Machine Learning to Optimize TCP Communications over High Frequency Communications Links
IEEE access(2022)
摘要
High Frequency communication is a proven method of beyond line of sight (BLOS) communications for decades. With the advent of Wideband HF (WBHF), the ability to communicate data and more specifically TCP over HF is being researched worldwide. HF-TCP, an optimised TCP for HF links provides improved TCP communication over challenging High Frequency (HF) communication systems. It relies on the modification of Forced Retransmission Timeout (fRTO) and Mean Segment Size (MSS) to improve the reliability of communication sessions. Calculating the fRTO for a communication session that would provide the optimum result over a communication link is a complex task due to the timers that manage the session and the HF channel characteristics. In this paper, the use of Machine Learning (ML) techniques to dynamically predict fRTO and MSS to improve the fRTO calculation process for all communication instances is proposed. To achieve this, a Predictor Model is used to predict fRTO and MSS while an Optimiser model optimises the Predictor model’s output. Decision Tree Regression was proven to be the most accurate among the various ML algorithms tested with 82 percent prediction accuracy. The performance of HF-TCP with proposed predicted fRTO and MSS is compared with that of standard TCP and the performance of the Predictor and Optimiser models is also analysed. The results show 72 percent of instances have an improvement in link efficiency when using HF-TCP with predicted fRTO and MSS over standard TCP.
更多查看译文
关键词
HF communications,HF-TCP,TCP/IP,wireless communications
AI 理解论文
溯源树
样例
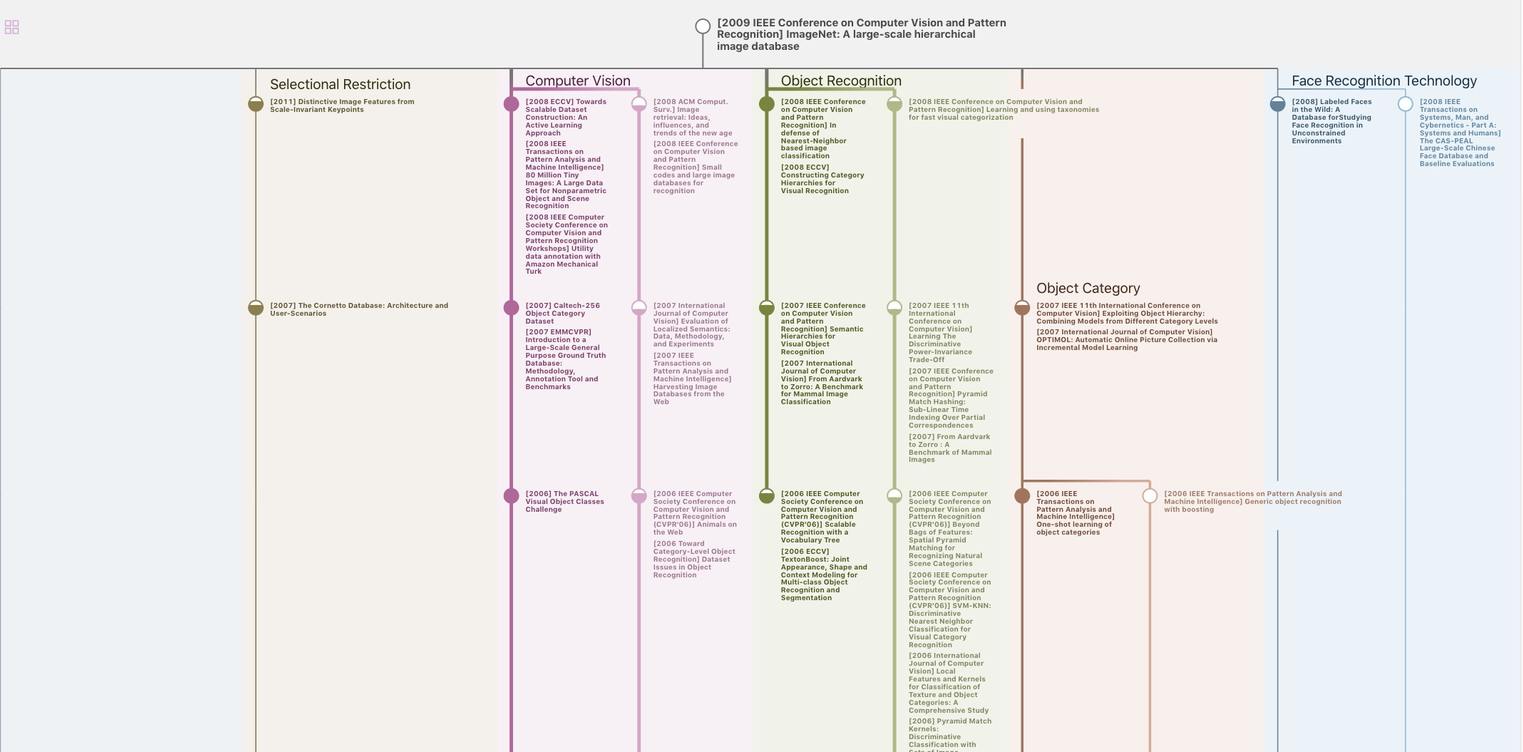
生成溯源树,研究论文发展脉络
Chat Paper
正在生成论文摘要