Dual Learning for Large Vocabulary On-Device ASR
2022 IEEE Spoken Language Technology Workshop (SLT)(2023)
摘要
Dual learning is a paradigm for semi-supervised machine learning that seeks to leverage unsupervised data by solving two opposite tasks at once. In this scheme, each model is used to generate pseudo-labels for unlabeled examples that are used to train the other model. Dual learning has seen some use in speech processing by pairing ASR and TTS as dual tasks. However, these results mostly address only the case of using unpaired examples to compensate for very small supervised datasets, and mostly on large, non-streaming models. Dual learning has not yet been proven effective for using unsupervised data to improve realistic on-device streaming models that are already trained on large supervised corpora. We provide this missing piece though an analysis of an on-device-sized streaming conformer trained on the entirety of Librispeech, showing relative WER improvements of 10.7%/5.2% without an LM and 11.7%/16.4% with an LM.
更多查看译文
关键词
dual learning,large vocabulary,on-device
AI 理解论文
溯源树
样例
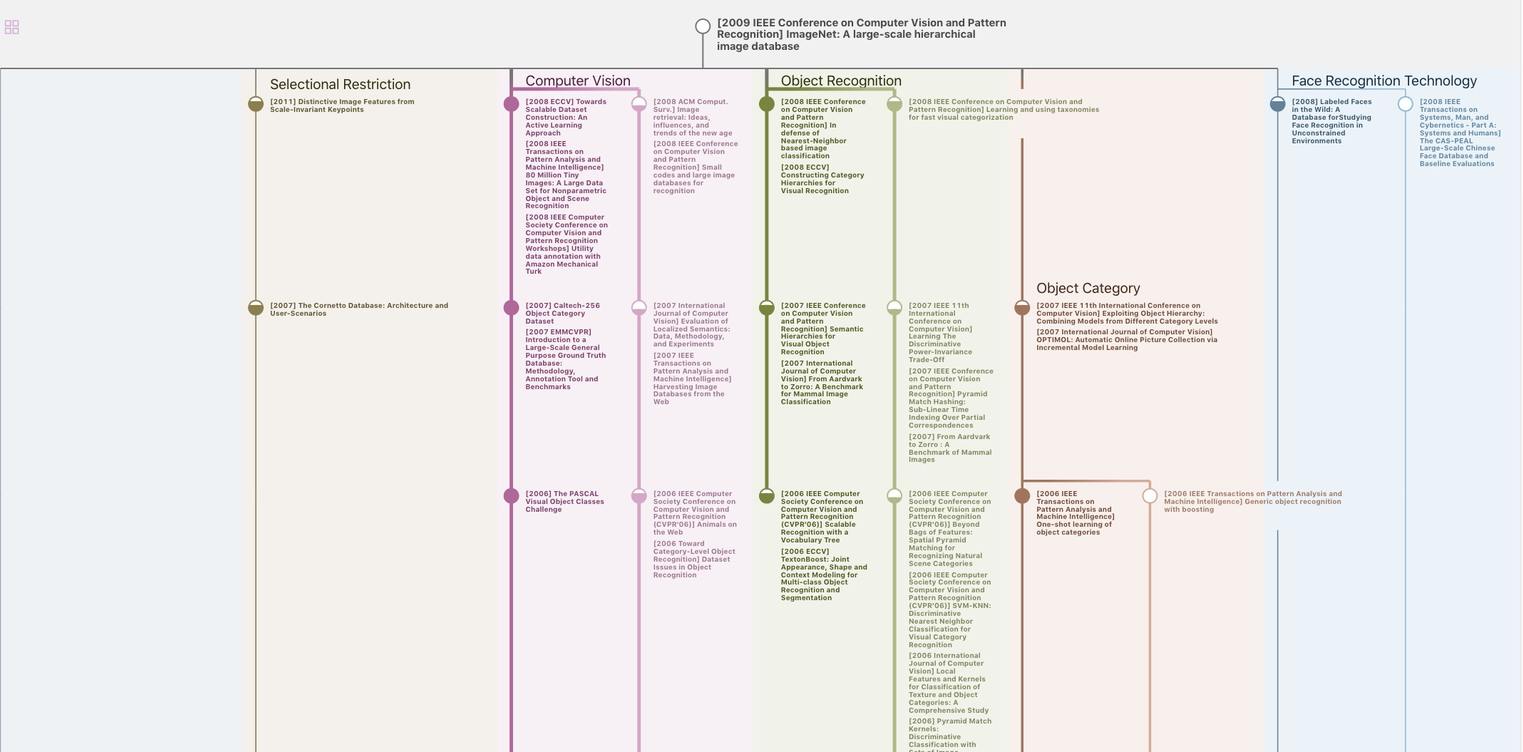
生成溯源树,研究论文发展脉络
Chat Paper
正在生成论文摘要