Ensemble Learning-Enabled Security Anomaly Identification for IoT Cyber-Physical Power Systems
ELECTRONICS(2022)
摘要
The public network access to smart grids has a great impact on the system's safe operation. With the rapid increase in Internet of Things (IoT) applications, cyber-attacks caused by multiple sources and flexible loads continue to rise, which results in equipment maloperation and security hazard problems. In this paper, a novel ensemble learning algorithm (ELA)-enabled security anomaly identification technique is proposed. Firstly, the propagation process of typical cyber-attacks was analyzed to illustrate the impact on message transmission and power operation. Then, a feature matching identification method was designed according to the sequence sets under different situations. The classification rate of these abnormal attack behaviors was acquired thereafter, which could aid in the listing of the ranking of the consequences of abnormal attack behaviors. Moreover, the weights of training samples can be further updated according to the performance of weak learning error rates. Through a joint hardware platform, numerical results show that the proposed technique is effective and performs well in terms of situation anomaly identification.
更多查看译文
关键词
cyber-physical power system (CPPS), Internet of Things (IoT) scenario, ensemble learning algorithm, analytic hierarchy process, abnormal attack behaviors
AI 理解论文
溯源树
样例
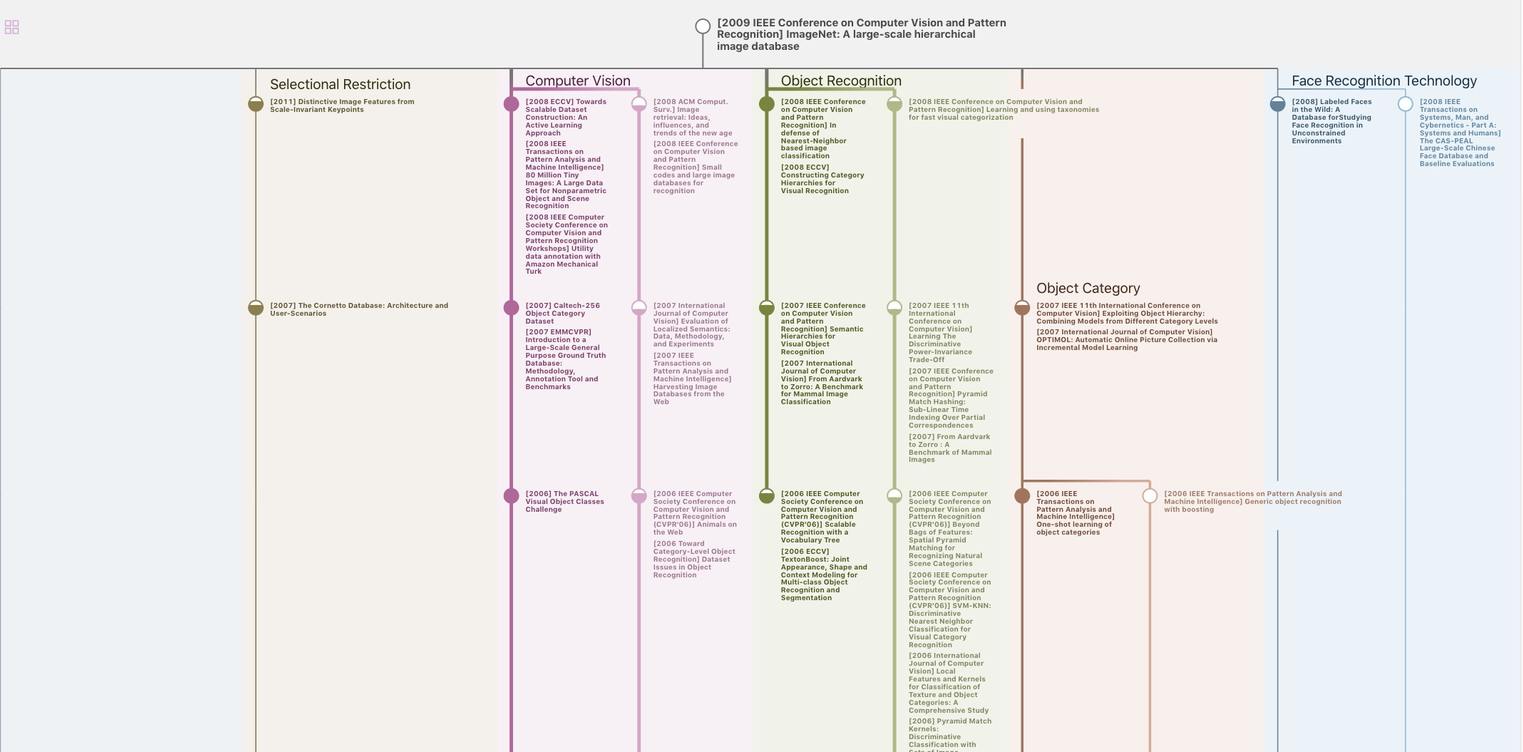
生成溯源树,研究论文发展脉络
Chat Paper
正在生成论文摘要