Weakly-supervised temporal segmentation of cell-cycle stages with center-cell focus using Recurrent Neural Networks
Bildverarbeitung für die Medizin(2023)
摘要
Training deep-learning models for biomedical images has always been a problem because of the unavailability of annotated training data. Here we propose using a model and a training approach for the weakly-supervised temporal classification of cell-cycle stages during mitosis. Instead of using annotated data, by using an ordered set of classes called transcripts, our proposed approach classifies the cell-cycle stages of cell video sequences. The network design helps to propagate information in time using Recurrent Neural Network layers and helps to focus the features on the center-cell using two loss functions. The proposed system is evaluated on four datasets from LiveCellMiner and has a comparable performance with the supervised approaches, considering the annotated data is not used for training. The predicted classification of the unannotated interphase-recovery class by our approach also aligns with the findings from the LiveCellMiner.
### Competing Interest Statement
The authors have declared no competing interest.
更多查看译文
关键词
temporal segmentation,recurrent neural networks,weakly-supervised,cell-cycle,center-cell
AI 理解论文
溯源树
样例
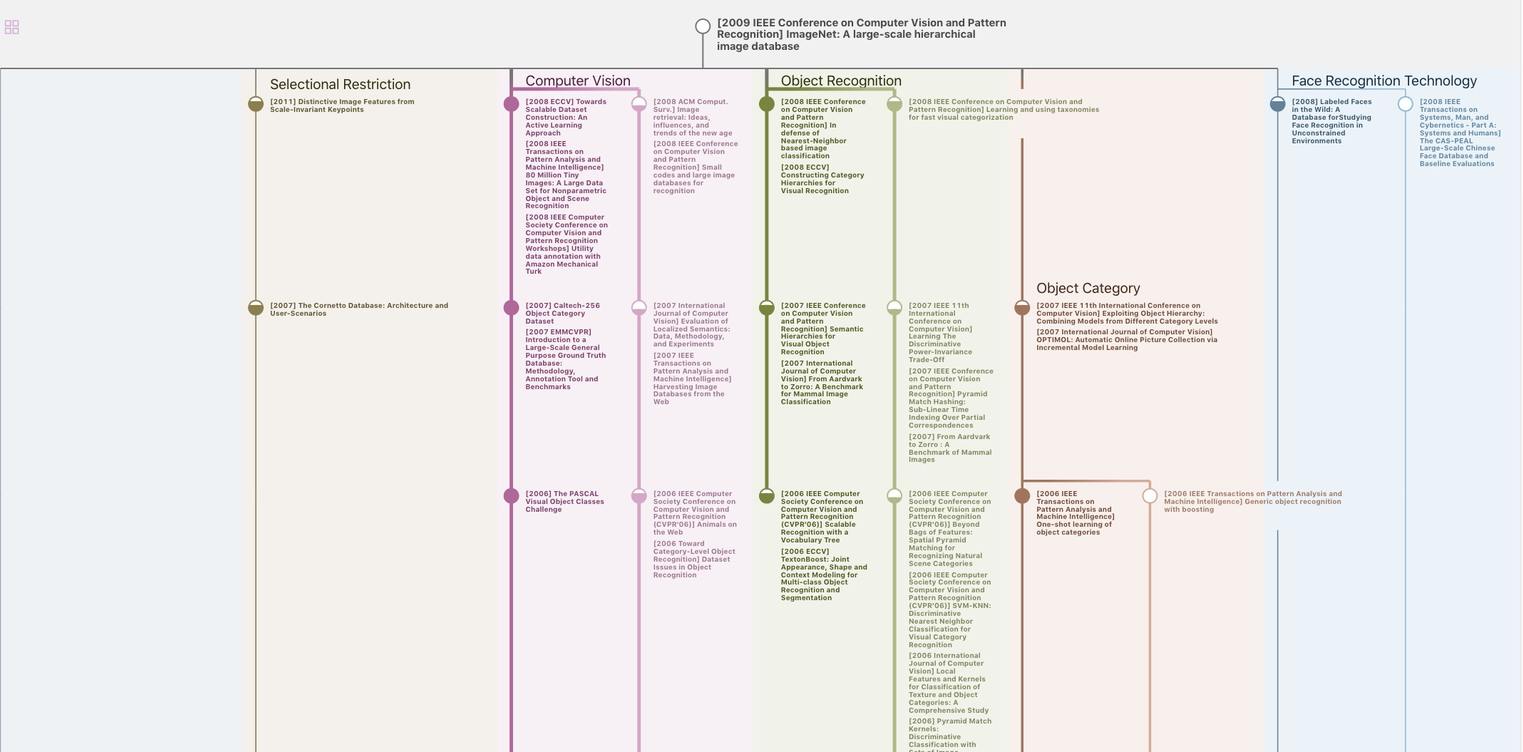
生成溯源树,研究论文发展脉络
Chat Paper
正在生成论文摘要