Schlably: A Python Framework for Deep Reinforcement Learning Based Scheduling Experiments
SOFTWAREX(2023)
摘要
Research on deep reinforcement learning (DRL) based production scheduling (PS) has gained a lot of attention in recent years, primarily due to the high demand for optimizing scheduling problems in diverse industry settings. Numerous studies are carried out and published as stand-alone experiments that often vary only slightly with respect to problem setups and solution approaches. The programmatic core of these experiments is typically very similar. Despite this fact, no standardized and resilient framework for experimentation on PS problems with DRL algorithms could be established so far. In this paper, we introduce schlably, a Python-based framework that provides researchers a comprehensive toolset to facilitate the development of PS solution strategies based on DRL. schlably eliminates the redundant overhead work that the creation of a sturdy and flexible backbone requires and increases the comparability and reusability of conducted research work.
更多查看译文
关键词
Production scheduling,Deep reinforcement learning,Python,Framework
AI 理解论文
溯源树
样例
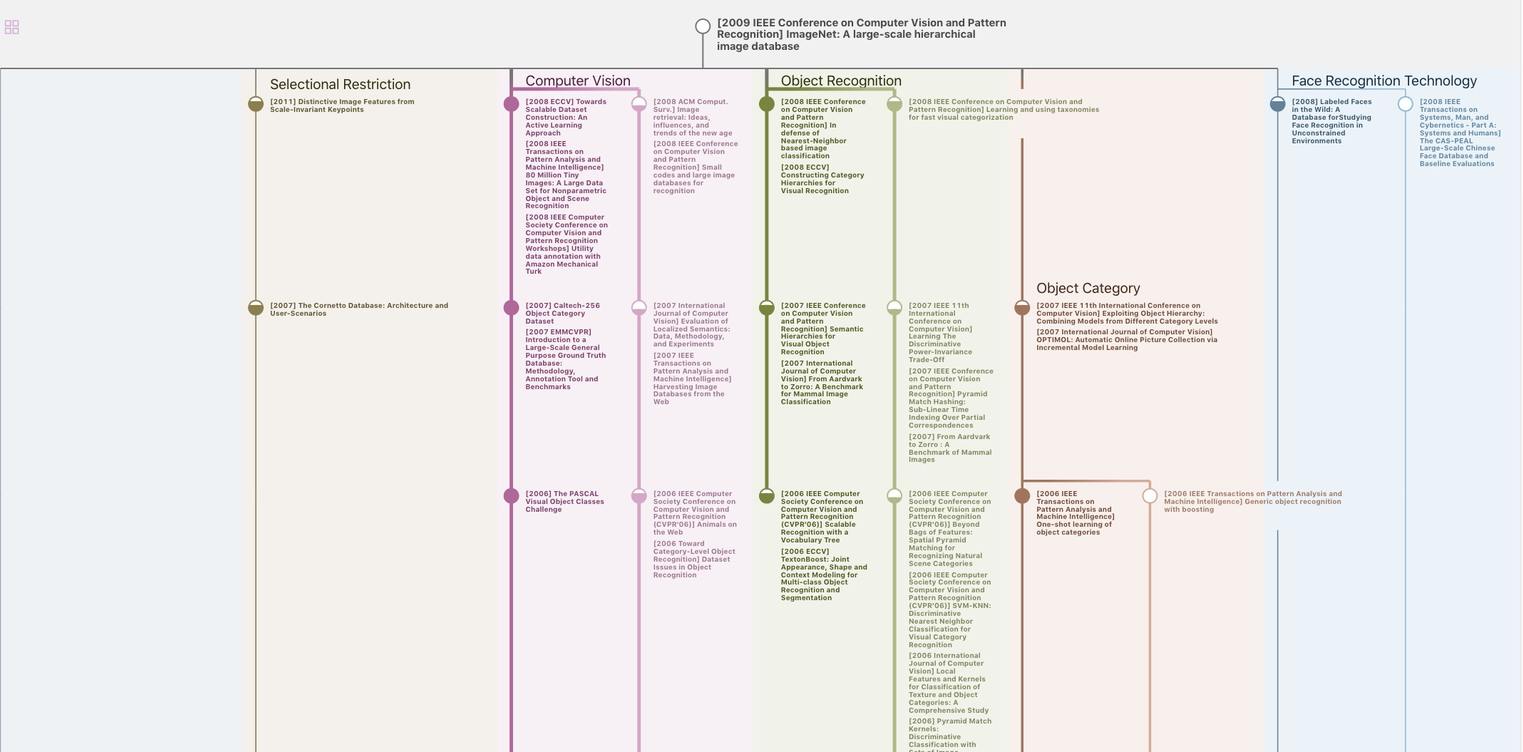
生成溯源树,研究论文发展脉络
Chat Paper
正在生成论文摘要