Short-Term Load Forecasting with an Ensemble Model Using Densely Residual Block and Bi-LSTM Based on the Attention Mechanism
Sustainability(2022)
摘要
Short-term load forecasting (STLF) is essential for urban sustainable development. It can further contribute to the stable operation of the smart grid. With the development of renewable energy, improving STLF accuracy has become a vital task. Nevertheless, most models based on the convolutional neural network (CNN) cannot effectively extract the crucial features from input data. The reason is that the fundamental requirement of adopting the convolutional neural network (CNN) is space invariance, which cannot be satisfied by the received data, limiting the forecasting performance. Thus, this paper proposes an innovative ensemble model that comprises a densely residual block (DRB), bidirectional long short-term memory (Bi-LSTM) layers based on the attention mechanism, and ensemble thinking. Specifically, the DRB is adopted to extract the potential high-dimensional features from different types of data, such as multi-scale load data, temperature data, and calendar data. The extracted features are the input of the Bi-LSTM layer. Then, the adopted attention mechanism can assign various weights to the hidden state of Bi-LSTM and focus on the crucial factors. Finally, the proposed two-stage ensemble thinking can further improve model generalization. The experimental results show that the proposed model can produce better forecasting performance compared to the existing ones, by almost 3.37–5.94%.
更多查看译文
关键词
short-term load forecasting,unshared convolutional neural network,bidirectional long short-term memory,attention mechanism,ensemble thinking
AI 理解论文
溯源树
样例
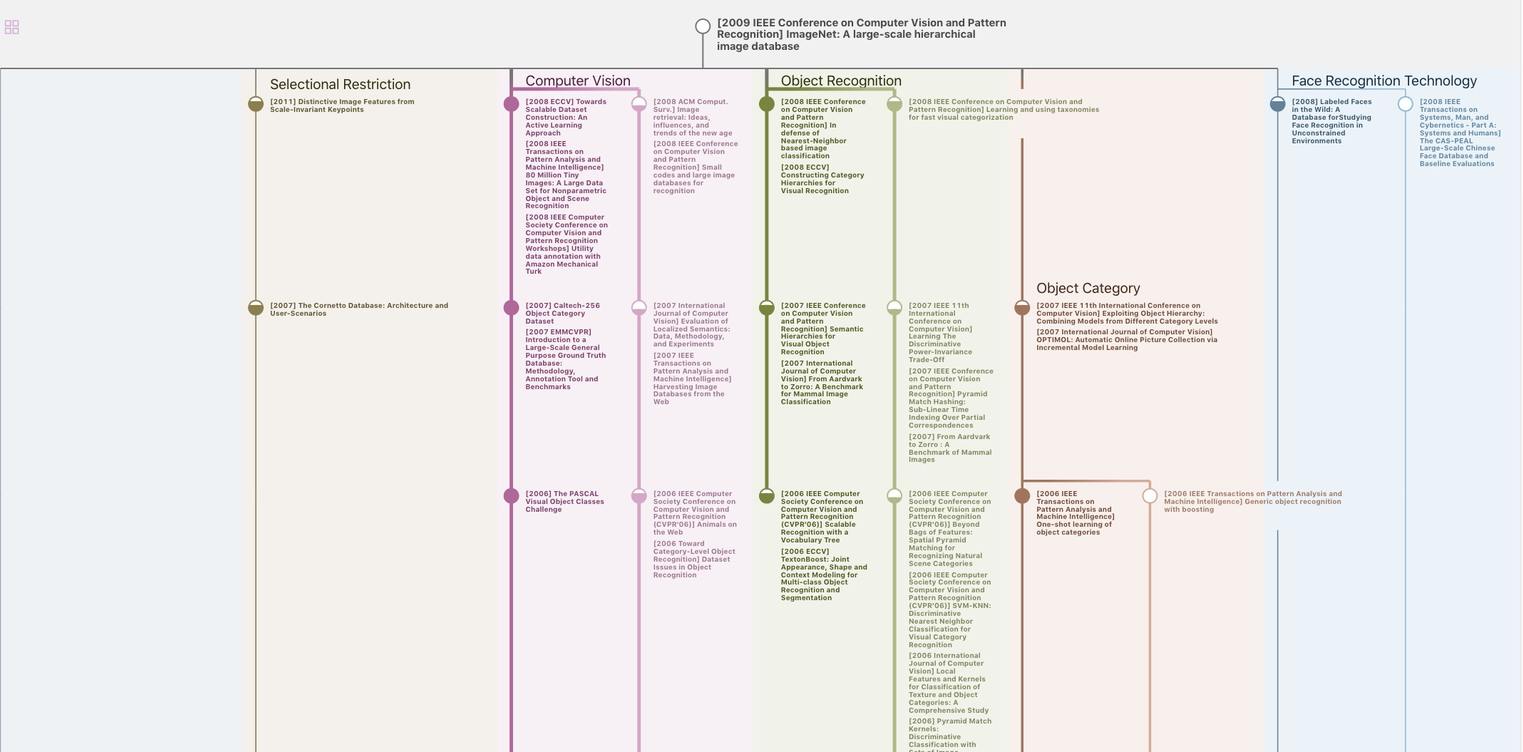
生成溯源树,研究论文发展脉络
Chat Paper
正在生成论文摘要