DeGTeC: A deep graph-temporal clustering framework for data-parallel job characterization in data centers
Future Generation Computer Systems(2022)
摘要
Complex data-parallel job contains task dependency information defined as Directed Acyclic Graph (DAG). For convenience, the DAG presented data-parallel jobs are named as DAG jobs. The prevalence of DAG jobs in modern data centers has made the scheduling oriented job characterization a big challenge. This paper proposes a deep graph-temporal clustering framework, i.e., DeGTeC, to efficiently categorize DAG jobs leveraging the graphic and temporal information in DAGs. The categorization result can then be naturally used to characterize the resource consumption pattern of DAG jobs. The DeGTeC framework is constructed mainly based on two autoencoders, i.e., TaskAE and JobAE. TaskAE and JobAE contain spectral graph convolutional network (GCN) layers, temporal convolutional network (TCN) layers, and the adaptive pooling layers to help build task embeddings and job embeddings. An extra embedding sorting step takes in the sequential order information and the depth-bias information for job clustering. To our best knowledge, DeGTeC is the first solution to do resource consumption characterization of DAG jobs fully leveraging the task dependencies defined in DAG. Experimental results demonstrate that the DeGTeC framework outperforms the state-of-the-art job resource consumption characterization methods.
更多查看译文
关键词
Data center,Data-parallel job,Clustering,Autoencoder,Graph convolutional network,Temporal convolutional network
AI 理解论文
溯源树
样例
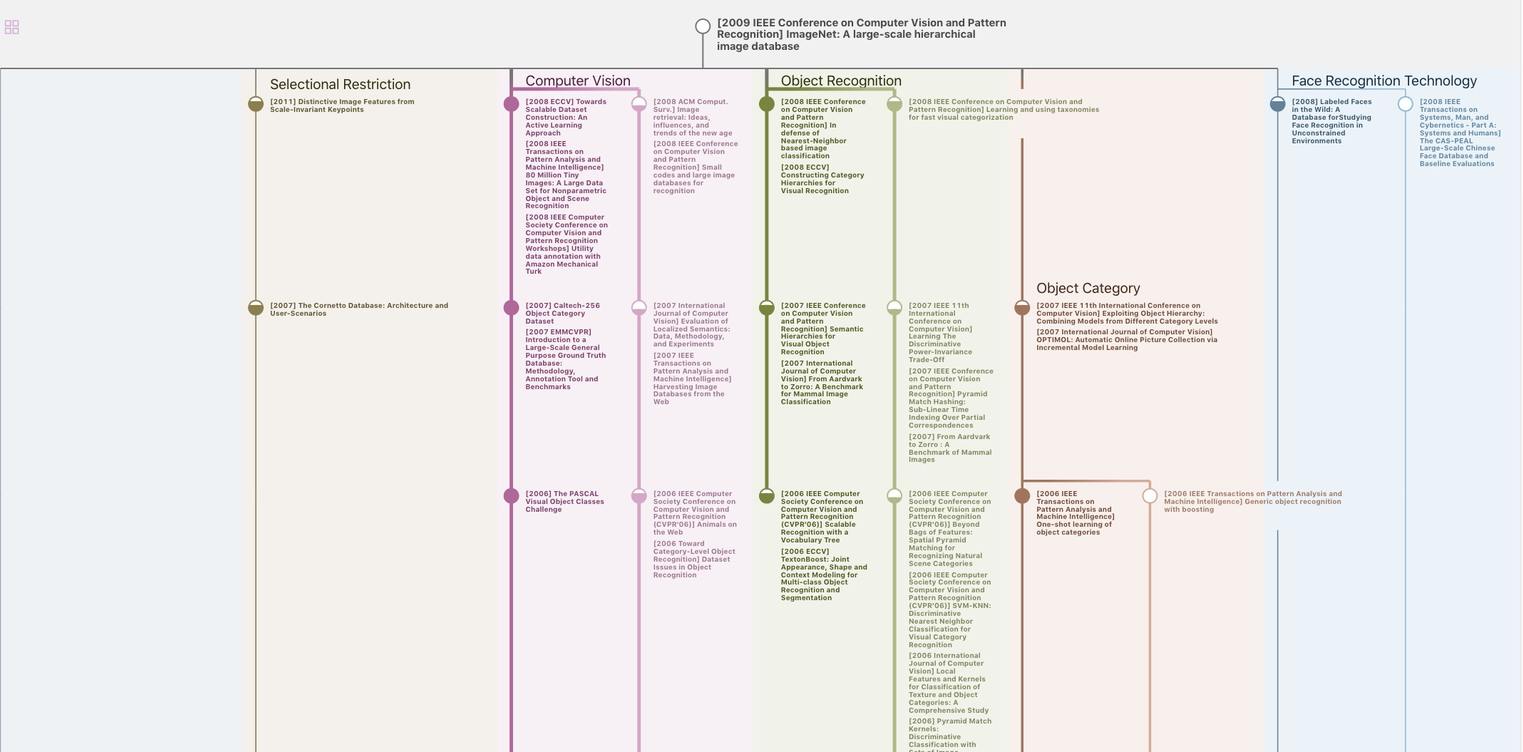
生成溯源树,研究论文发展脉络
Chat Paper
正在生成论文摘要