Neural Entity Alignment with Cross-Modal Supervision
Information processing & management(2023)
摘要
The majority of currently available entity alignment (EA) solutions primarily rely on structural information to align entities, which is biased and disregards additional multi-source information. To compensate for inadequate structural details, this article suggests the SKEA framework, which is a simple but flexible framework for Entity Alignment with cross-modal supervision of Supporting Knowledge. We employ a relational aggregate network to specifically utilize the details about the entity and its neighbors. To overcome the limitations of relational features, two multi-modal encode modules are being used to extract visual and textural information. A new set of potential aligned entity pairs are generated by SKEA in each iteration using the knowledge of two reference modalities, which can enhance the model’s supervision. It is important to note that the supporting information used in our framework does not participate in the network’s backpropagation, which considerably improves efficiency and differs dramatically from earlier work. In comparison to existing baselines, experiments demonstrate that our proposed framework can incorporate multi-aspect information efficiently and enable supervisory signals from other modalities to transmit to entities. The maximum performance improvement of 5.24% indicates our suggested framework’s superiority, especially for sparse KGs.
更多查看译文
关键词
Knowledge graph alignment,Supporting knowledge,Relational attention network,Semi-supervised learning
AI 理解论文
溯源树
样例
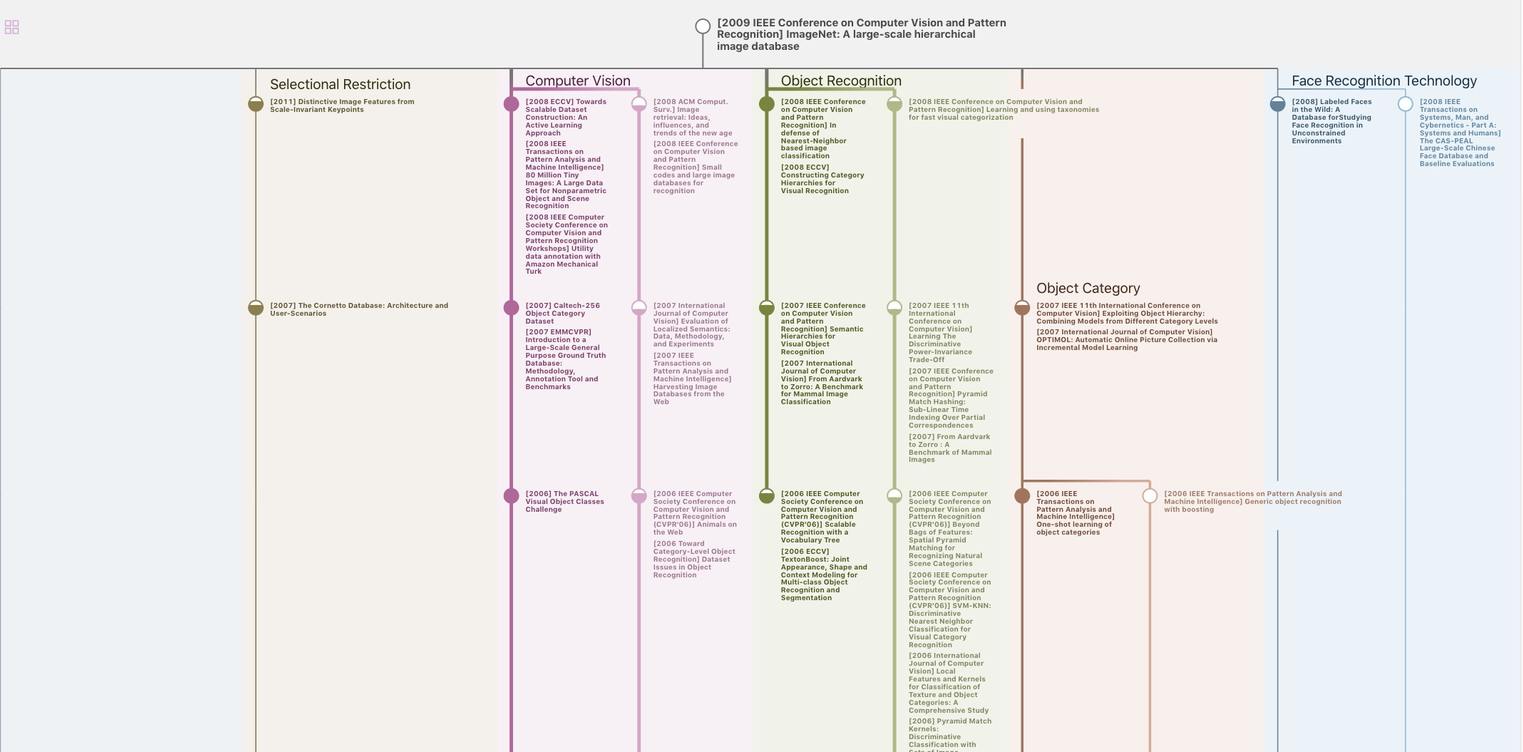
生成溯源树,研究论文发展脉络
Chat Paper
正在生成论文摘要