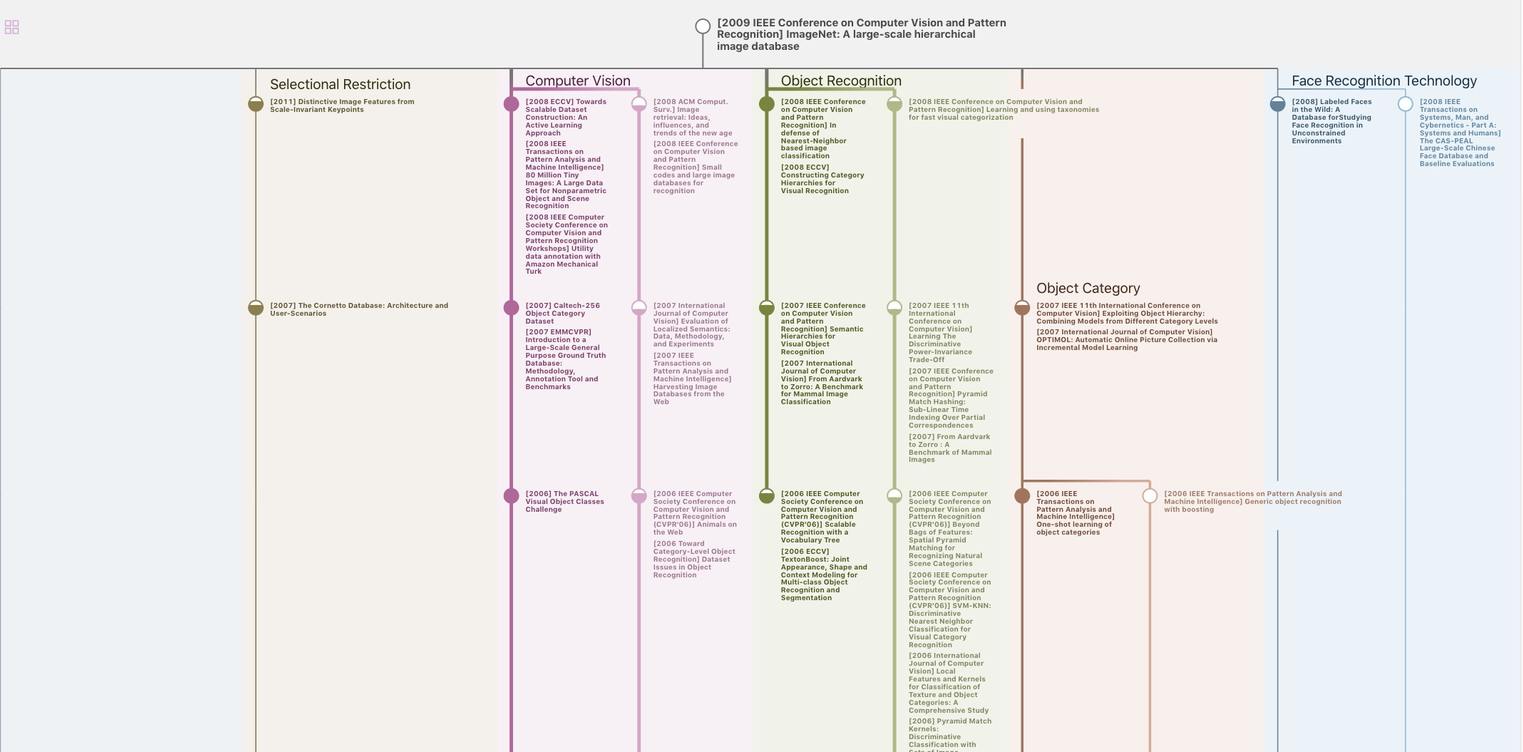
生成溯源树,研究论文发展脉络
Chat Paper
正在生成论文摘要