Identifying the Predictors of Patient-Centered Communication by Machine Learning Methods
Processes(2022)
摘要
Patient-centered communication (PCC) quality is critical to increasing the quality of patient-centered care. Based on the nationally representative data of the Health Information National Trends Survey (HINTS) 2019-2020 (N = 4593), this study combined four machine learning methods, namely, Generalized Linear Models (GLM), Random Forests (Random Forests), Deep Neural Networks (Deep Learning), and Gradient Boosting Machines (GBM), to identify important PCC predictors through variable importance metrics. Fifteen variables were identified as important predictors, involving multiple dimensions, such as individual sociodemographic characteristics, health-related factors, and individual living habits. Among them, four novel potential associated variables are included, an individual's level of verbal expression, exercise habits, etc., which significantly impacted respondents' perceived PCC quality. This study revealed the value of combining feature selection with machine learning approaches to identify broad variables that could enhance PCC prediction and clinical decision-making, influence future PCC prediction research, and improve patient-centered care. In the future, other easy-to-interpret models can be combined to conduct further research on the impact direction and mechanism of important predictors on PCC.
更多查看译文
关键词
patient-centered communication,machine learning,HINTS,predictors
AI 理解论文
溯源树
样例
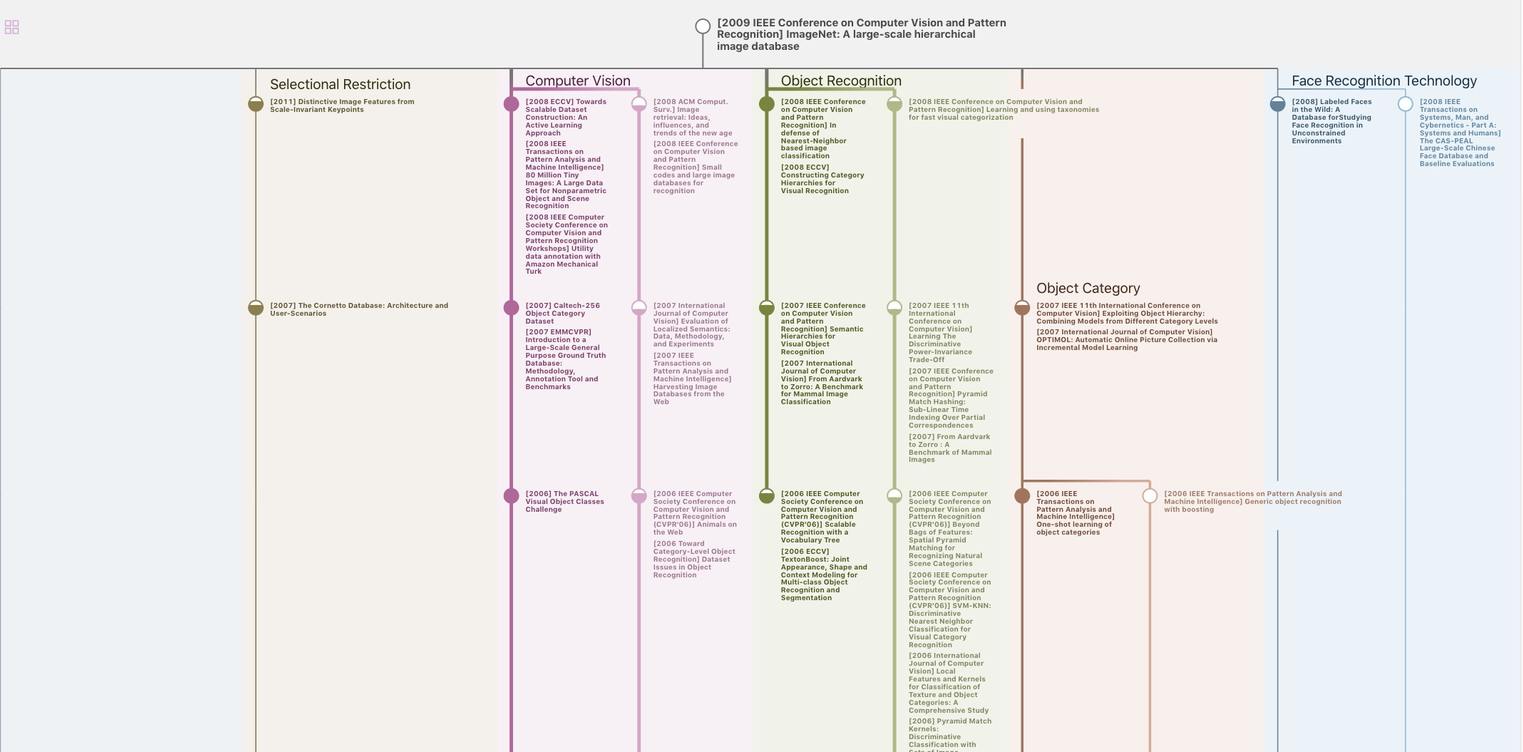
生成溯源树,研究论文发展脉络
Chat Paper
正在生成论文摘要