Investigating Differential Privacy Outcomes
ENTERPRISE INFORMATION SYSTEMS, ICEIS 2021(2022)
摘要
Personal information is an important asset nowadays. Based on customers' data, organizations are able to improve products and services, to customize solutions and to propose new ones. Privacy threats can arise from internal or external sources, having intentional or unintentional motivation. Differential Privacy (DP) is an approach that aims to utilize data while protecting the privacy of individuals. The general idea behind DP is to add noise in data in a way to keep privacy. In this paper, we provide a practical vision of DP. We introduce the D coefficient, which is an approach proposed to aid the understanding of the epsilon parameter of DP. We also conduct experiments with the four data analysis algorithms, in a way to compare the accuracy of each algorithm when applying DP. We found that the D coefficient plays an important role of setting a tangible interpretation for the amount of privacy provided by each DP mechanism. Moreover, we confirmed the consistency of the DP mathematical definition, which gives more confidence and clarity in the trade off between privacy and data utility.
更多查看译文
关键词
Privacy,Differential privacy,Classification algorithms,Data analysis
AI 理解论文
溯源树
样例
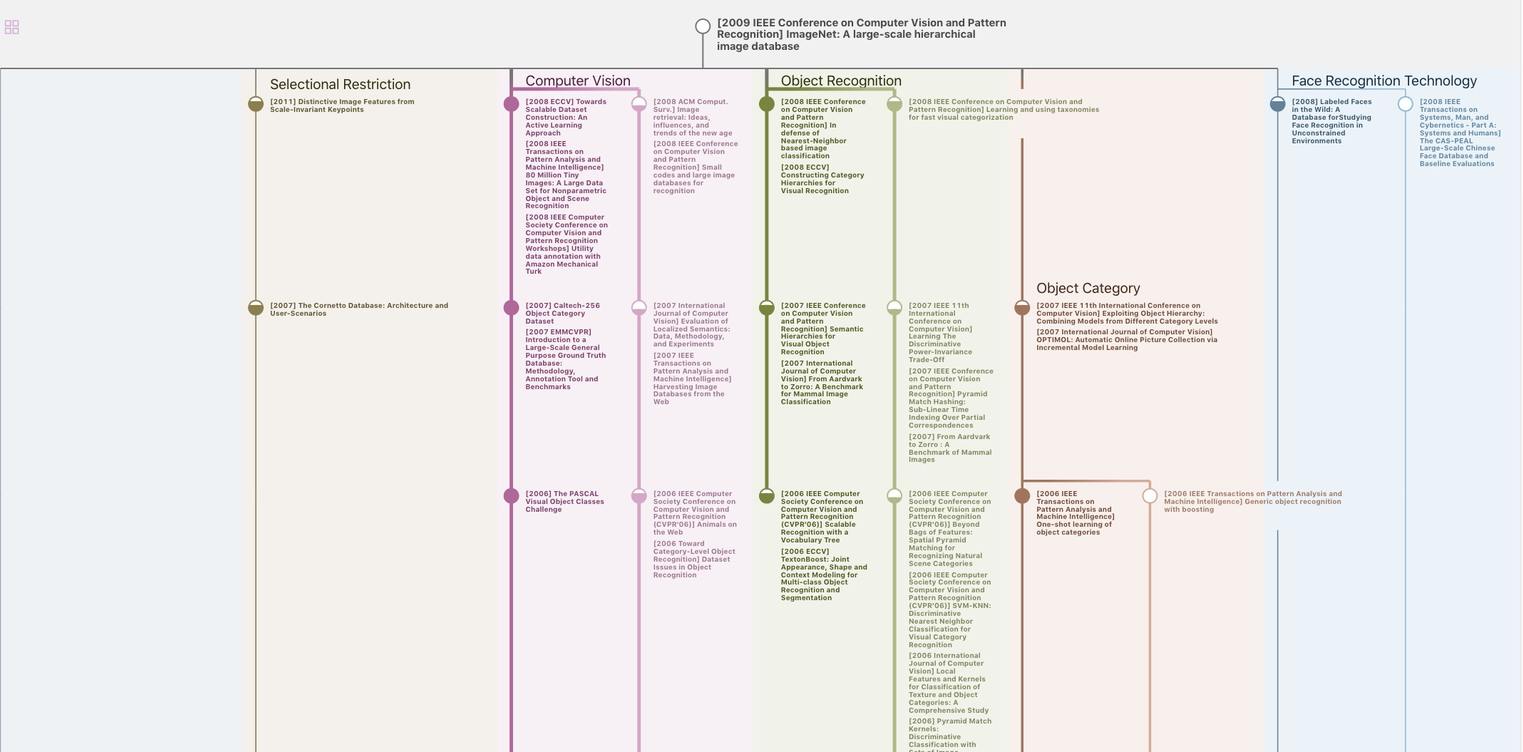
生成溯源树,研究论文发展脉络
Chat Paper
正在生成论文摘要