DA-RDD: Toward Domain Adaptive Road Damage Detection Across Different Countries
IEEE Transactions on Intelligent Transportation Systems(2023)
摘要
Recent advances on road damage detection relies on a large amount of labeled data, whilst collecting pavement image is labor-intensive and time-consuming. Unsupervised Domain Adaptation (UDA) provides a promising solution to adapt a source domain to the target domain, however, cross-domain crack detection is still an open problem. In this paper, we propose domain adaptive road damage detection termed as DA-RDD, by incorporating image-level with instance-level feature alignment for domain-invariant representation learning in an adversarial manner. Specifically, importance weighting is introduced to evaluate the intermediate samples for image-level alignment between domains, and we aggregate RoI-wise feature with multi-scale contextual information to recover the crack details for progressive domain alignment at instance level. Additionally, a large-scale road damage dataset (based on Road Damage Dataset 2020 (RDD2020)) named as RDD2021 is constructed with $100k$ synthetic labeled distress images. Extensive experimental results on damage detection across different countries demonstrate the universality and superiority of DA-RDD, and empirical studies on RDD2021 further claim its effectiveness and advancement. To our best knowledge, it is the first time to investigate domain adaptative pavement crack detection, and we expect the contributions in this work would facilitate the development of generalized road damage detection in the future.
更多查看译文
关键词
Road damage detection,domain adaptation,pavement distress dataset,adversarial learning,intelligent transportation systems
AI 理解论文
溯源树
样例
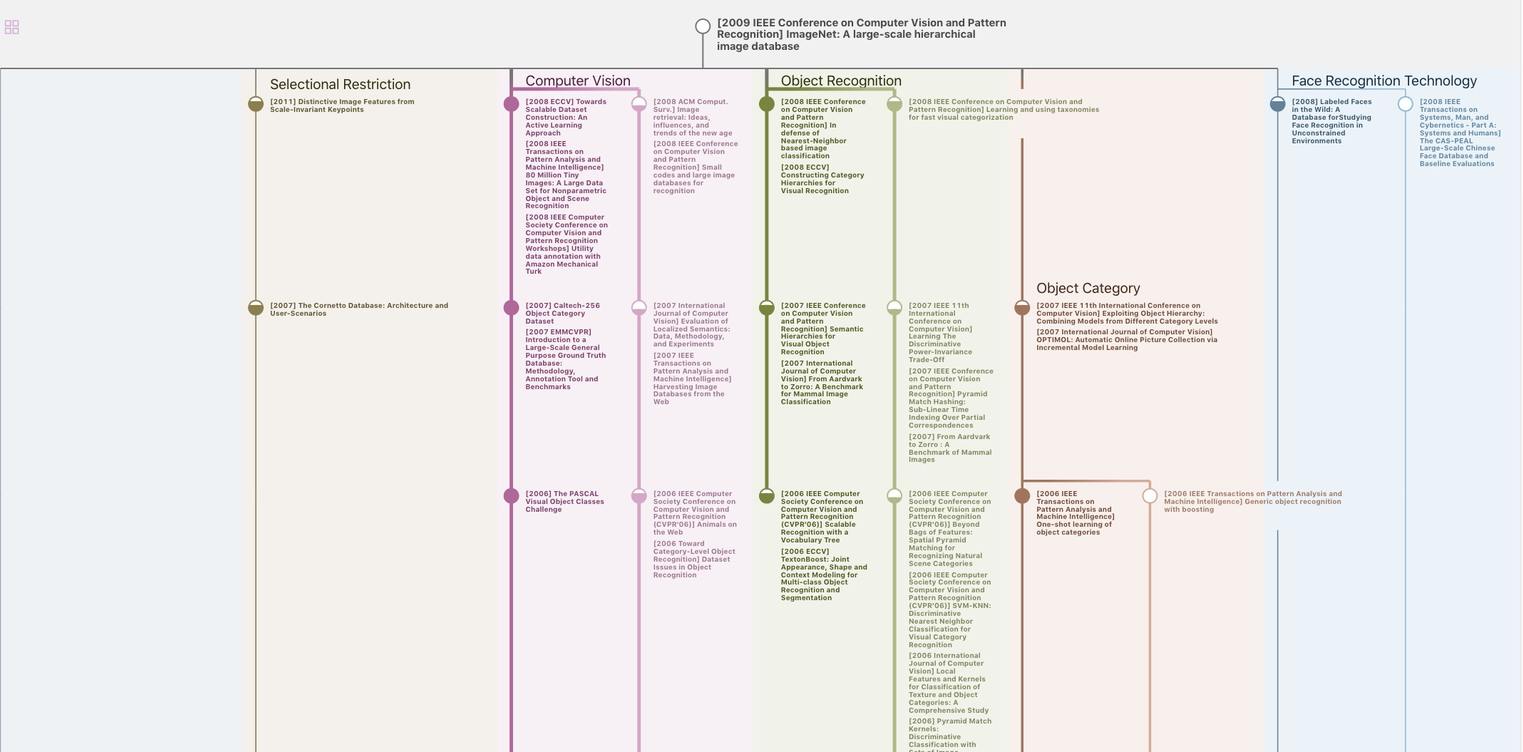
生成溯源树,研究论文发展脉络
Chat Paper
正在生成论文摘要