Influence of Uncoordinated N Content on Ni-N-C Electrocatalyst for Co2 Reduction: Combining First Principle with Machine Learning
Fuel(2023)
摘要
Electrocatalysis of CO2 reduction reaction (CO2 RR) has attracted elevated attentions since it is favorable to prompt the carbon cycle in a sustainable pathway. However, the applications of this technology is obstructed by the low activity and selectivity due to the inert of CO2 and favored hydrogen evolution reaction. Development of robust catalysts is the most feasible method to decrease the overpotential and suppress the competitive side reaction. Single-atom-catalysts (SACs) is one of the most promising catalysts with the satisfied activity. However, the clear and popular rules are still absence to explore the SACs with the desired properties due to the complicated items related with the overall performance. The suitable transition metals (TMs) are screened out based on the defective g-C3N4 (V-N-C3N4) supporter by the first principle. Among 24 screened transition metals, the Ni-V-N-C3N4 exhibits the better performance as compared to others. Later, the machine learning (ML) algorithm builds a bridge between catalytic activity and intrinsic structural and atomic features. Then, the same coordination environment is constructed on the graphene to explore the possibility to replace the V-N-C3N4 with other carbon substrate. When the doped amount of uncoordinated N reaches 25 wt%, the catalytic activity of Ni-N-C SAC based on the graphene is close to that based on the V-N-C3N4. This work introduces a method to construct the SACs by regulating the N-doped amount on the supporter.
更多查看译文
关键词
CO2 reduction,Defective g-C3N4,Single-atom-catalyst,First principle,Machine learning
AI 理解论文
溯源树
样例
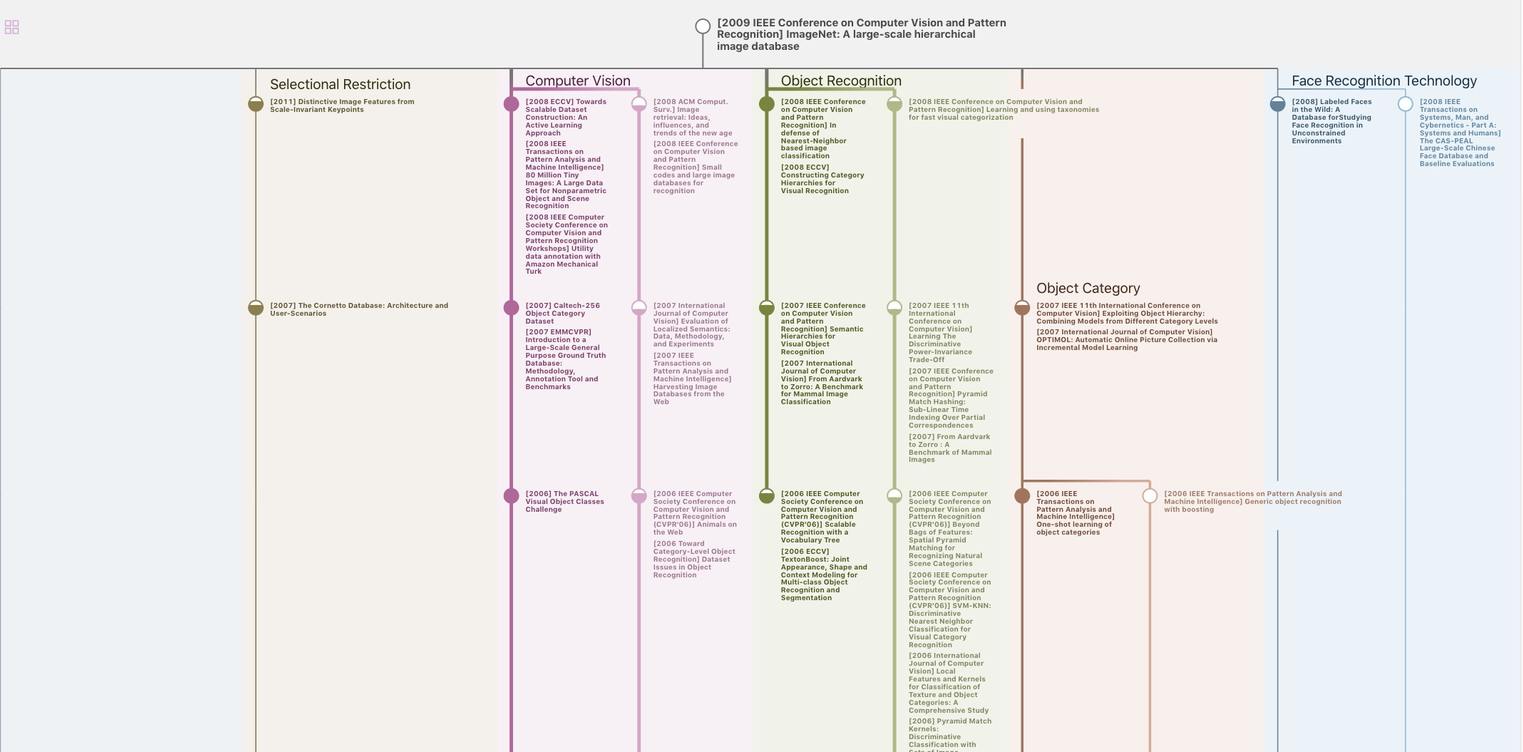
生成溯源树,研究论文发展脉络
Chat Paper
正在生成论文摘要