Finite-field coupling via learning the charge response kernel
ELECTRONIC STRUCTURE
摘要
Response of the electronic density at the electrode-electrolyte interface to the external field (potential) is fundamental in electrochemistry. In density-functional theory, this is captured by the so-called charge response kernel (CRK). Projecting the CRK to its atom-condensed form is an essential step for obtaining the response charge of atoms. In this work, the atom-condensed CRK is learnt from the molecular polarizability using machine learning (ML) models and subsequently used for the response-charge prediction under an external field (potential). As the machine-learnt CRK shows a physical scaling of polarizability over the molecular size and does not (necessarily) require the matrix-inversion operation in practice, this opens up a viable and efficient route for introducing finite-field coupling in the atomistic simulation of electrochemical systems powered by ML models.
更多查看译文
关键词
conceptual DFT, electronic polarization, machine learning, electrode-electrolyte interface, finite-field method, atomic charge
AI 理解论文
溯源树
样例
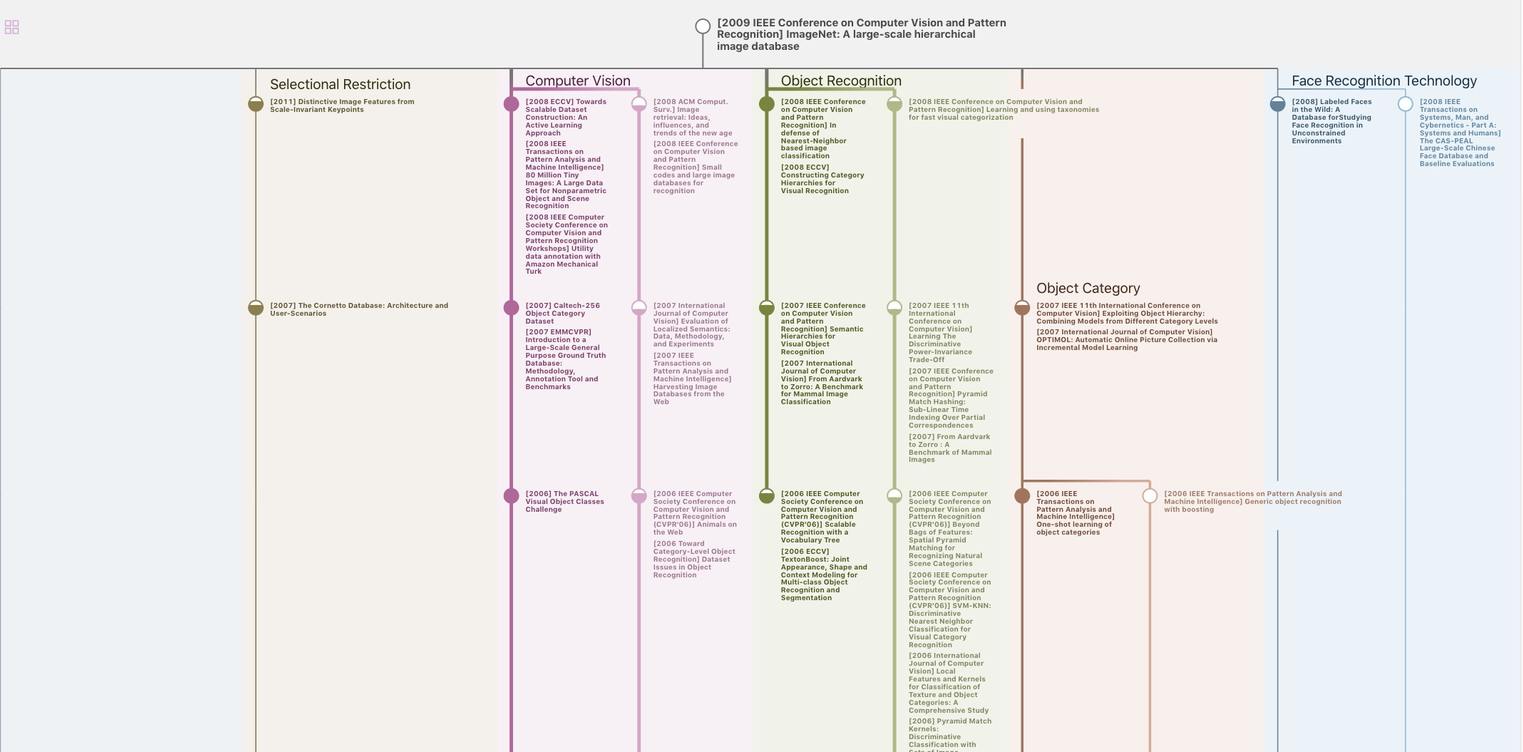
生成溯源树,研究论文发展脉络
Chat Paper
正在生成论文摘要