Multiple-level Point Embedding for Solving Human Trajectory Imputation with Prediction
arxiv(2023)
摘要
Sparsity is a common issue in many trajectory datasets, including human mobility data. This issue frequently brings more difficulty to relevant learning tasks, such as trajectory imputation and prediction. Nowadays, little existing work simultaneously deals with imputation and prediction on human trajectories. This work plans to explore whether the learning process of imputation and prediction could benefit from each other to achieve better outcomes. And the question will be answered by studying the coexistence patterns between missing points and observed ones in incomplete trajectories. More specifically, the proposed model develops an imputation component based on the self-attention mechanism to capture the coexistence patterns between observations and missing points among encoder-decoder layers. Meanwhile, a recurrent unit is integrated to extract the sequential embeddings from newly imputed sequences for predicting the following location. Furthermore, a new implementation called Imputation Cycle is introduced to enable gradual imputation with prediction enhancement at multiple levels, which helps to accelerate the speed of convergence. The experimental results on three different real-world mobility datasets show that the proposed approach has significant advantages over the competitive baselines across both imputation and prediction tasks in terms of accuracy and stability.
更多查看译文
关键词
human trajectory imputation,point,prediction,multiple-level
AI 理解论文
溯源树
样例
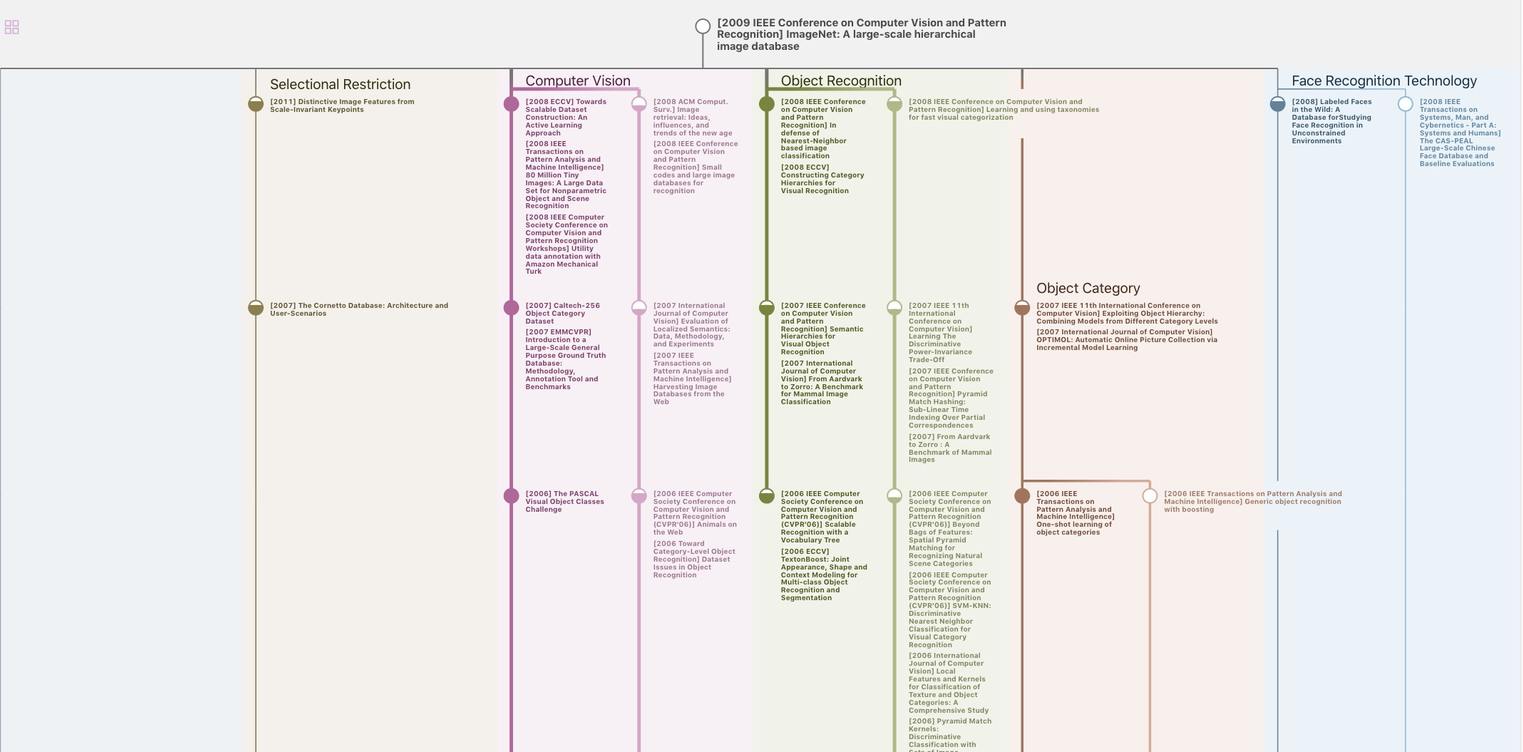
生成溯源树,研究论文发展脉络
Chat Paper
正在生成论文摘要