Multi-Feature Extension via Semi-Autoencoder for Personalized Recommendation
APPLIED SCIENCES-BASEL(2022)
摘要
Over the past few years, personalized recommendation systems aim to address the problem of information overload to help users achieve useful information and make quick decisions. Recently, due to the benefits of effective representation learning and no labeled data requirements, autoencoder-based models have commonly been used in recommendation systems. Nonetheless, auxiliary information that can effectively enlarge the feature space is always scarce. Moreover, most existing methods ignore the hidden relations between extended features, which significantly affects the recommendation accuracy. To handle these problems, we propose a Multi-Feature extension method via a Semi-AutoEncoder for personalized recommendation (MFSAE). First, we extract auxiliary information from DBpedia as feature extensions of items. Second, we leverage the LSI model to learn hidden relations on top of item features and embed them into low-dimensional feature vectors. Finally, the resulting feature vectors, combined with the original rating matrix and side information, are fed into a semi-autoencoder for recommendation prediction. We ran comprehensive experiments on the MovieLens datasets. The results demonstrate the effectiveness of MFSAE compared to state-of-the-art methods.
更多查看译文
关键词
multi-feature extension, autoencoder, personalized recommendation, collaborative filtering, knowledge graph
AI 理解论文
溯源树
样例
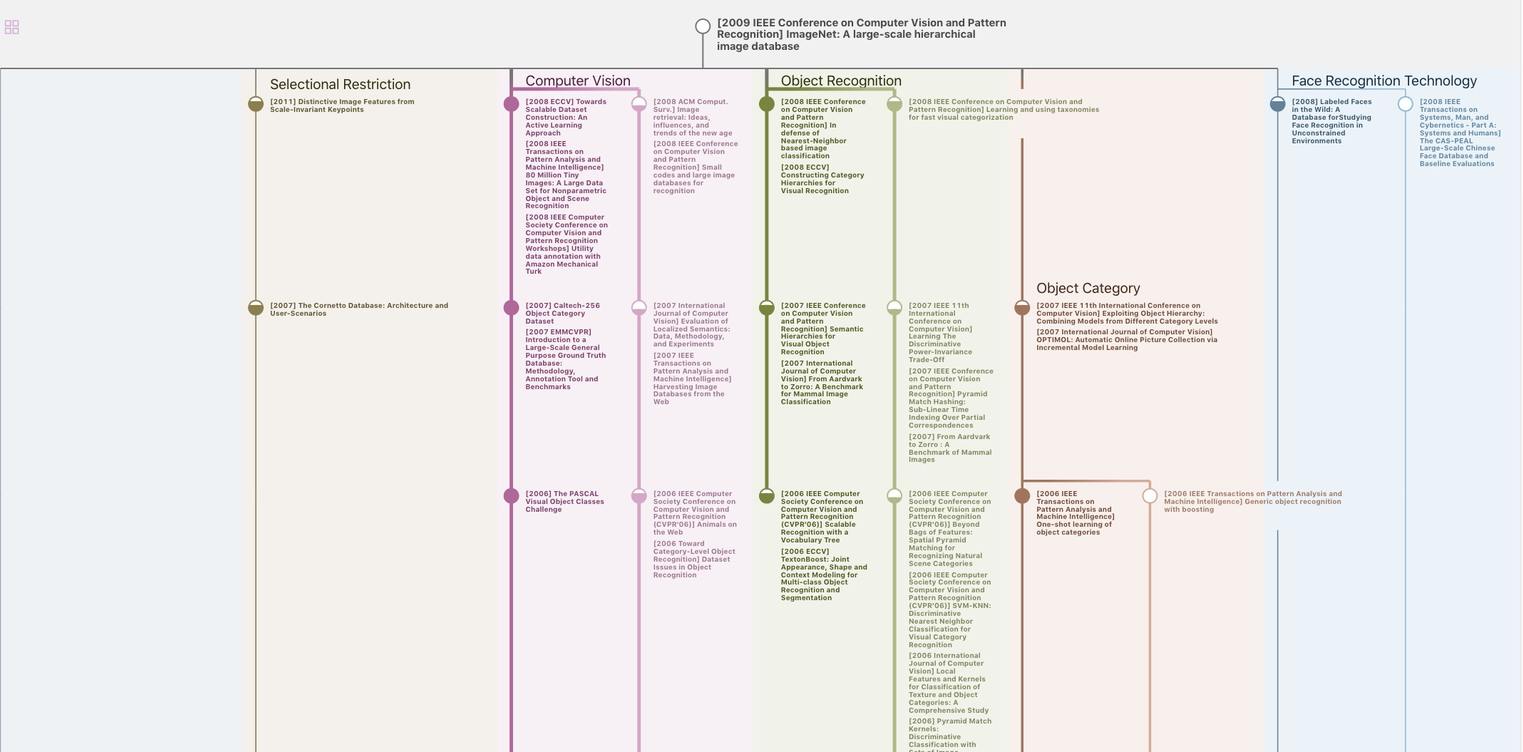
生成溯源树,研究论文发展脉络
Chat Paper
正在生成论文摘要