Pacs-integrated auto-segmentation workflow for brain metastases using nnu-net
NEURO-ONCOLOGY(2022)
摘要
Abstract PURPOSE Monitoring metastatic disease to the brain is laborious and time-consuming, especially in the setting of multiple metastases and when performed manually. Response assessment in brain metastases based on maximal unidimensional diameter as per the RANO-BM guideline is commonly performed1, however, accurate volumetric lesion estimates can be crucial for clinical decision-making2 and enhance outcome prediction3. We propose a deep learning (DL)-based auto-segmentation approach with the potential for improvement of time-efficiency, reproducibility and robustness against inter-rater variability. Materials and METHODS We retrospectively retrieved 259 patients with a total number of 916 lesions from our institutional database from 2014 - 2021. Patients with prior history of local radiation therapy or surgery were excluded. Manually generated trainee segmentations were revised and adjusted by a board-certified radiologist and served as ground truth for evaluation of segmentation accuracy. Model performance was tested via dice-similarity-coefficient (DSC). Volumetric measurements were then obtained within our PACS-integrated workflow on Visage 7 (Visage Imaging, Inc., San Diego, CA) at the click of one button. RESULTS For model training and evaluation, a train-test split of 90:10 on patient-level was performed (n= 234:25 (Patients), n= 861:55 (Lesions). A DL-algorithm (nnUNet) was incrementally trained on 10 batches of 23 patients. The DSC of the U-Net gradually increased throughout the training process and heuristically reached a plateau of 0.85. The sensitivity of the algorithm was 83% with detection of 46 out of 55 lesions in the testing dataset. The lesions that were not detected by the algorithm were below 5 mm in size. The false positive rate was 8% (n=4/50). CONCLUSION Our study demonstrates the feasibility of PACS-based integration of automatized segmentation workflows of brain metastases. An incremental-training approach is recommended to adapt DL algorithms to specific hospital settings.
更多查看译文
关键词
brain metastases,pacs-integrated,auto-segmentation,nnu-net
AI 理解论文
溯源树
样例
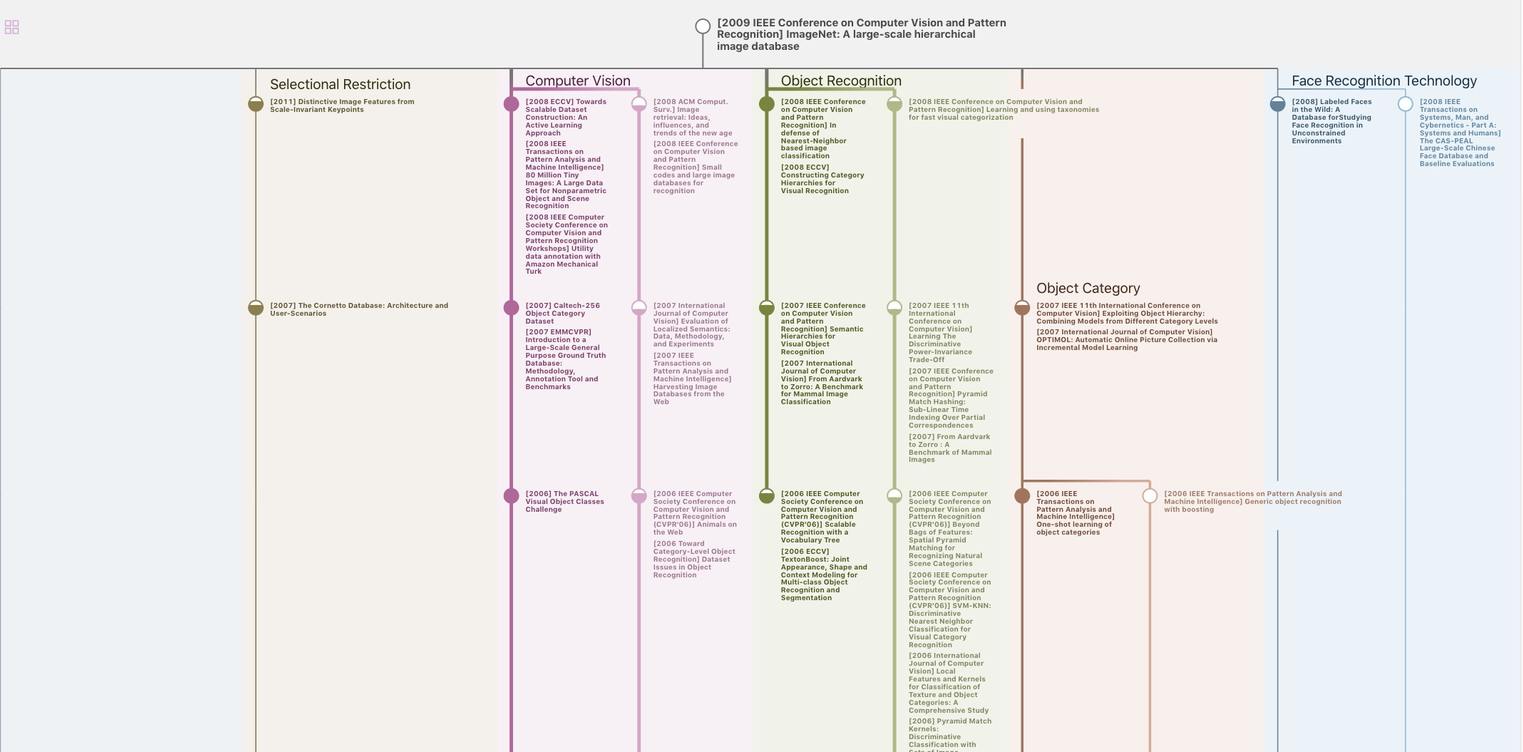
生成溯源树,研究论文发展脉络
Chat Paper
正在生成论文摘要