A VGGNet-Based Method for Refined Bathymetry from Satellite Altimetry to Reduce Errors
REMOTE SENSING(2022)
摘要
Only approximately 20% of the global seafloor topography has been finely modeled. The rest either lacks data or its data are not accurate enough to meet practical requirements. On the one hand, the satellite altimeter has the advantages of large-scale and real-time observation. Therefore, it is widely used to measure bathymetry, the core of seafloor topography. However, there is often room to improve its precision. Multibeam sonar bathymetry is more precise but generally limited to a smaller coverage, so it is in a complementary relationship with the satellite-derived bathymetry. To combine the advantages of satellite altimetry-derived and multibeam sonar-derived bathymetry, we apply deep learning to perform multibeam sonar-based bathymetry correction for satellite altimetry bathymetry data. Specifically, we modify a pretrained VGGNet neural network model to train on three sets of bathymetry data from the West Pacific, Southern Ocean, and East Pacific. Experiments show that the correlation of bathymetry data before and after correction can reach a high level, with the performance of R-2 being as high as 0.81, and the normalized root-mean-square deviation (NRMSE) improved by over 19% compared with previous research. We then explore the relationship between R-2 and water depth and conclude that it varies at different depths. Thus, the terrain specificity is a factor that affects the precision of the correction. Finally, we apply the difference in water depth before and after the correction for evaluation and find that our method can improve by more than 17% compared with previous research. The results show that the VGGNet model can perform better correction to the bathymetry data. Hence, we provide a novel method for accurate modeling of the seafloor topography.
更多查看译文
关键词
seafloor topography inversion, bathymetry, multibeam sonar, satellite altimetry, VGGNet
AI 理解论文
溯源树
样例
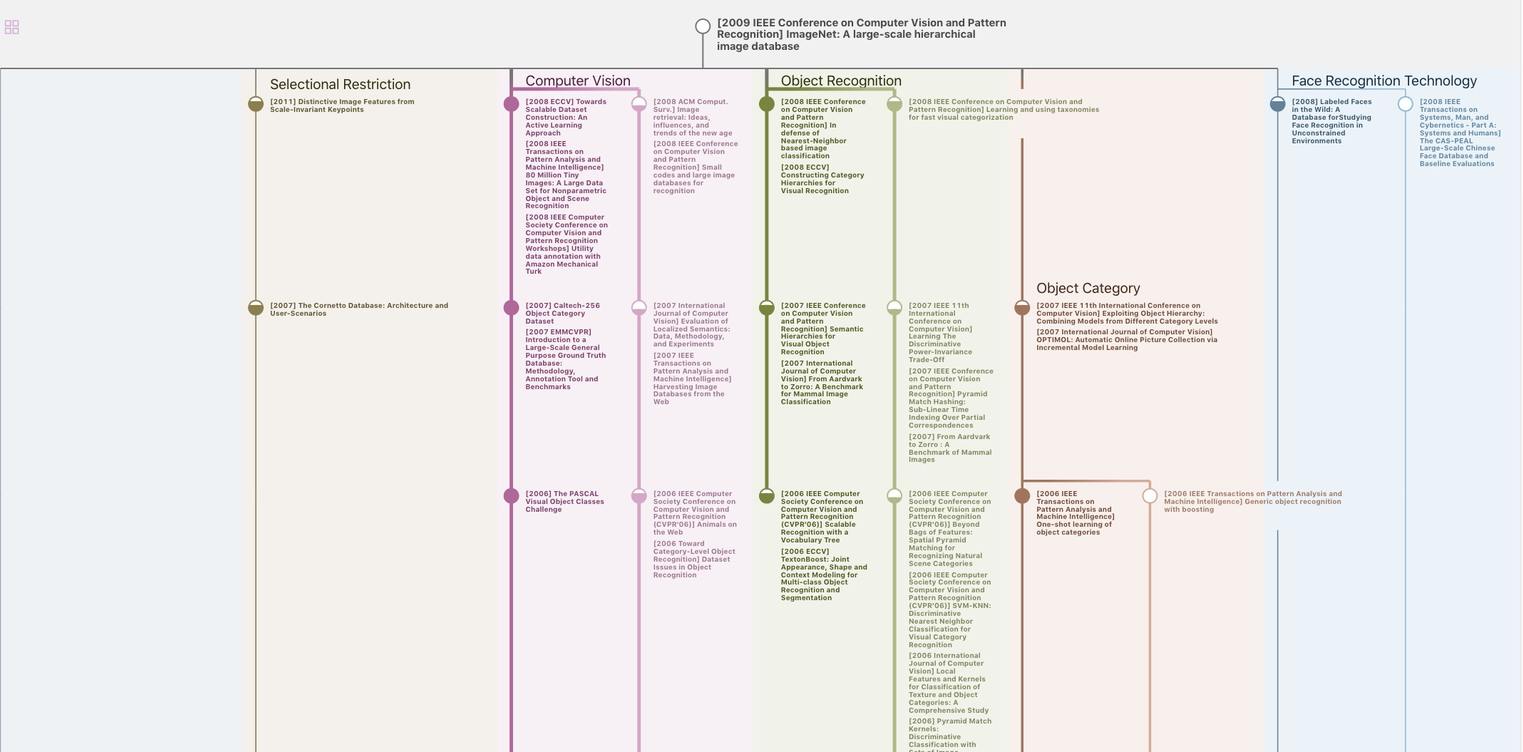
生成溯源树,研究论文发展脉络
Chat Paper
正在生成论文摘要