Predicting and explaining performance and diversity of neural network architecture for semantic segmentation
Expert Systems with Applications(2023)
摘要
This paper proposes searching for network architectures which achieve similar performance while promoting diversity, in order to facilitate ensembling. We explain prediction performance and diversity of various network sizes and activation functions applied to semantic segmentation of the CityScapes dataset. We show that both performance and diversity can be predicted from neural network architecture using explainable boosting machines. A grid search of 144 models is performed, and many of the models exhibit no significant difference in mean performance within a 95% confidence interval. Notably, we find the best performing models have varied network architecture parameters. The explanations for performance largely agree with the accepted wisdom of the machine learning community, which shows that the method is extracting information of value. We find that diversity between models can be achieved by varying network size. Moreover, homogeneous network sizes generally show positive correlation in predictions, and larger models tend to converge to similar solutions. These explanations provide a better understanding of the effects of network parameters to deep learning practitioners; they could also be used in place of naïve search methods or a model pool to inform growing an ensemble.
更多查看译文
关键词
Ensembles,Diversity,Semantic segmentation,Computer vision
AI 理解论文
溯源树
样例
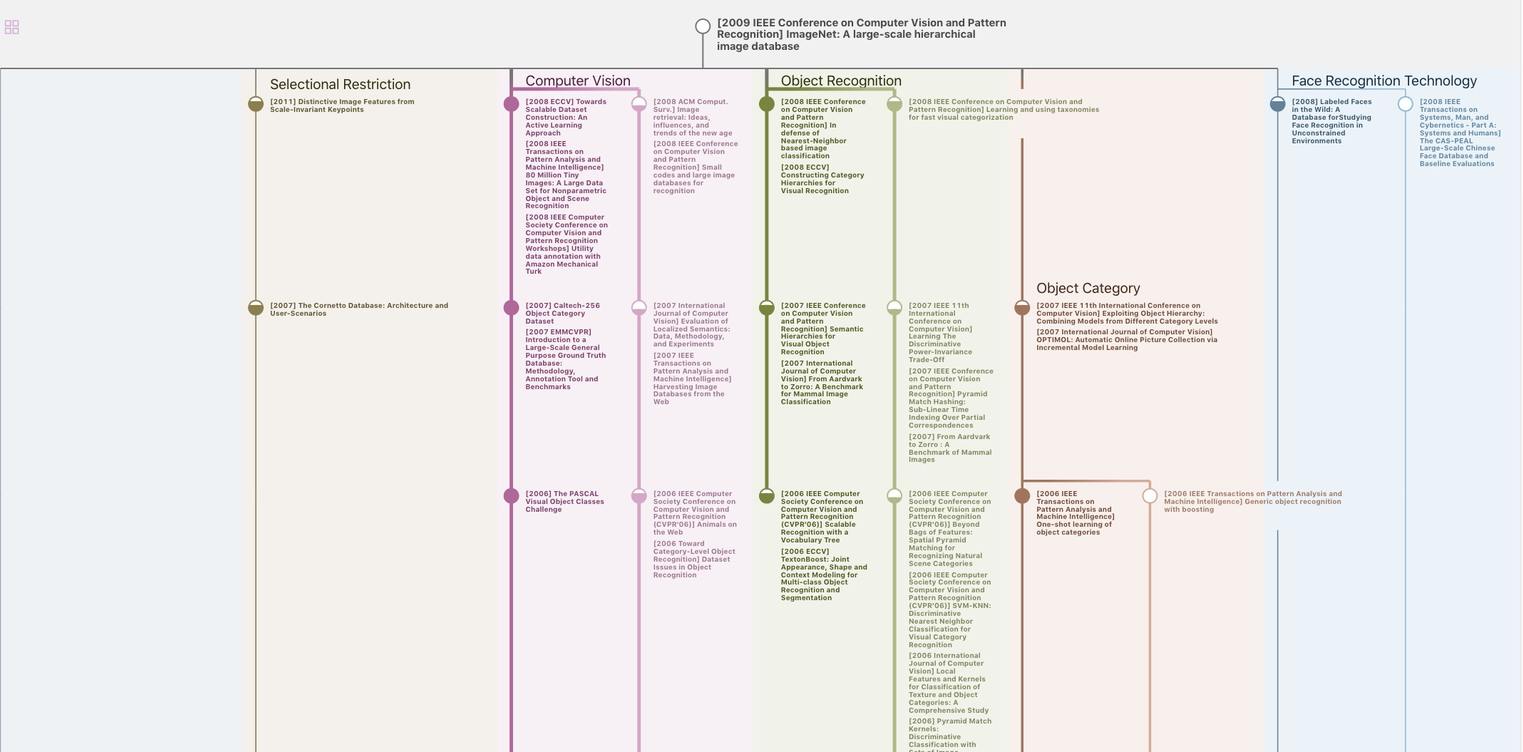
生成溯源树,研究论文发展脉络
Chat Paper
正在生成论文摘要