The Effect of the ADASYN Method on Widespread Metrics of Machine Learning Efficiency
Современные информационные технологии и IT-образование(2019)
摘要
The article presents the results of experimental work comparing the performance metrics of machine learning algorithms on imbalanced text corpora using the method of synthetic data generation ADASYN and without it. The work was carried out on an imbalanced corpus, consisting of 5,211 news texts, formed by cluster sampling for one year. The corpus annotation is produced according to the indicators of the tonality of texts by categories: neutral, positive, and negative, with a significant predominance of articles of neutral tone. There are many widely used methods to overcome the problem of data imbalance. Often, when working with imbalanced data, the resulting accuracy provides acceptable results, but other performance indicators are low. Such contradictory results usually occur when it comes to in-depth analysis of the text in the study of social or medical phenomena. This paper shows how performance metrics of the same machine learning algorithms change when using the ADASYN method while analyzing an imbalanced text corpus using the K-nearest neighbors’ method and Naive Bayes. The study considers the issue of the application of the method and its results in solving the problem of text classification. Comparative characteristics of the machine algorithms operation before and after the application of ADASYN provide a researcher with a better understanding of which machine learning performance metrics are more suitable when working with imbalanced data. As a result, authors present the observations and conclusions about the features of the method and put forward a number of proposals for further research in this area to compare the results obtained with the effects of the application of another method.
更多查看译文
关键词
adasyn,data imbalance,machine learning,performance metrics,accuracy
AI 理解论文
溯源树
样例
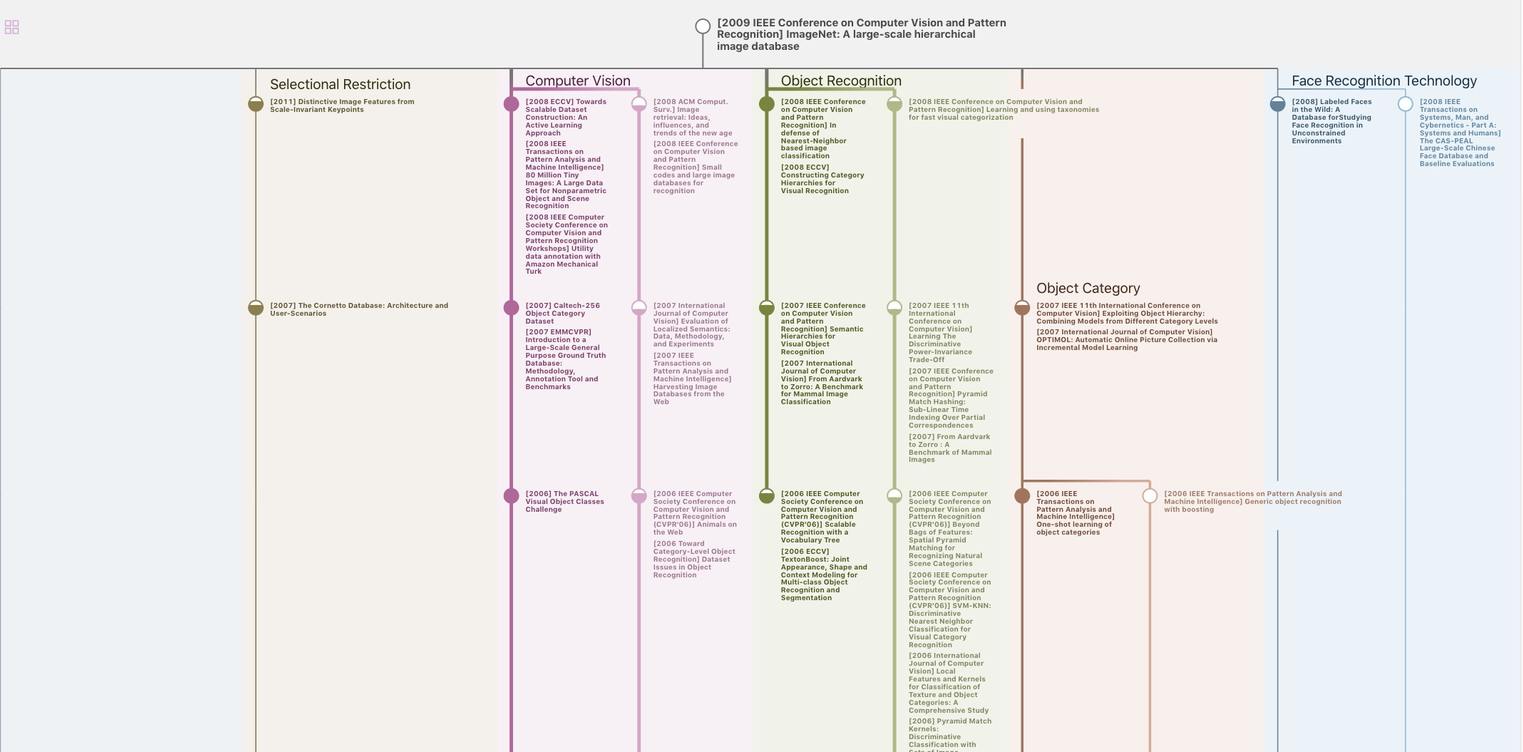
生成溯源树,研究论文发展脉络
Chat Paper
正在生成论文摘要