Explainable Multi-Class Classification Based on Integrative Feature Selection for Breast Cancer Subtyping
MATHEMATICS(2022)
摘要
Breast cancer subtype classification is a multi-class classification problem that can be handled using computational methods. Three main challenges need to be addressed. Consider first the high dimensionality of the available datasets relative to the extremely small number of instances. Second, the integration of different levels of data makes the dimensionality problem even more challenging. The third challenging issue is the ability to explain the predictions provided by a machine learning model. Recently, several deep learning models have been proposed for feature extraction and classification. However, due to the small size of the datasets, they were unable to achieve satisfactory results, particularly in multi-class classification. Aside from that, explaining the impact of features on classification has not been addressed in previous works. To cope with these problems, we propose a multi-stage feature selection (FS) framework with two data integration schemes. Using multi-omics data, four machine learning models, namely support vector machines, random forest, extra trees, and XGBoost, were investigated at each level. The SHAP framework was used to explain how specific features influenced classification. Experimental results demonstrated that ensemble models with early integration and two stage feature selection improved results compared to baseline experiments and to state-of-the art methods. Furthermore, more explanations regarding the implications of the main relevant features in the predictions are provided, which could serve as a baseline for future biological investigations.
更多查看译文
关键词
feature selection, multi-class classification, explainable AI, breast cancer sub-typing, multi-omics data, SHAP plots
AI 理解论文
溯源树
样例
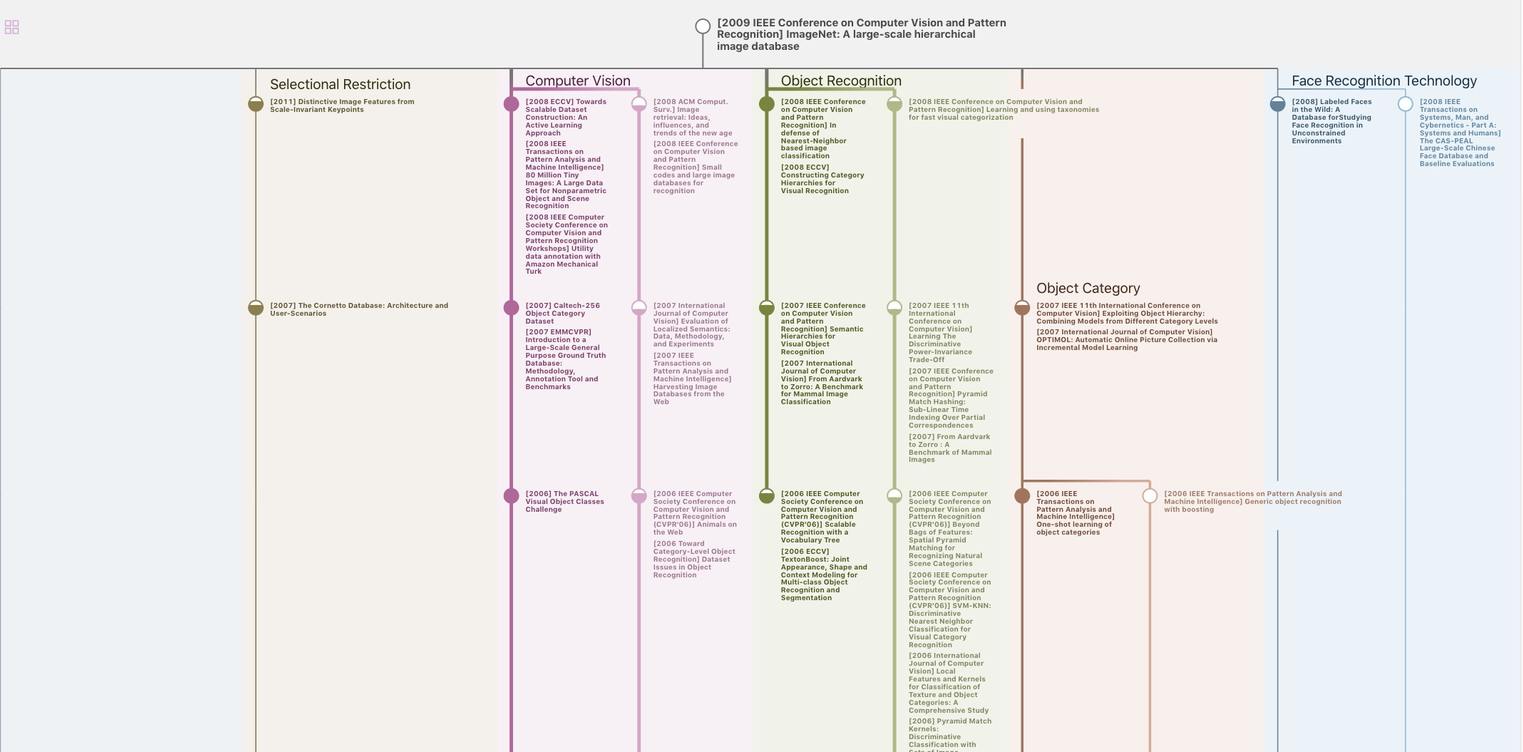
生成溯源树,研究论文发展脉络
Chat Paper
正在生成论文摘要