An accelerated active learning Kriging model with the distance-based subdomain and a new stopping criterion for reliability analysis
Reliability Engineering & System Safety(2023)
摘要
Reliability analysis for computationally expensive models is a challenging problem. Monte Carlo simulation is commonly employed in conjunction with the active learning assisted Kriging model (AK-MCS), which can significantly reduce the number of model evaluations. However, updating a Kriging model with numerous samples is time-consuming, especially for the small failure probability estimation. To alleviate the computational effort induced by the active learning process, the distance-based subdomain is first developed to select the candidate points in the vicinity of the limit state surface. Accordingly, a Kriging model is trained within distance-based subdomains and Kriging predictions on the whole population can be avoided. On the other hand, to further mitigate the computational effort caused by time-demanding model evaluations, a new stopping criterion is formulated based on the expected upper bound of the relative error of failure probability. Furthermore, a reasonable target threshold for the new stopping criterion is suggested based on the quantification of the effect of the selected threshold on the relative error of failure probability. Two analytical performance functions and three numerical models including a 61-bar truss, a reinforced concrete planar frame and a bolted steel beam–column joint are investigated to demonstrate the efficiency and accuracy of the proposed approach.
更多查看译文
关键词
Reliability analysis,Active learning,Kriging model,Distance-based subdomain,Small failure probability
AI 理解论文
溯源树
样例
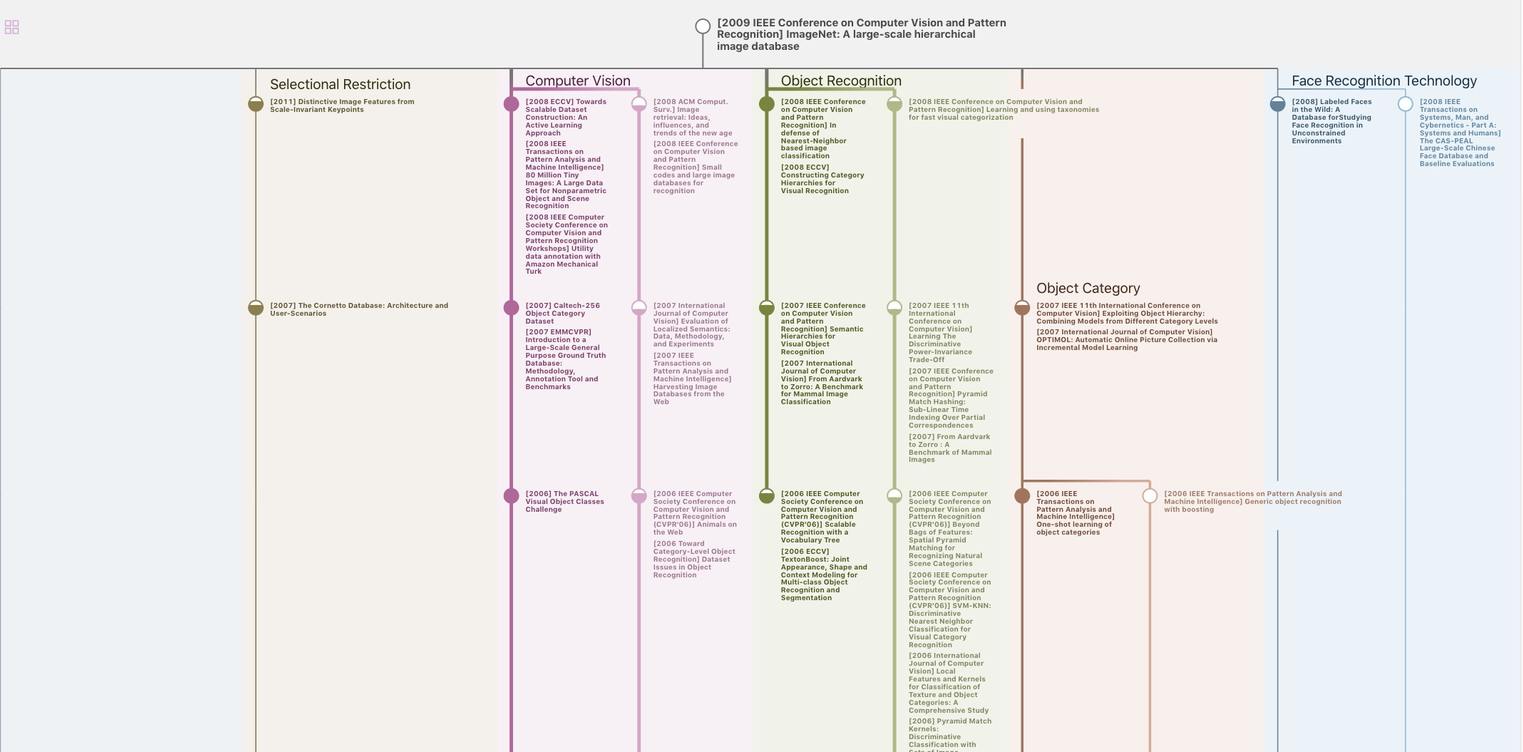
生成溯源树,研究论文发展脉络
Chat Paper
正在生成论文摘要