Adapting multilingual speech representation model for a new, underresourced language through multilingual fine-tuning and continued pretraining
Information Processing & Management(2023)
摘要
In recent years, neural models learned through self-supervised pretraining on large scale multilingual text or speech data have exhibited promising results for underresourced languages, especially when a relatively large amount of data from related language(s) is available. While the technology has a potential for facilitating tasks carried out in language documentation projects, such as speech transcription, pretraining a multilingual model from scratch for every new language would be highly impractical. We investigate the possibility for adapting an existing multilingual wav2vec 2.0 model for a new language, focusing on actual fieldwork data from a critically endangered tongue: Ainu. Specifically, we (i) examine the feasibility of leveraging data from similar languages also in fine-tuning; (ii) verify whether the model's performance can be improved by further pretraining on target language data. Our results show that continued pretraining is the most effective method to adapt a wav2vec 2.0 model for a new language and leads to considerable reduction in error rates. Furthermore, we find that if a model pretrained on a related speech variety or an unrelated language with similar phonological characteristics is available, multilingual fine-tuning using additional data from that language can have positive impact on speech recognition performance when there is very little labeled data in the target language.
更多查看译文
关键词
Automatic speech transcription,ASR,Wav2vec 2,0,Pretrained transformer models,Speech representation models,Cross-lingual transfer,Language documentation,Endangered languages,Underresourced languages,Sakhalin Ainu
AI 理解论文
溯源树
样例
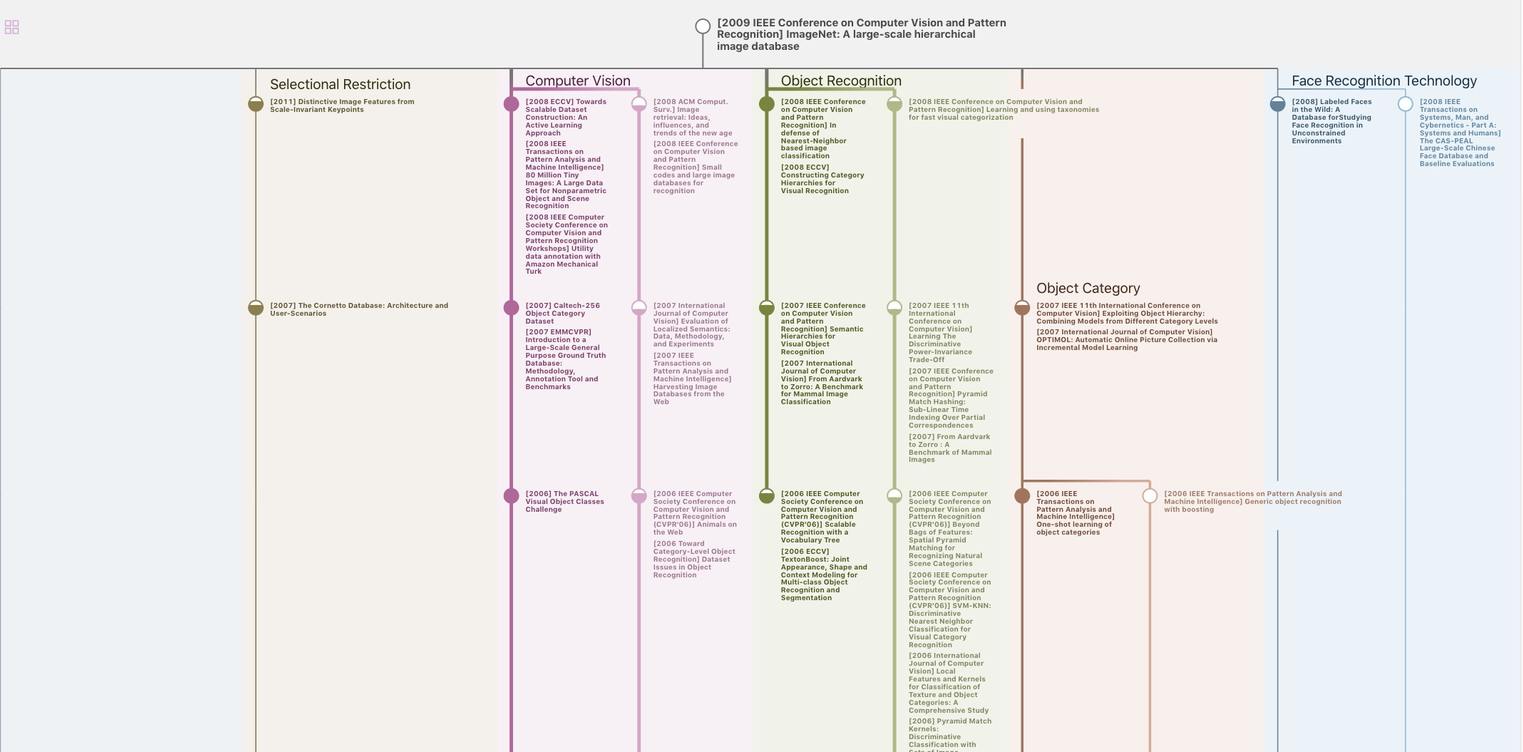
生成溯源树,研究论文发展脉络
Chat Paper
正在生成论文摘要