Interpretation of multi-task clearance models from molecular images supported by experimental design
Artificial Intelligence in the Life Sciences(2022)
摘要
Recent methodological advances in deep learning (DL) architectures have not only improved the performance of predictive models but also enhanced their interpretability potential, thus considerably increasing their transparency. In the context of medicinal chemistry, the potential to not only accurately predict molecular properties, but also chemically interpret them, would be strongly preferred. Previously, we developed accurate multi-task convolutional neural network (CNN) and graph convolutional neural network (GCNN) models to predict a set of diverse intrinsic metabolic clearance parameters from image- and graph-based molecular representations, respectively. Herein, we introduce several model interpretability frameworks to answer whether the model explanations obtained from CNN and GCNN multi-task clearance models could be applied to predict chemical transformations associated with experimentally confirmed metabolic products. We show a strong correlation between the CNN pixel intensities and corresponding clearance predictions, as well as their robustness to different molecular orientations. Using actual case examples, we demonstrate that both CNN and GCNN interpretations frequently complement each other, suggesting their high potential for combined use in guiding medicinal chemistry design.
更多查看译文
关键词
molecular images,models,multi-task
AI 理解论文
溯源树
样例
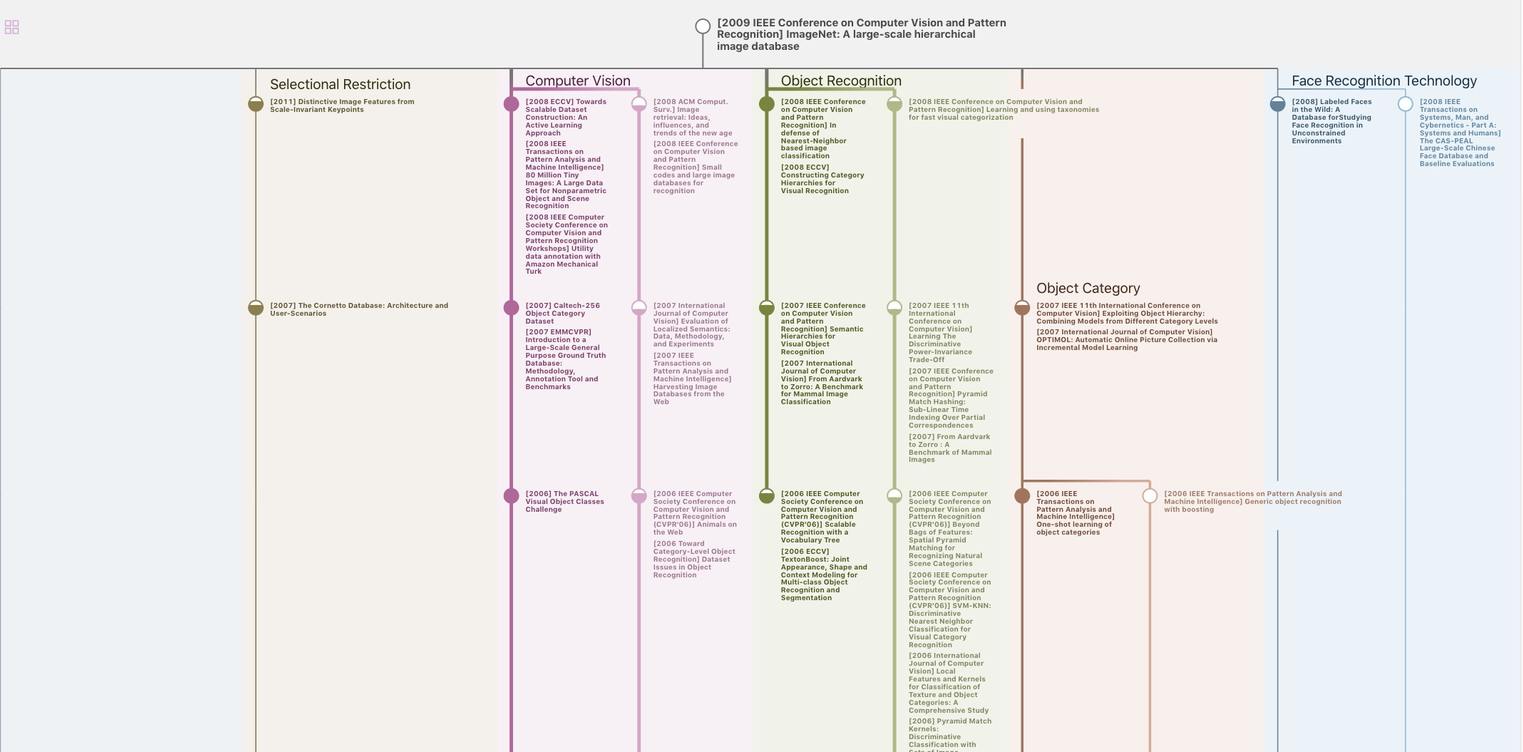
生成溯源树,研究论文发展脉络
Chat Paper
正在生成论文摘要