Multi-granularity stock prediction with sequential three-way decisions
Information Sciences(2023)
摘要
The current single granularity-based static prediction approaches face difficulties in capturing the dynamic trend of stock markets by the fusion of historical prices and streaming textual data (e.g., financial news, financial reports, and tweets). Besides, the traditional stock predictions by two-way decisions do not consider the continual investigation of the uncertain samples in boundary regions, thus increasing financial risk and loss. To address these issues, this paper introduces a novel sequential three-way stock prediction model under the multilevel granular structure of hybrid data. We construct three kinds of multi-granularity methods to combine price and textual data, i.e., high-frequency data with features extracted by Sentiment Analysis (senthigh), high-frequency data with features extracted by BERT (berthigh), and low-frequency data with features extracted by BERT (bertlow). Subsequently, we employ sequential three-way decisions to continually predict the future trend of each test sample at adequate granularity. In addition, an autonomous attention mechanism is embedded inside the CNN and RNN to handle large embeddings and long time series dynamically. Finally, the comparative experiments are conducted on 5-year stock data to demonstrate that the proposed models outperform the non-sequential two-way predictions.
更多查看译文
关键词
Sequential three-way decisions,Multi-granularity,Stock prediction,Data fusion
AI 理解论文
溯源树
样例
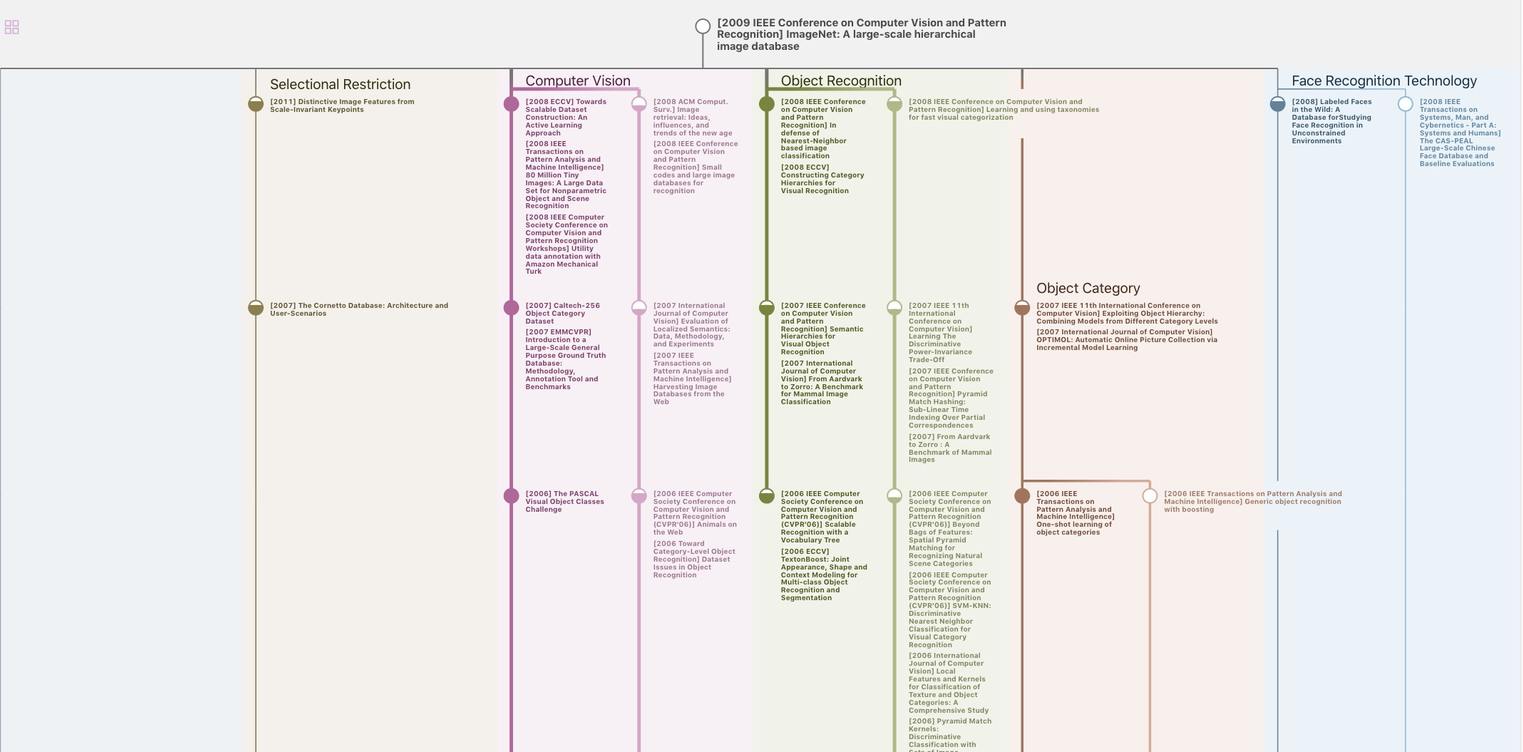
生成溯源树,研究论文发展脉络
Chat Paper
正在生成论文摘要