Validation of conditional and superresolution generative adversarial networks for imputation of missing brain mri sequences
NEURO-ONCOLOGY(2022)
摘要
Abstract Missing MRI sequences are common in radiology due to differences in protocols, time constraints, and image artifacts. Missing sequences are a nuisance for subsequent algorithms, such as automated segmentation algorithms requiring multiple MRI sequences (e.g., HD-GLIO, which requires T2, T1, post-contrast T1 (T1c), and FLAIR). Accurate tumor segmentation is a fundamental step in extracting radiomics analysis to use, for example, to predict the IDH status of glioma or their overall survival. We validated a conditional (pix2pix) and super-resolution GAN (SOUP-GAN) for imputing MRI sequences on 285 adult glioma with complete MRI sequences available. We simulated missing T1 sequences and subsequently imputed them from T1c using pix2pix and from sagittal T1 using SOUP-GAN. Using HD-GLIO, we obtained the true segmentations from the complete MRI sequences. HD-GLIO was also used after replacing the original T1 sequence with the one imputed with pix2pix and SOUP-GAN. Thus, there were three sets of segmentations for each subject. We compared T2 and T1c radiomics features extracted with Pyradiomics using segmentations obtained with complete data with features extracted from segmentation obtained with the imputed T1 sequences using intraclass correlation coefficient (ICC) and Bland-Altman (BA) analysis. Median (interquartile range) ICC for shape features extracted from T2 and T1c were 0.82(0.76-0.92) using pix2pix and 0.89(0.86-0.94) using SOUP-GAN. Median ICC for first-order-statistics features were 0.90(0.83-0.93) for T2 and 0.90(0.88-0.92) for T1c using pix2pix, and 0.61(0.22-0.82) for T2 and 0.42(0.34-0.57) for T1c using SOUP-GAN. Median ICC for texture features were 0.86(0.82-0.87) for T2 and 0.87(0.79-0.90) for T1c using pix2pix, and 0.69(0.47-0.81) for T2 and 0.76(0.65-0.83) for T1c using SOUP-GAN. We show that T2 and T1c shape features (e.g., volumetric analysis) can be adequately extracted from segmentations obtained with an algorithm requiring multiple MRI sequences using T1 sequences imputed with GANs; however, the effect of imputation differed for first-order-statistics and texture features.
更多查看译文
关键词
missing brain mri sequences,imputation,super-resolution
AI 理解论文
溯源树
样例
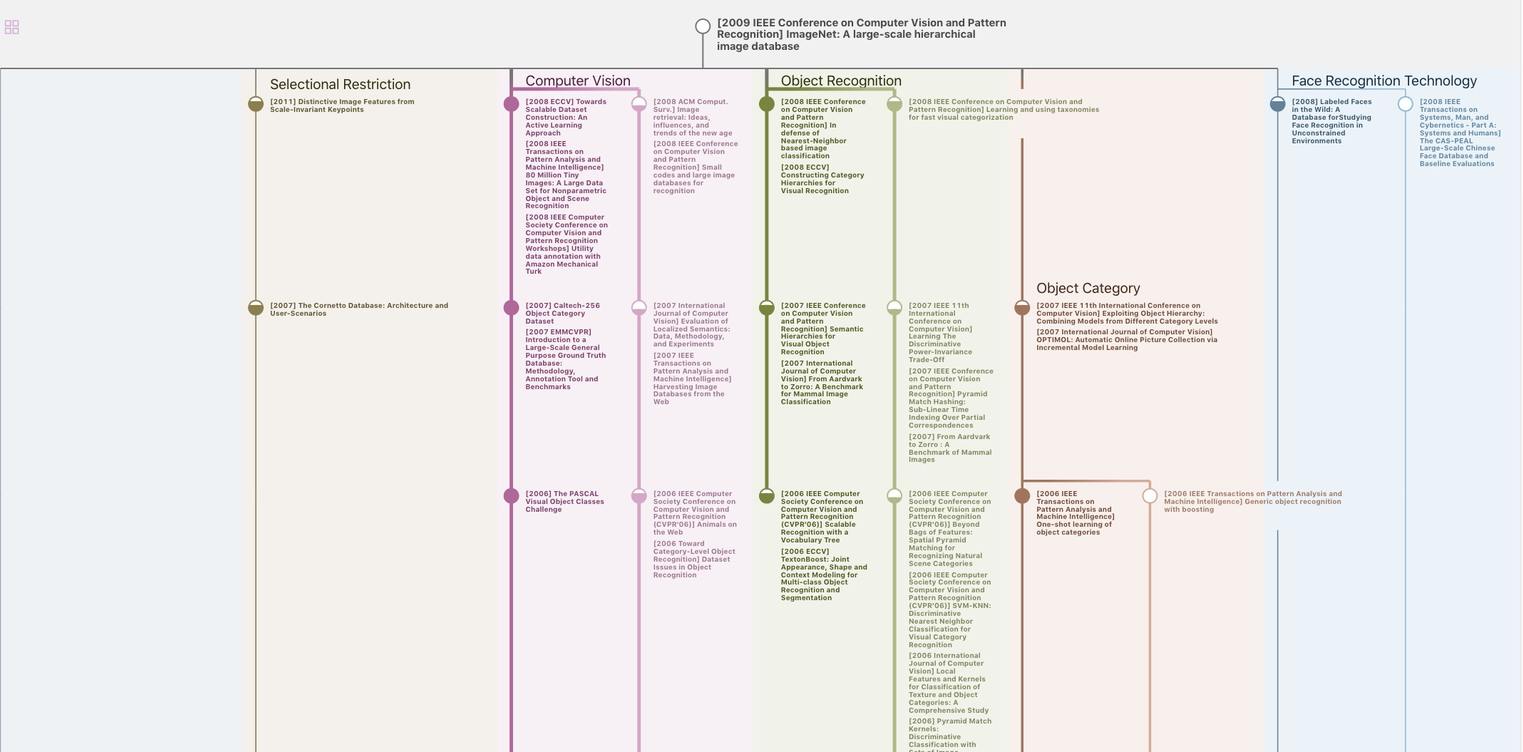
生成溯源树,研究论文发展脉络
Chat Paper
正在生成论文摘要