Lane-Level Heterogeneous Traffic Flow Prediction: A Spatiotemporal Attention-Based Encoder-Decoder Model
IEEE INTELLIGENT TRANSPORTATION SYSTEMS MAGAZINE(2023)
摘要
Urban road traffic flow prediction is the key basis for the development of intelligent transportation systems. Lane-level heterogeneous traffic flow prediction will become a new and important challenge in the future development of intelligent transportation. In this study, a spatiotemporal attention-based encoder-decoder model is proposed to solve the lane-level heterogeneous traffic flow prediction problem. Specifically, the traffic flows of different locations and different types of vehicles are all regarded as traffic flows running in independent spaces. A spatial attention layer is added to the encoder to capture the spatial characteristics of traffic flows, and a temporal attention layer is added to the decoder to analyze the temporal correlation of each time interval. Finally, we validate the model by using real traffic monitoring data in the Shunyi District, Beijing, China, and prove the superior performance of the model. Meanwhile, the output of attention weights can deepen the further interpretation of the spatiotemporal correlation of heterogeneous traffic flows.
更多查看译文
关键词
Predictive models, Deep learning, Correlation, Transportation, Roads, Spatiotemporal phenomena, Data models
AI 理解论文
溯源树
样例
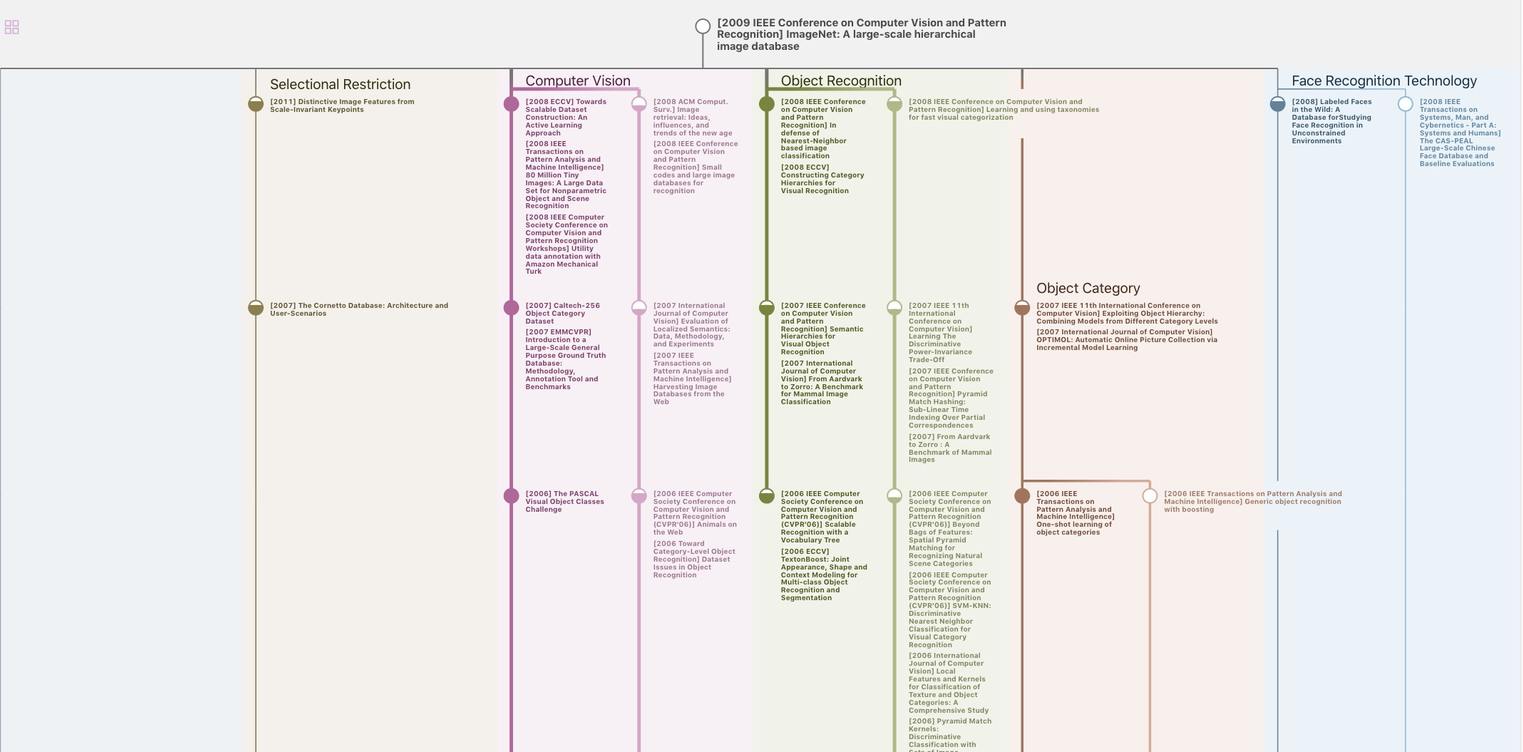
生成溯源树,研究论文发展脉络
Chat Paper
正在生成论文摘要