Multiple metric learning via local metric fusion
Information Sciences(2023)
摘要
Adaptive distance metric learning based on the characteristics of data can significantly improve the learner’s performance. Due to the limitations of single metric learning for heterogeneous data, multiple local metric learning has become an essential representative tool to describe local properties of data. Most existing multiple metric learning algorithms need to perform metric learning on a pre-obtained instance division. However, the number of clusters in the pre-obtained division affects the effectiveness of metric learning. To tackle this problem, we propose a Multiple Metric Learning via Local Metric Fusion (MML-LMF) framework, which unifies local metric learning and fusion of similar local metrics into one metric and adaptively determines the number of local metrics. As an application of the MML-LMF framework to pairwise constraints, we devise a MML-LMF algorithm by constructing a concrete optimization model and acquiring a closed-form solution to the model. The experimental results on several benchmarks, person re-identification, and face verification datasets show that the performance of the proposed algorithm is superior to that of the existing state-of-the-art global and multiple metric learning algorithms.
更多查看译文
关键词
Metric learning,Multiple metric learning,Metric fusion
AI 理解论文
溯源树
样例
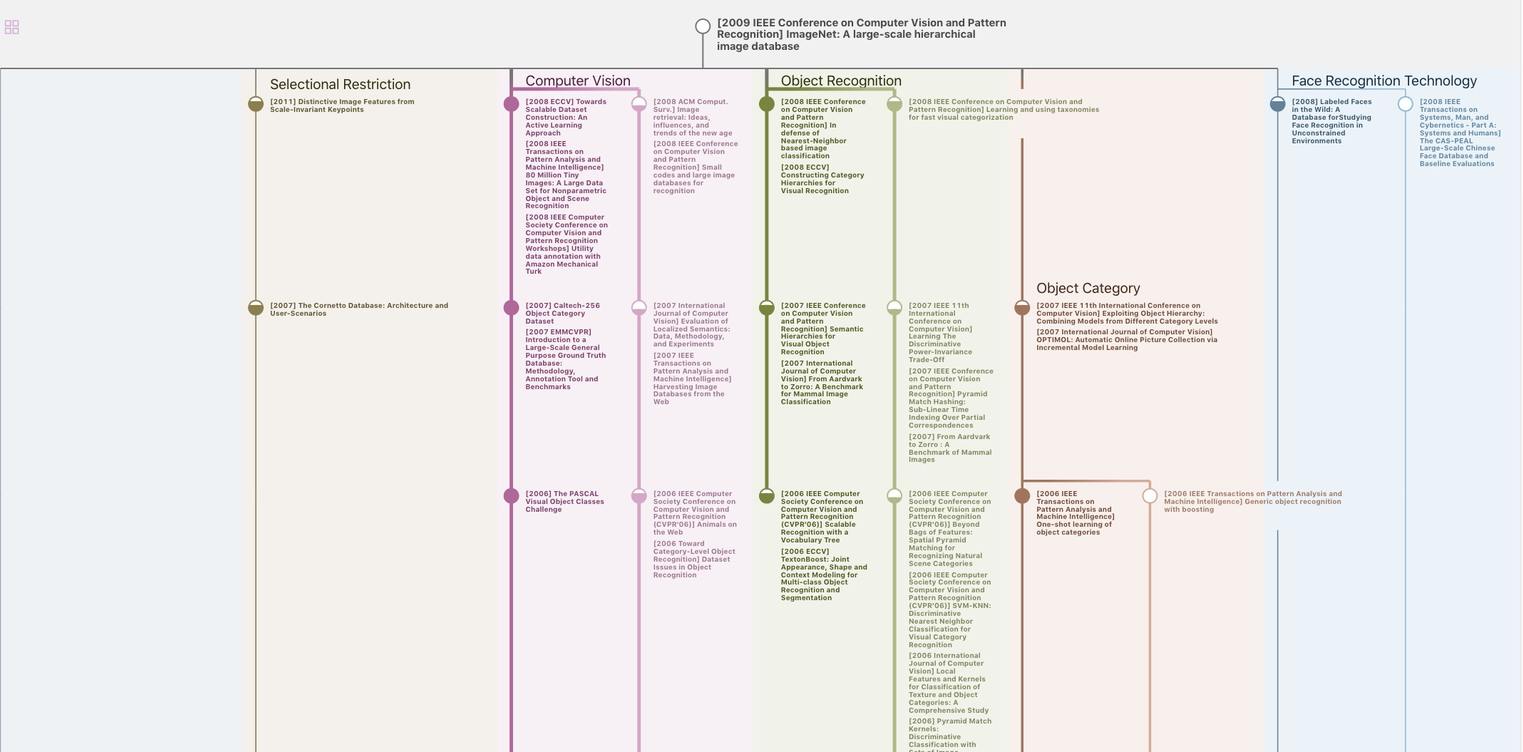
生成溯源树,研究论文发展脉络
Chat Paper
正在生成论文摘要