Sampled-data Control of Probabilistic Boolean Control Networks: A Deep Reinforcement Learning Approach
Information Sciences(2023)
摘要
The rise of reinforcement learning (RL) has guided a new paradigm: unraveling the inter-vention strategies to control systems with unknown dynamics. Model-free RL provides an exhaustive framework to devise therapeutic methods to alter the regulatory dynamics of gene regulatory networks (GRNs). This paper presents an RL-based technique to control GRNs modeled as probabilistic Boolean control networks (PBCNs). In particular, a double deep -Q network (DDQN) approach is proposed to address the sampled-data control (SDC) problem of PBCNs, and optimal state feedback controllers are obtained, rendering the PBCNs stabilized at a given equilibrium point. Our approach is based on options, i.e., the temporal abstractions of control actions in the Markov decision processes (MDPs) framework. First, we define options and hierarchical options and give their properties. Then, we introduce multi-time models to compute the optimal policies leveraging the options framework. Furthermore, we present a DDQN algorithm: i) to concurrently design the feedback controller and the sampling period; ii) wherein the controller intelligently decides the sampled period to update the control actions under the SDC scheme. The pre-sented method is model-free and offers scalability, thereby providing an efficient way to control large-scale PBCNs. Finally, we compare our control policy with state-of-the-art con-trol techniques and validate the presented results.(c) 2022 Elsevier Inc. All rights reserved.
更多查看译文
关键词
Sampled-data control (SDC),Probabilistic Boolean control networks,(PBCNs),Markov decision processes (MDPs),Deep reinforcement learning,Gene regulatory networks (GRNs)
AI 理解论文
溯源树
样例
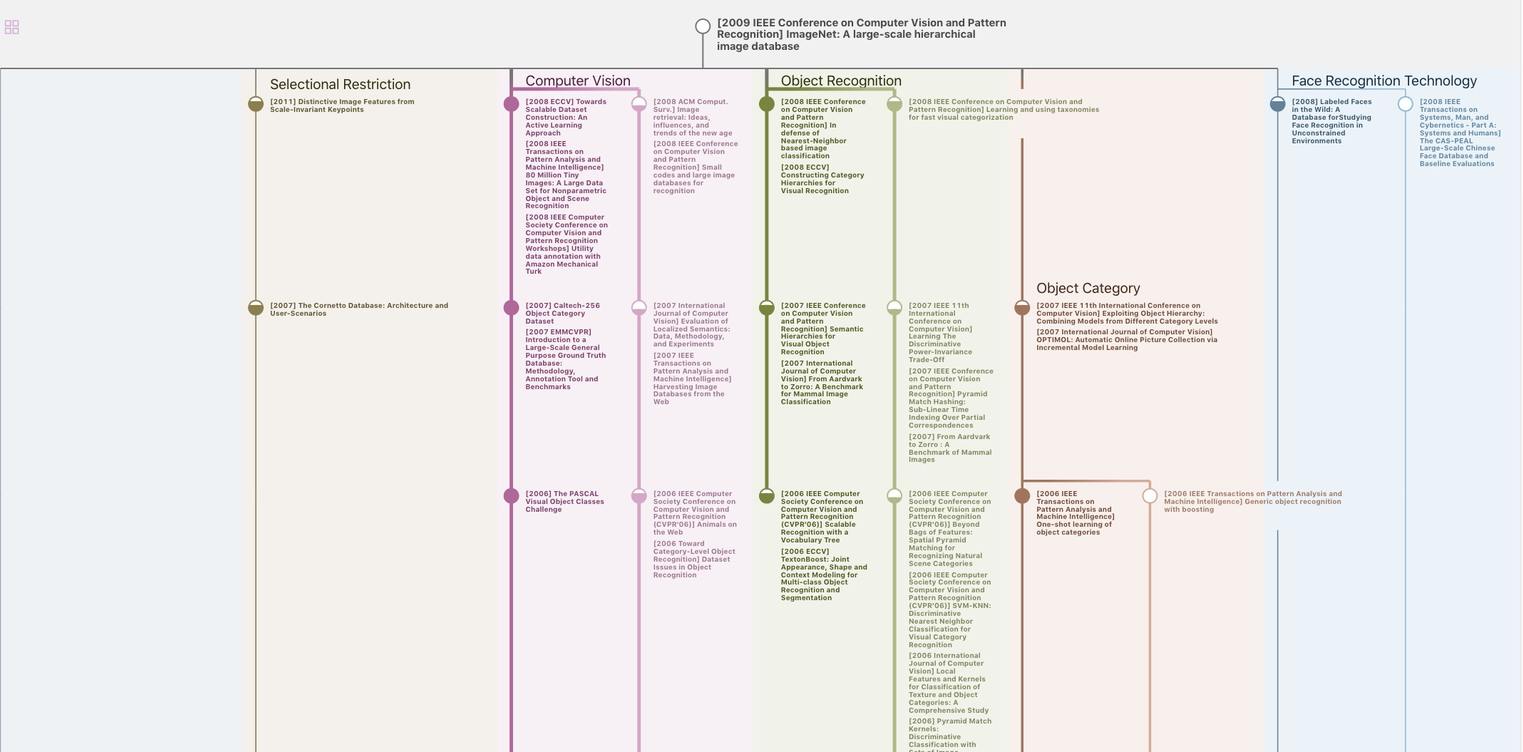
生成溯源树,研究论文发展脉络
Chat Paper
正在生成论文摘要