Penalized additive neural network regression
INTELLIGENT DATA ANALYSIS(2022)
摘要
In this study, we develop a penalized additive regression estimation method based on a neural network architecture. An additive neural network model is constructed by using a linear combination of univariate neural networks, or equivalently functional components. We use a B-spline activation function, which is useful to capture local features of data, for nodes that constitute the model. A penalty function is adopted to induce sparsity in functional components and nodes on each component simultaneously. This enables us to obtain a sparse representation, which in turn improves accountability of the model. To implement the proposed estimation method, we devise an efficient iterative algorithm based on a coordinate-wise updating process. An initialization scheme specialized for the B-spline activation function is proposed. The initialization approach enables the proposed method to achieve better performance compared with random initialization scheme. Numerical studies show that the fitted functional components of our estimator adapt to local and sparse structures based on a given dataset.
更多查看译文
关键词
Additive regression model, neural network architecture, penalization, sparsity
AI 理解论文
溯源树
样例
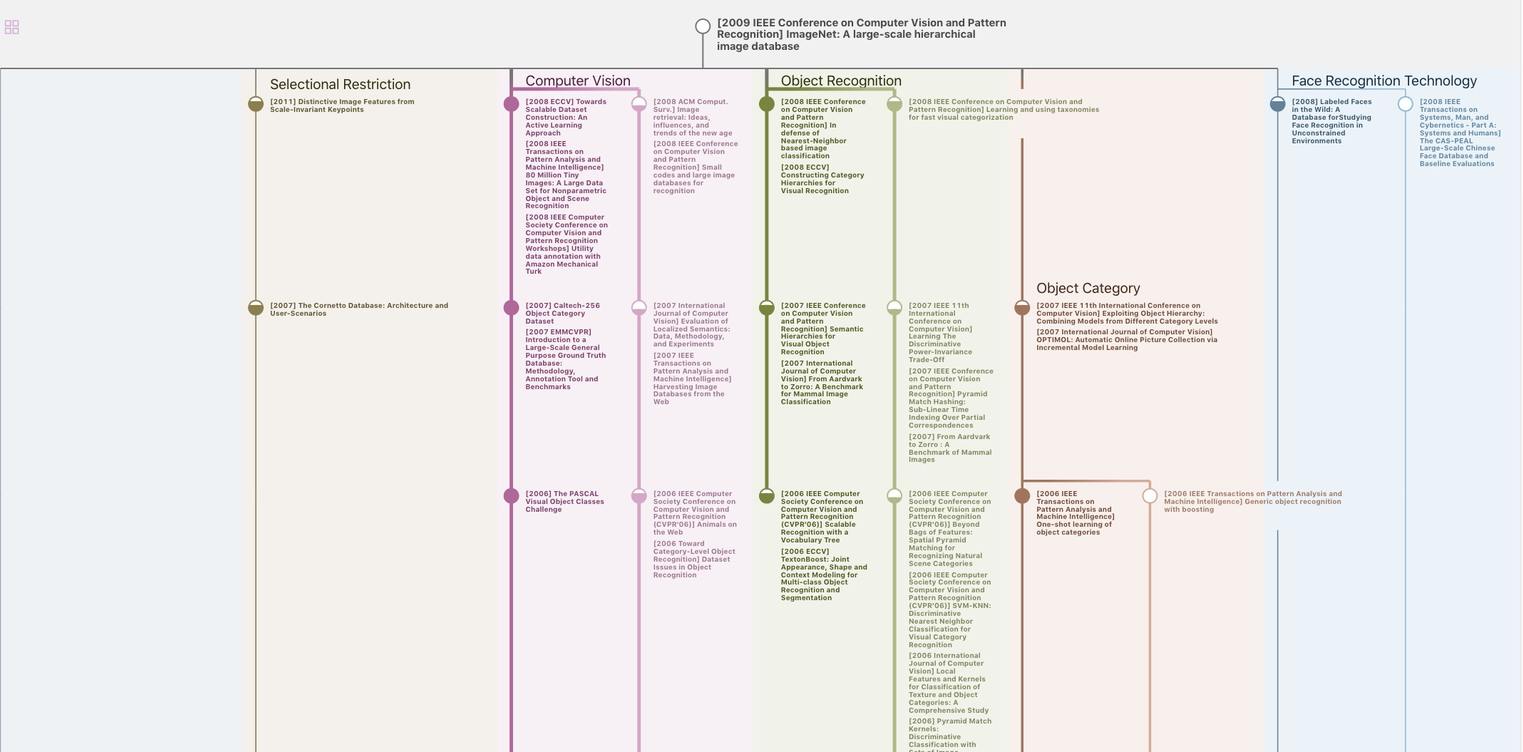
生成溯源树,研究论文发展脉络
Chat Paper
正在生成论文摘要